Probabilistic Modeling Ensemble Vision Transformer Improves Complex Polyp Segmentation
MEDICAL IMAGE COMPUTING AND COMPUTER ASSISTED INTERVENTION, MICCAI 2023, PT VII(2023)
摘要
Colorectal polyps detected during colonoscopy are strongly associated with colorectal cancer, making polyp segmentation a critical clinical decision-making tool for diagnosis and treatment planning. However, accurate polyp segmentation remains a challenging task, particularly in cases involving diminutive polyps and other intestinal substances that produce a high false-positive rate. Previous polyp segmentation networks based on supervised binary masks may have lacked global semantic perception of polyps, resulting in a loss of capture and discrimination capability for polyps in complex scenarios. To address this issue, we propose a novel Gaussian-Probabilistic guided semantic fusion method that progressively fuses the probability information of polyp positions with the decoder supervised by binary masks. Our Probabilistic Modeling Ensemble Vision Transformer Network(PETNet) effectively suppresses noise in features and significantly improves expressive capabilities at both pixel and instance levels, using just simple types of convolutional decoders. Extensive experiments on five widely adopted datasets show that PETNet outperforms existing methods in identifying polyp camouflage, appearance changes, and small polyp scenes, and achieves a speed about 27FPS in edge computing devices. Codes are available at: https://github.com/Seasonsling/PETNet.
更多查看译文
关键词
Colonoscopy,Polyp Segmentation,Vision Transformer
AI 理解论文
溯源树
样例
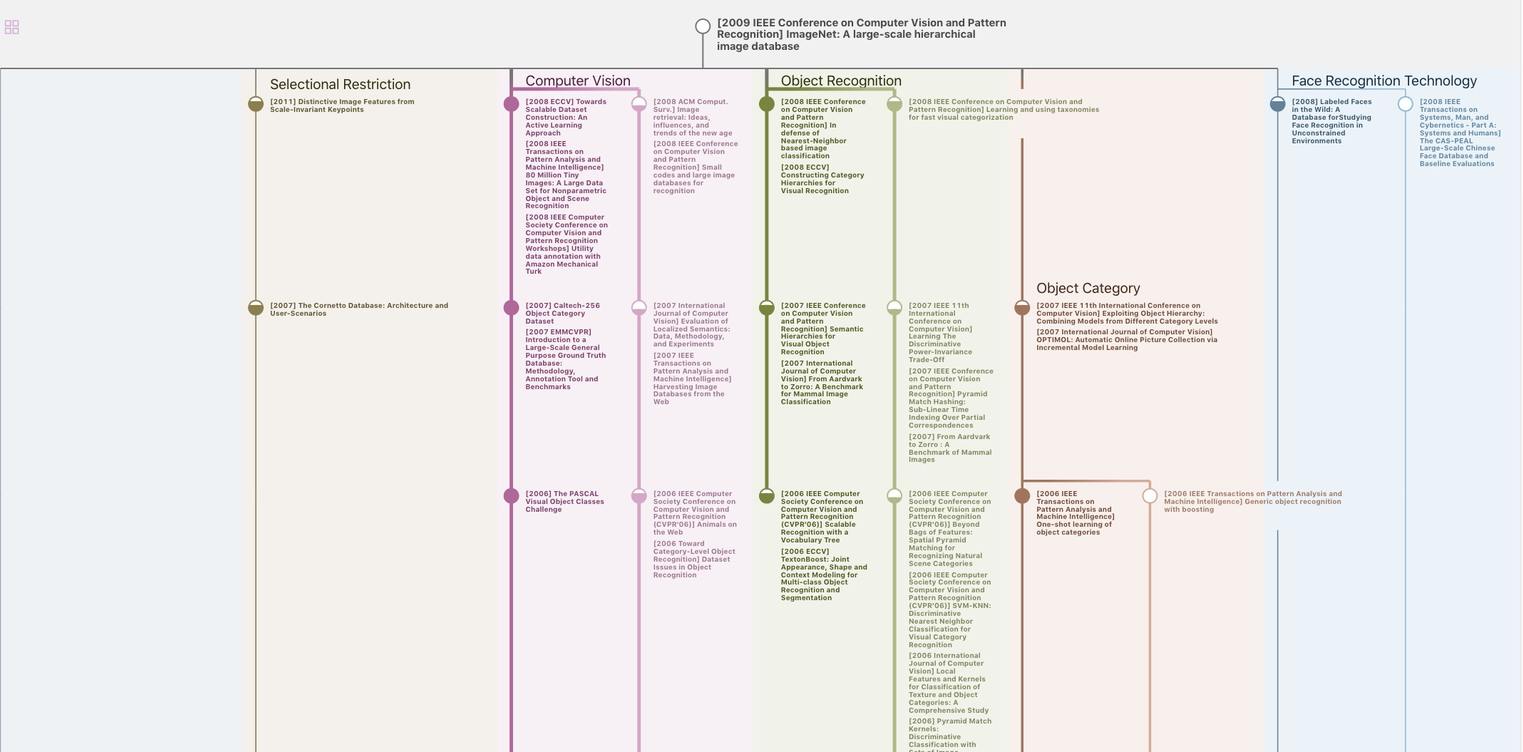
生成溯源树,研究论文发展脉络
Chat Paper
正在生成论文摘要