Safety Monitoring for Pedestrian Detection in Adverse Conditions.
RV(2023)
摘要
Pedestrian detection is an important part of the perception system of autonomous vehicles. Foggy and low-light conditions are quite challenging for pedestrian detection, and several models have been proposed to increase the robustness of detections under such challenging conditions. Checking if such a model performs well is largely evaluated by manually inspecting the results of object detection. We propose a monitoring technique that uses Timed Quality Temporal Logic (TQTL) to do differential testing: we first check when an object detector (such as vanilla YOLO) fails to accurately detect pedestrians using a suitable TQTL formula on a sequence of images. We then apply a model specialized to adverse weather conditions to perform object detection on the same image sequence. We use Image-Adaptive YOLO (IA-YOLO) for this purpose. We then check if the new model satisfies the previously failing specifications. Our method shows the feasibility of using such a differential testing approach to measure the improvement in quality of detections when specialized models are used for object detection.
更多查看译文
关键词
pedestrian detection,safety monitoring
AI 理解论文
溯源树
样例
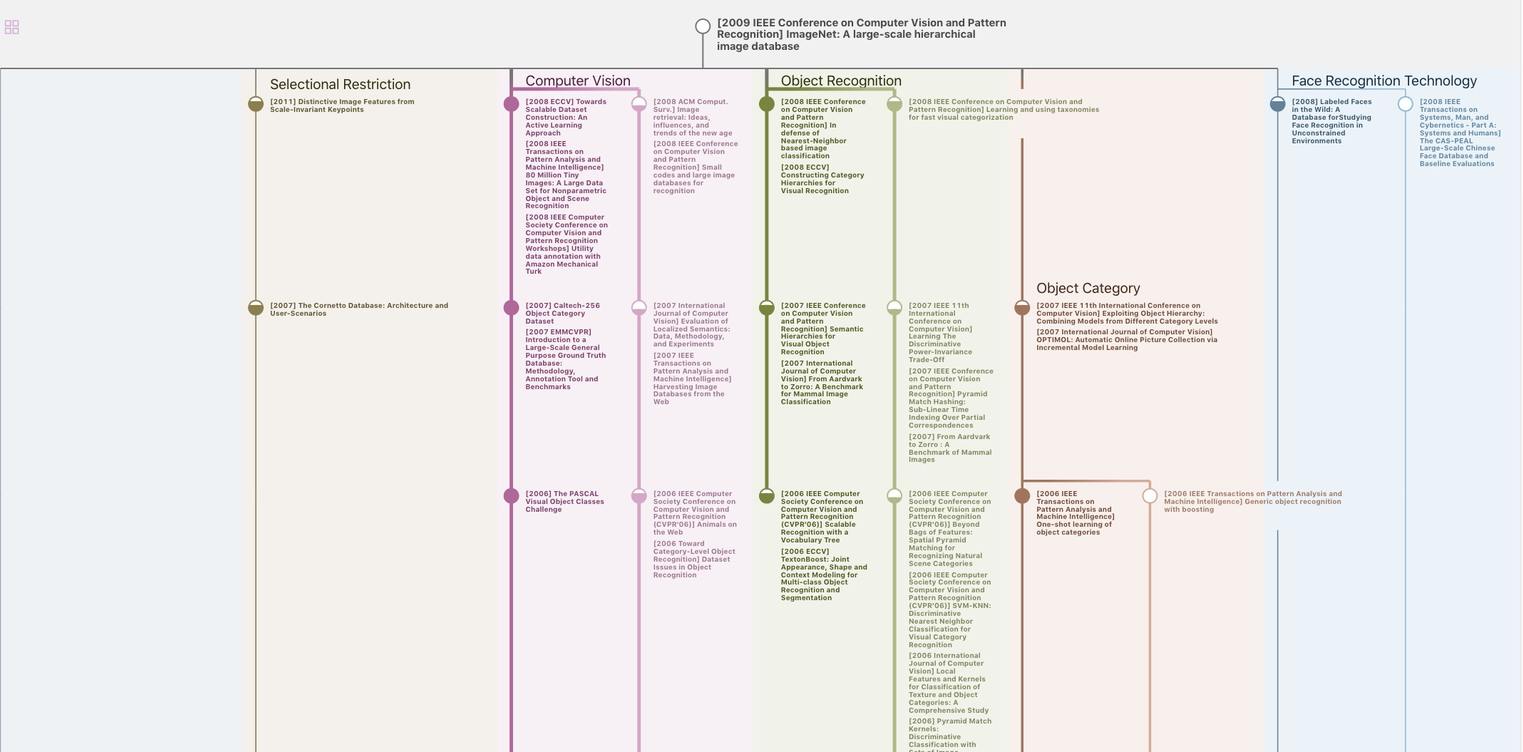
生成溯源树,研究论文发展脉络
Chat Paper
正在生成论文摘要