Tell Me More: Black Box Explainability for APT Detection on System Provenance Graphs
ICC 2023 - IEEE INTERNATIONAL CONFERENCE ON COMMUNICATIONS(2023)
摘要
Nowadays, companies, critical infrastructure and governments face cyber attacks every day ranging from simple denial-of-service and password guessing attacks to complex nation-state attack campaigns, so-called advanced persistent threats (APTs). Defenders employ intrusion detection systems (IDSs) among other tools to detect malicious activity and protect network assets. With the evolution of threats, detection techniques have followed with modern systems usually relying on some form of artificial intelligence (AI) or anomaly detection as part of their defense portfolio. While these systems are able to achieve higher accuracy in detecting APT activity, they cannot provide much context about the attack, as the underlying models are often too complex to interpret. This paper presents an approach to explain single predictions (i. e., detected attacks) of any graph-based anomaly detection systems. By systematically modifying the input graph of an anomaly and observing the output, we leverage a variation of permutation importance to identify parts of the graph that are likely responsible for the detected anomaly. Our approach treats the anomaly detection function as a black box and is thus applicable to any whole-graph explanation problems. Our results on two established datasets for APT detection (StreamSpot & DARPA TC Engagement Three) indicate that our approach can identify nodes that are likely part of the anomaly. We quantify this through our area under baseline (AuB) metric and show how the AuB is higher for anomalous graphs. Further analysis via the Wilcoxon rank-sum test confirms that these results are statistically significant with a p-value of 0.0041%.
更多查看译文
关键词
advanced persistent threat,explainable artificial intelligence,data provenance,APT,AI,XAI
AI 理解论文
溯源树
样例
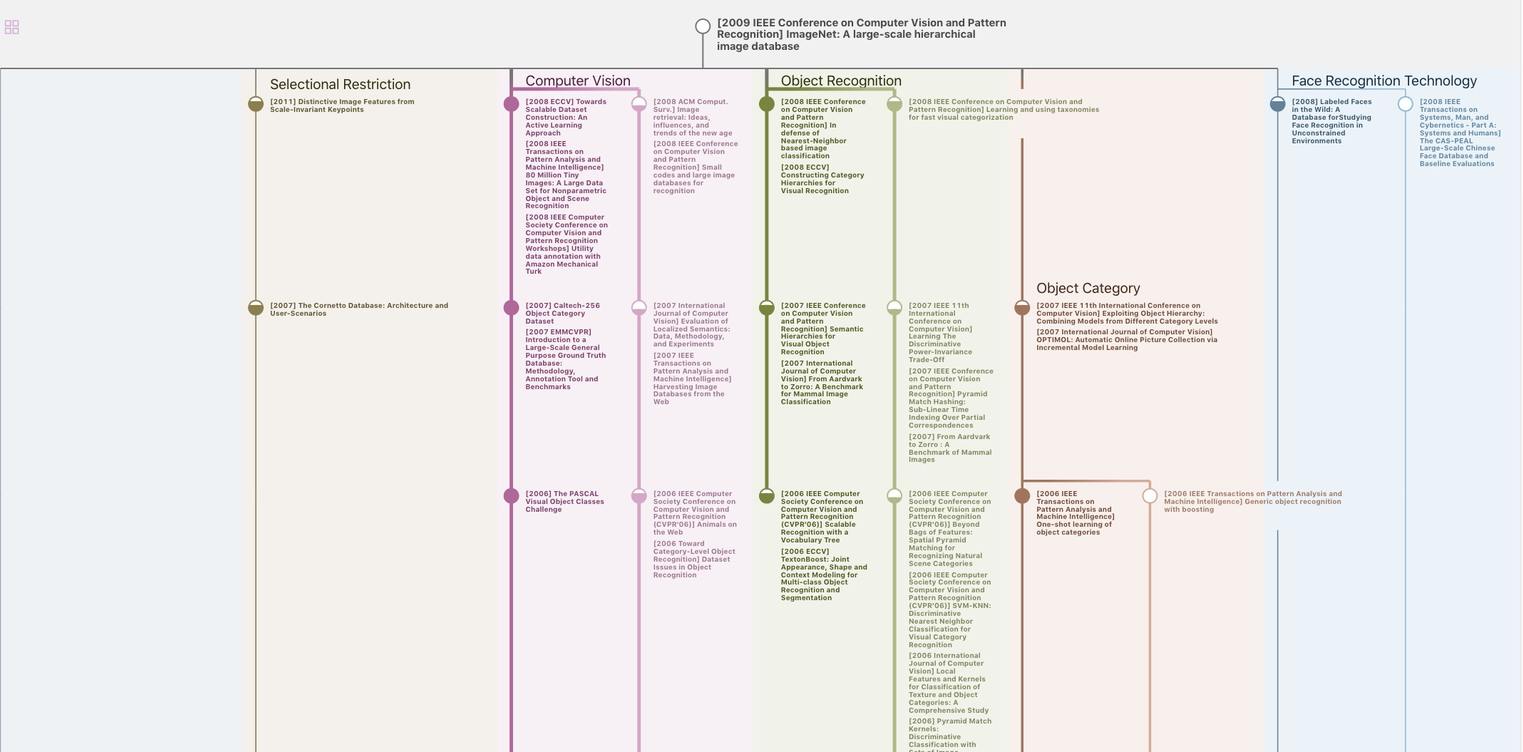
生成溯源树,研究论文发展脉络
Chat Paper
正在生成论文摘要