DDA-Net: A Discrepancy-Based Domain Adaptation Network for CSI Feedback Transferability
ICC 2023 - IEEE INTERNATIONAL CONFERENCE ON COMMUNICATIONS(2023)
摘要
The deep learning (DL)-based channel state information (CSI) feedback methods have been intensively explored in recent years. Most existing works are trained offline based on the prestored datasets. However, in real-world deployment, the pretrained model may not fit the field environment due to the wireless environment changes. In a previous study, a supervised learning approach has been introduced to deal with this CSI feedback transferability problem by finetuning the model. Nevertheless, the transmission for the original uncompressed CSI data will cause intense traffic in air interface. In this paper, a novel unsupervised transfer learning framework named Discrepancy-based Domain Adaptation Network (DDA-Net) is proposed to solve this problem. By minimizing the discrepancy between the CSI codeword datasets from the pretrained environment and field environment, the encoder and decoder are finetuned to extract the common features in both environments, so that the DL-based CSI feedback model can also work properly in a drifted environment without transmitting any original uncompressed CSI data. Simulation results show that the DDA approach can augment CSI feedback reconstruction accuracy and combat overfitting problems in the deployment environment. Compared to the existing supervised learning approach, the DDA approach can achieve similar CSI recovery performance without transmitting the original uncompressed CSI data, reducing considerable transmission traffic.
更多查看译文
关键词
CSI feedback,transfer learning,domain adaption,unsupervised learning
AI 理解论文
溯源树
样例
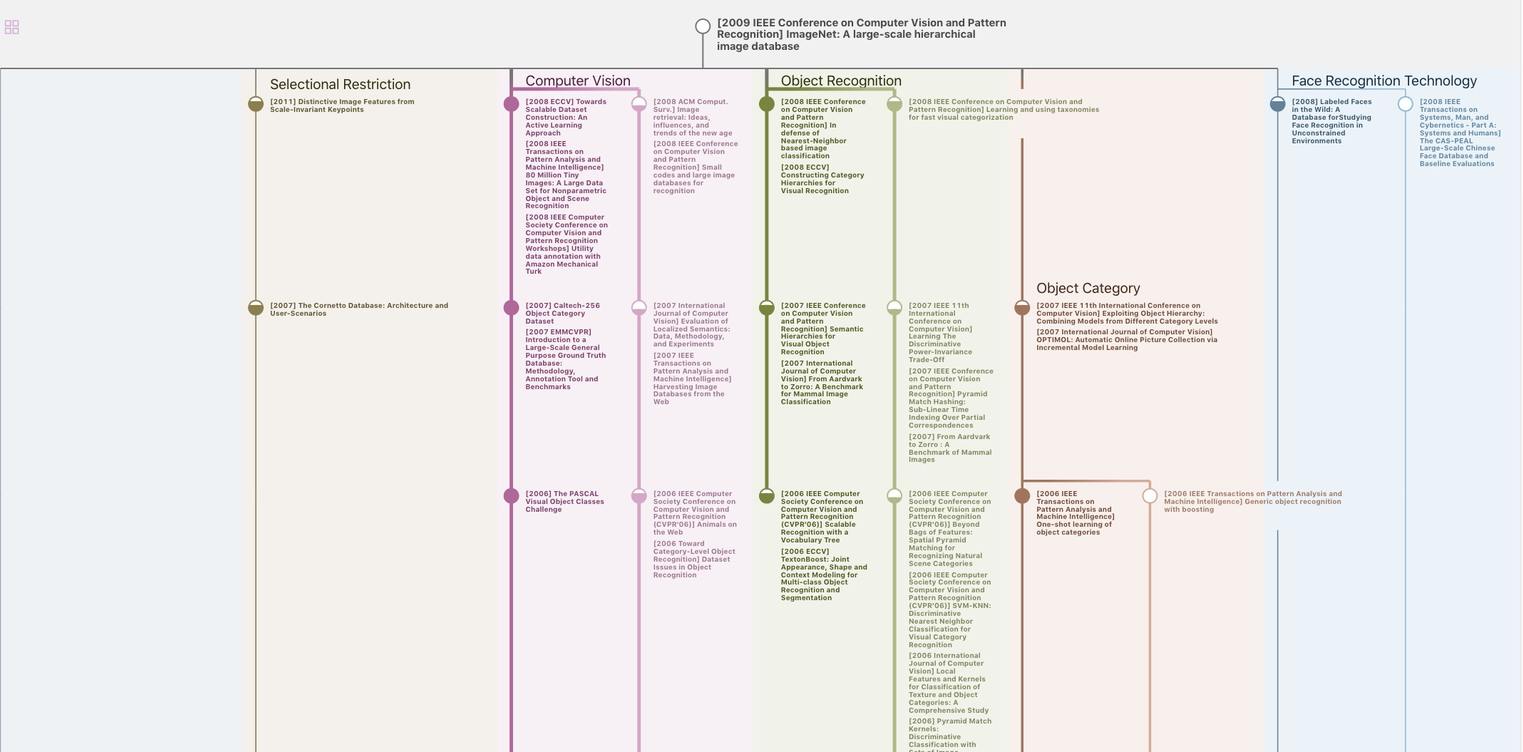
生成溯源树,研究论文发展脉络
Chat Paper
正在生成论文摘要