Radionuclide Identification in Open Environment Based on CsI(Na) Scintillation Detector Using Machine Learning Methods.
IC3INA(2023)
摘要
The country’s border areas are vulnerable areas to be affected by neighbouring countries, such as natural resource abuses, smuggling, and environmental pollution. One of the environmental pollution threats is coming from radionuclide sources, both natural and artificial, such as nuclear facility technologies. To safeguard against those threats, PRTDRAN BRIN has planned to provide the technology of radiation and environment monitoring stations at several national border areas. Currently, these stations can only measure gross radiation levels, lacking the capacity for radionuclide identification. This deficiency leads to potentially misleading readings in the presence of anomalies, often attributable to Naturally Occurring Radioactive Material (NORM) or Technologically Enhanced Naturally Occurring Radioactive Material (TNORM). Hence, a device with radionuclide identification capability was built using machine learning methods based on CsI(Na) Scintillation Detector. Datasets collection was conducted in the laboratory with varying counting times from 1 upto 120 minutes and the source-detector distance from 50 up to 150 cm. The Histogram of Oriented Gradients (HOG) method was selected as the feature extraction method with several methods of division regions according to radionuclide peaks. The classification methods determined to be compared are Linear Regression (LR), Linear Discrimination Analysis (LDA), K-Nearest Neighbor (KNN), Decision Tree, Naïve Bayes, Support Vector Machine (SVM), and Multi-Layer Perceptron (MLP) of Neural Network. The test result showed that combining the classification method using SVM and segmenting the spectrum into 3 regions as a feature extraction method is the best, with an accuracy score of 97.845% and training time of 0.997 ms.
更多查看译文
AI 理解论文
溯源树
样例
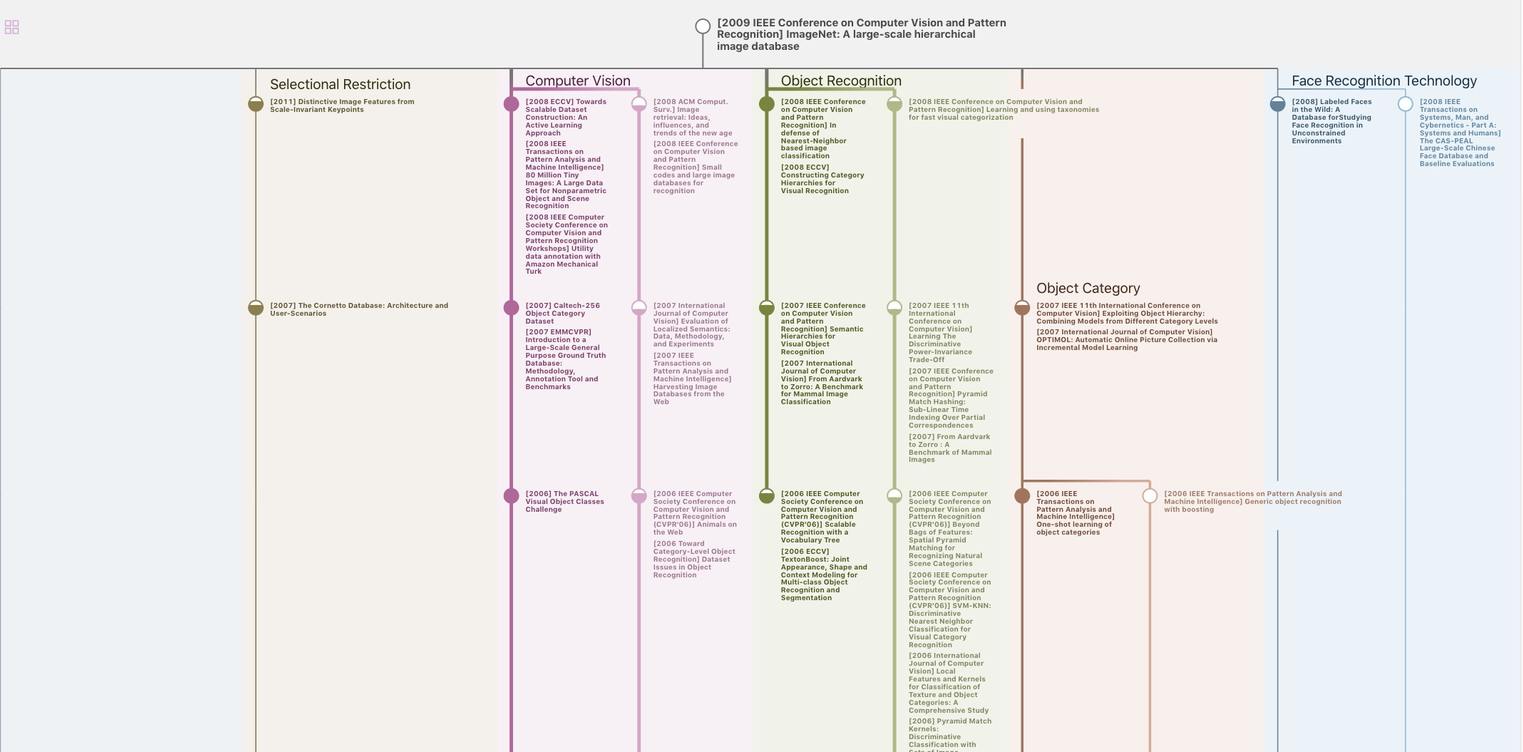
生成溯源树,研究论文发展脉络
Chat Paper
正在生成论文摘要