Data-Efficient Adaptive Global Pruning for Convolutional Neural Networks in Edge Computing
ICC 2023 - IEEE INTERNATIONAL CONFERENCE ON COMMUNICATIONS(2023)
摘要
Deep convolutional neural networks are hindered from empowering resource-constrained devices due to their demanding computational and storage resources. Structured pruning effectively removes the redundant components from neural networks and obtains compact models. Previous pruning methods usually evaluate the importance of filters from a layer-wise perspective, which is deprived of global guidance. We propose an adaptive pruning algorithm based on relevance scores to evaluate the contribution of each channel by calculating its relevance score from the back-propagation of the neural network's output. Our method identifies and removes channels with low contribution from a global perspective. Unlike previous methods that manually set the pruning rate for each pruning iteration, our method adaptively adjusts the pruning rate. In addition, our method performs satisfactorily with limited data for one-shot pruning in the absence of fine-tuning. The ability to obtain compact models through one-shot pruning with limited data is ideally suited for edge computing scenarios. We validate the effectiveness of our method with multiple combinations of convolutional neural networks and datasets. Our approach outperforms existing pruning methods in scenarios with limited data.
更多查看译文
关键词
Edge computing,convolutional neural networks,structured pruning,data-efficient,model compression
AI 理解论文
溯源树
样例
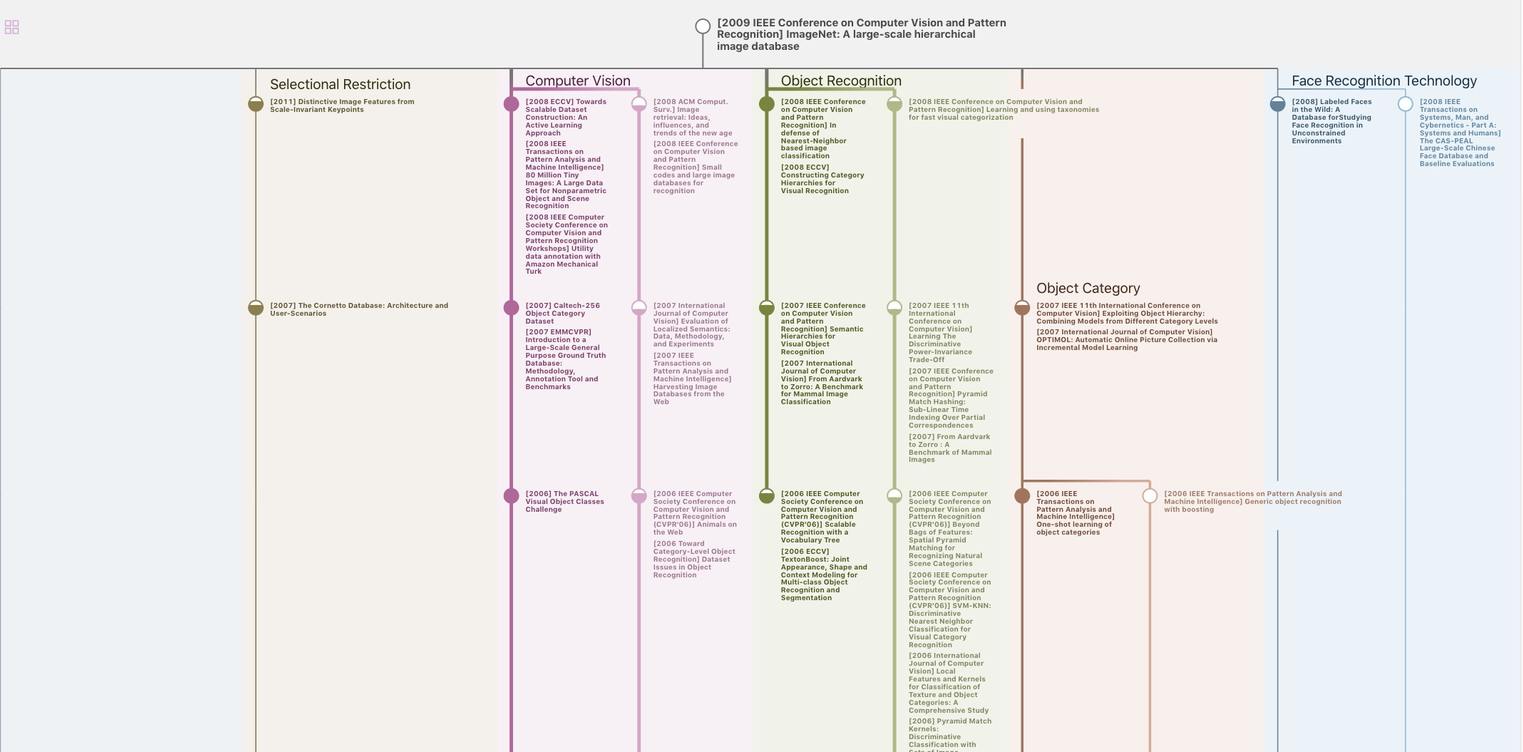
生成溯源树,研究论文发展脉络
Chat Paper
正在生成论文摘要