Cellular Network Antenna Tilt Anomaly Detection Using Federated Unsupervised Learning
ICC 2023 - IEEE INTERNATIONAL CONFERENCE ON COMMUNICATIONS(2023)
摘要
An important issue for cellular network operators is how to maintain radio coverage so that any issues can be addressed before they impact user services. This is particularly important in dense small cell network deployment scenarios such as vehicular networks. Antenna electrical tilt is a key factor in this, as unintended deviations from the planned value can adversely affect coverage and service reliability. We propose a novel method to detect antenna tilt anomalies using existing data sources without the need for additional hardware to be deployed in the radio access network. Our approach goes beyond previous techniques by using federated unsupervised learning based on polar coordinates, together with a geometrical transformation to normalise data across multiple sites. By using this approach to combine scarce training data from multiple cells, we can achieve detection accuracy in excess of 95% in a way that minimises training data size as well as computing power and memory usage.
更多查看译文
关键词
federated learning,unsupervised learning,antenna tilt,fault detection
AI 理解论文
溯源树
样例
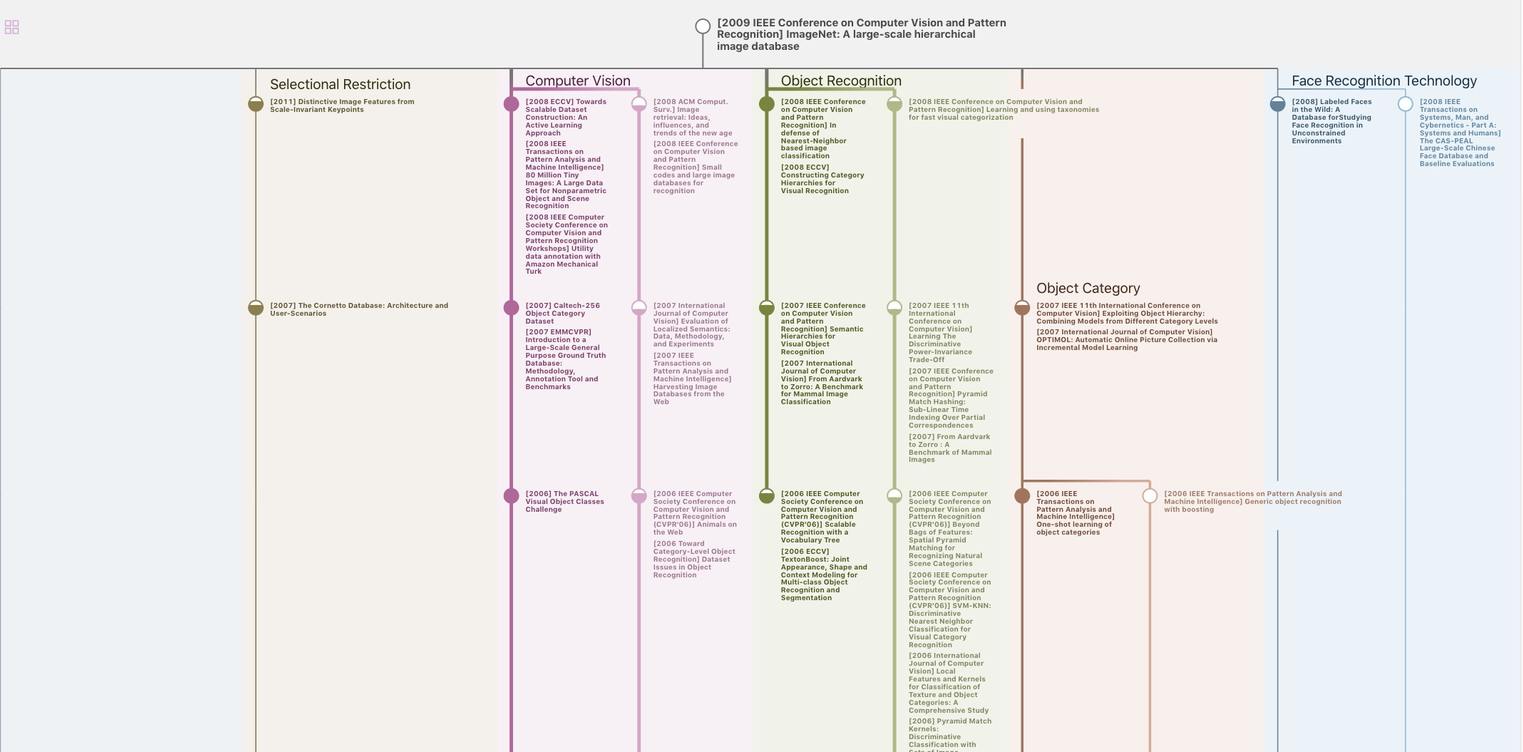
生成溯源树,研究论文发展脉络
Chat Paper
正在生成论文摘要