Low-Complexity Channel Estimation and Localization with Random Beamspace Observations.
ICC(2023)
摘要
We investigate the problem of low-complexity, high-dimensional channel estimation with beamspace observations, for the purpose of localization. Existing work on beamspace ESPRIT (estimation of signal parameters via rotational invariance technique) approaches requires either a shift-invariance structure of the transformation matrix, or a full-column rank condition. We extend these beamspace ESPRIT methods to a case when neither of these conditions is satisfied, by exploiting the full-row rank of the transformation matrix. We first develop a tensor decomposition-based approach, and further design a matrix-based ESPRIT method to achieve auto-pairing of the channel parameters, with reduced complexity. Numerical simulations show that the proposed methods work in the challenging scenario, and the matrix-based ESPRIT approach achieves better performance than the tensor ESPRIT method.
更多查看译文
AI 理解论文
溯源树
样例
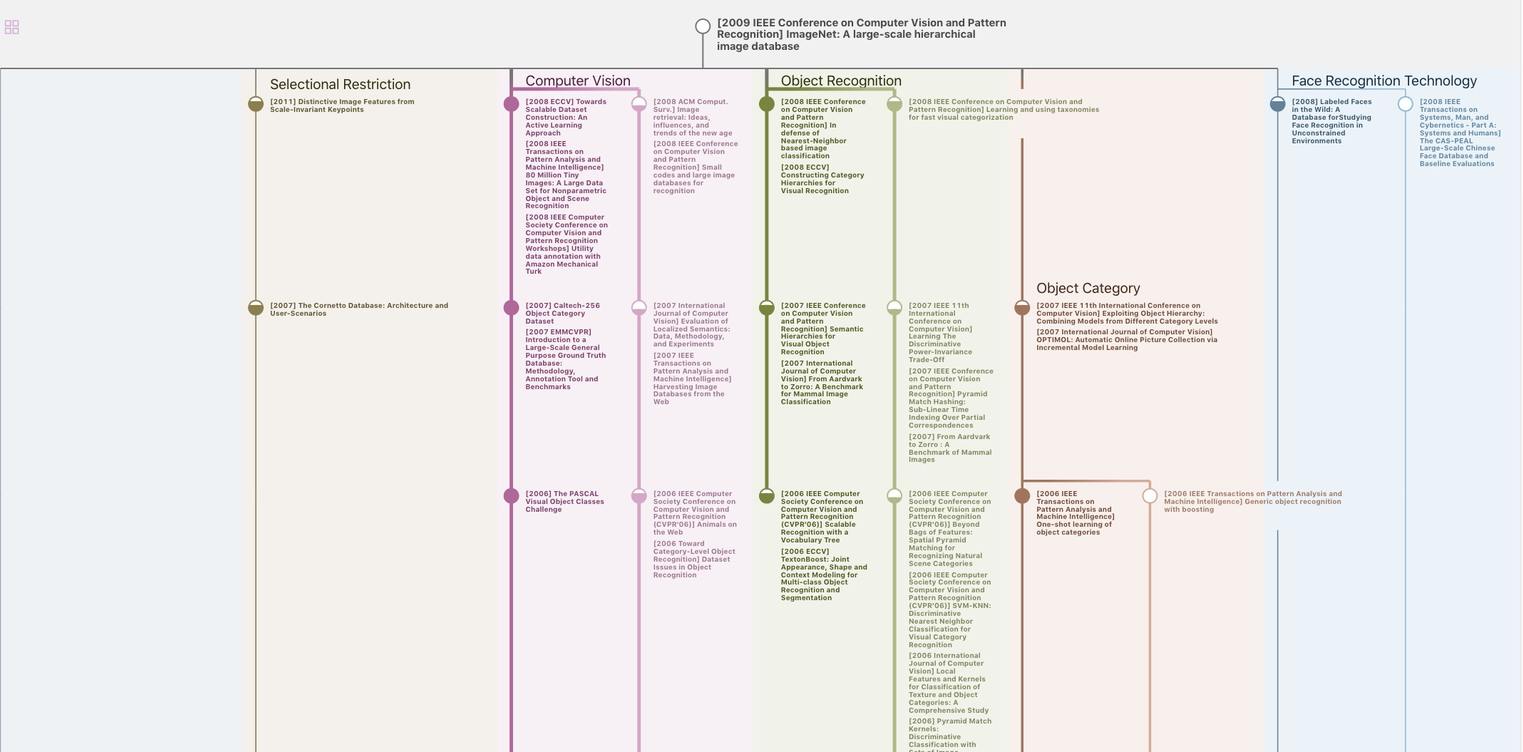
生成溯源树,研究论文发展脉络
Chat Paper
正在生成论文摘要