Supervised Contrastive Learning For Musical Onset Detection
PROCEEDINGS OF THE 18TH INTERNATIONAL AUDIO MOSTLY CONFERENCE, AM 2023(2023)
摘要
This paper applies supervised contrastive learning to musical onset detection to alleviate the issue of noisy annotated data for onset datasets. The results are compared against a state-of-the-art, convolutional, cross-entropy model. Both models were trained on two datasets. The first dataset comprised of a manually annotated selection of music. This data was then augmented with inaccurate labelling to produce the second data set. When trained on the original data the supervised contrastive model produced an F1 score of 0.878. This was close to the cross-entropy model score of 0.888. This showed that supervised contrastive loss is applicable to onset detection but does not outperform cross-entropy models in an ideal training case. When trained on the augmented set the contrastive model consistently outperformed the cross-entropy model across increasing percentage inaccuracies, with a difference in F1 score of 0.1 for the most inaccurate data. This demonstrates the robustness of supervised contrastive learning with inaccurate data for onset detection, suggesting that supervised contrastive loss could provide a new onset detection architecture which is invariant to noise in the data or inaccuracies in labelling.
更多查看译文
关键词
contrastive learning,onset detection,data inaccuracies
AI 理解论文
溯源树
样例
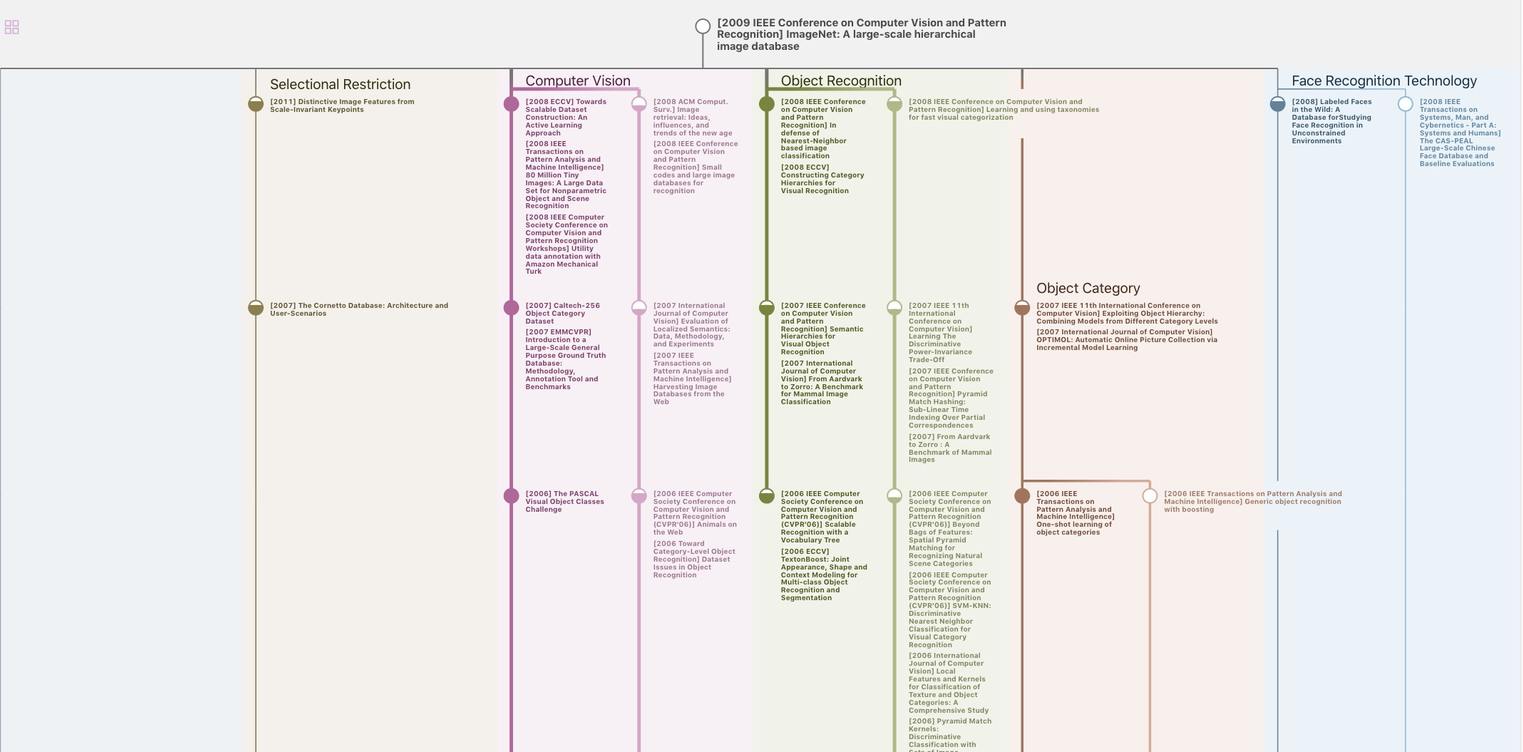
生成溯源树,研究论文发展脉络
Chat Paper
正在生成论文摘要