CycleSTTN: A Learning-Based Temporal Model for Specular Augmentation in Endoscopy
MEDICAL IMAGE COMPUTING AND COMPUTER ASSISTED INTERVENTION, MICCAI 2023, PT X(2023)
摘要
Feature detection and matching is a computer vision problem that underpins different computer assisted techniques in endoscopy, including anatomy and lesion recognition, camera motion estimation, and 3D reconstruction. This problem is made extremely challenging due to the abundant presence of specular reflections. Most of the solutions proposed in the literature are based on filtering or masking out these regions as an additional processing step. There has been little investigation into explicitly learning robustness to such artefacts with single-step end-to-end training. In this paper, we propose an augmentation technique (CycleSTTN) that adds temporally consistent and realistic specularities to endoscopic videos. Such videos can act as ground truth data with known texture occluded behind the added specularities. We demonstrate that our image generation technique produces better results than a standard CycleGAN model. Additionally, we leverage this data augmentation to re-train a deep-learning based feature extractor (SuperPoint) and show that it improves. CycleSTTN code is made available here.
更多查看译文
关键词
Surgical Data Science,Surgical AI,Generative AI,Deep Learning,Endoscopy,Specularity
AI 理解论文
溯源树
样例
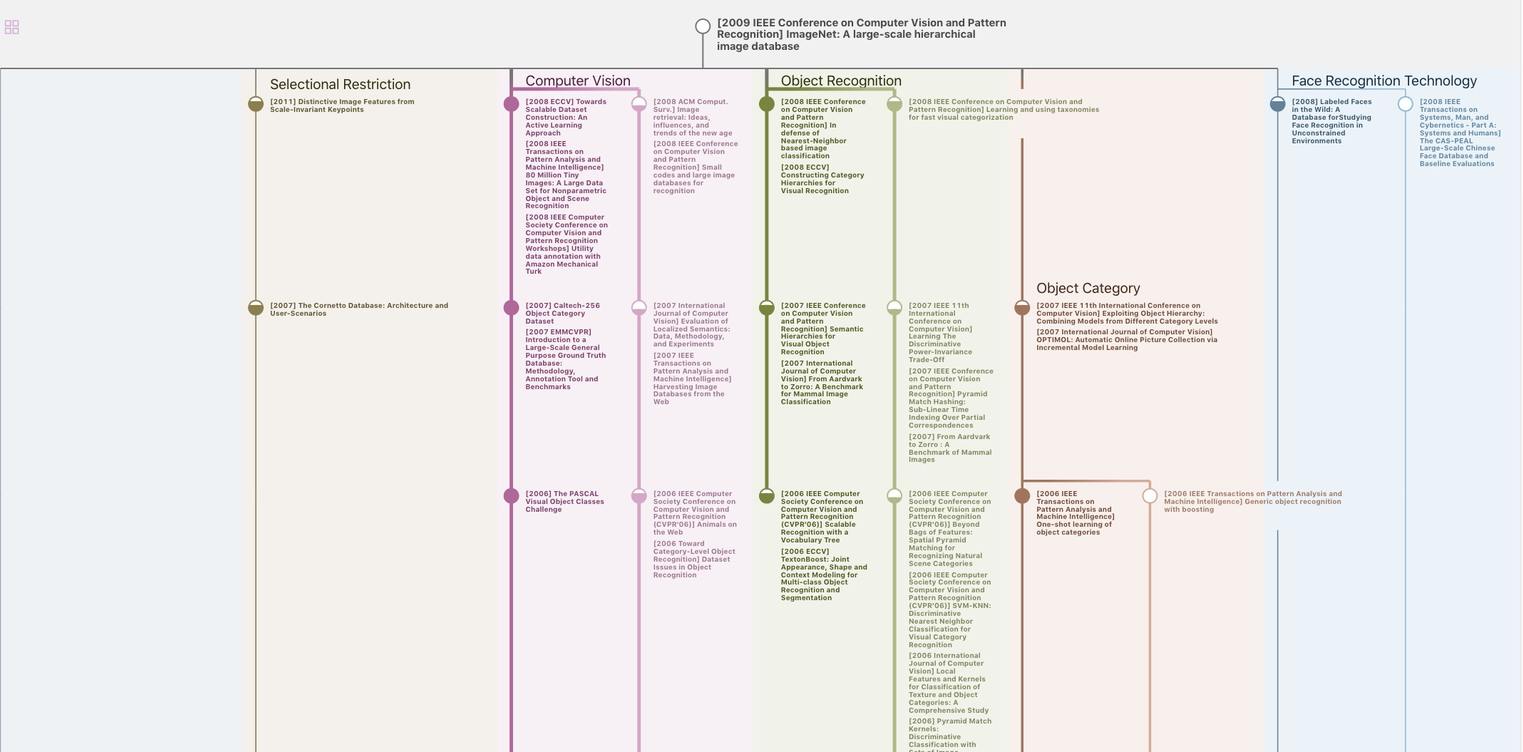
生成溯源树,研究论文发展脉络
Chat Paper
正在生成论文摘要