Joint Resource Allocation and String-Stable CACC Design with Multi-Agent Reinforcement Learning
ICC 2023 - IEEE INTERNATIONAL CONFERENCE ON COMMUNICATIONS(2023)
摘要
Resource allocation has always been a challenging task in vehicular networks due to their dynamic nature. In this paper, we study the decentralized joint subchannel allocation and power control problem for a Cooperative Adaptive Cruise Control (CACC) system to satisfy string stability in a platoon of connected and autonomous vehicles. The developed optimization problem takes the string stability of the platoon as well as the reliability of all the Vehicle to Vehicle (V2V) links into account, aiming at maximizing the total ergodic capacity. We tackle the optimization problem from two different angles. The first approach is a centralized classical algorithm governed from the Base Station (BS) perspective, where we assume that the BS only knows the large-scale fading information of the V2V links due to the rapidly changing channel conditions in vehicular environments. In the second strategy, we devise a Federated Multi-Agent Reinforcement Learning (MARL) based algorithm where each transmitter vehicle in the platoon acts as an independent agent and tries to find an optimal policy to maximize its total expected reward. Finally, to better understand the policies each agent has learned, we also compare the performance of these algorithms in terms of per-link achievable capacity.
更多查看译文
关键词
MARL,CACC,resource allocation,federated learning
AI 理解论文
溯源树
样例
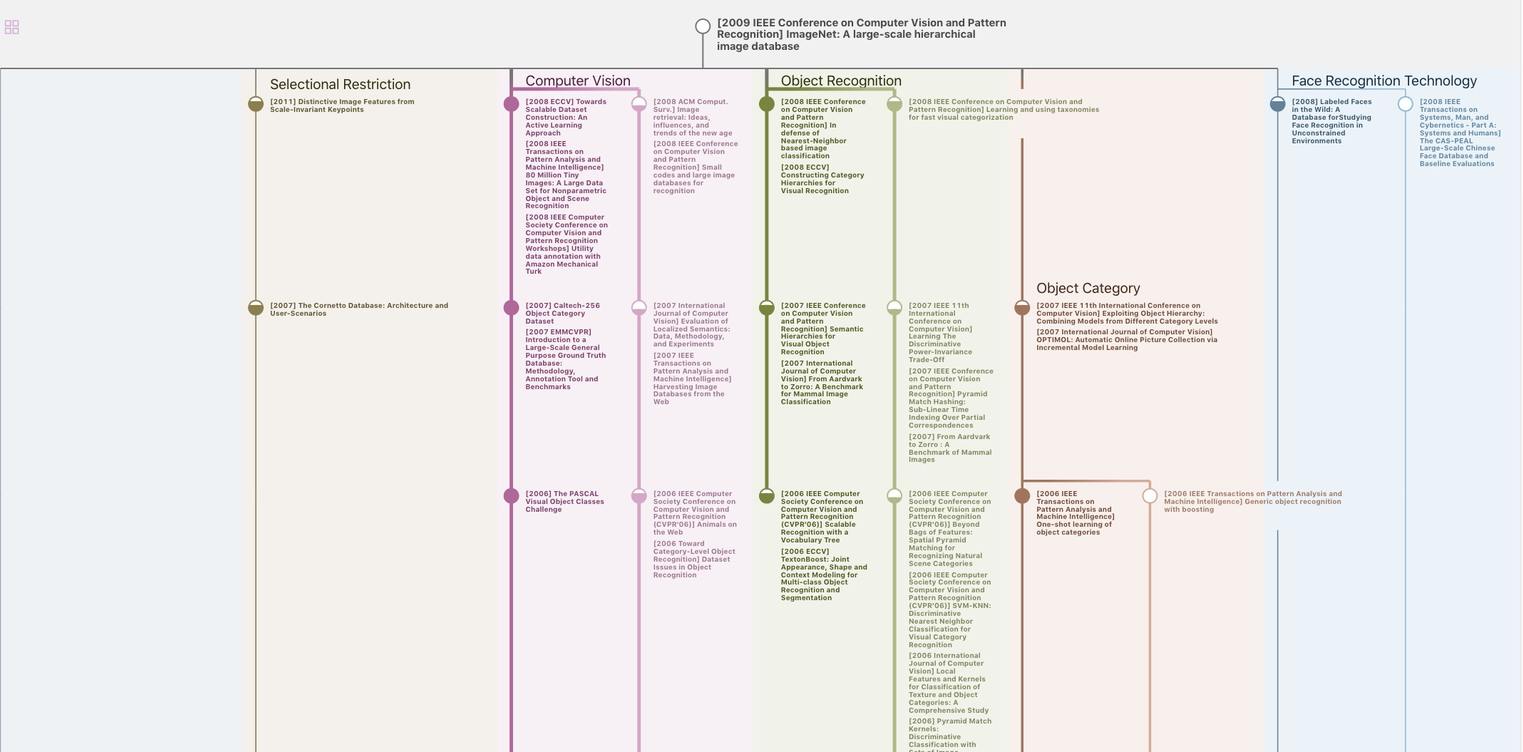
生成溯源树,研究论文发展脉络
Chat Paper
正在生成论文摘要