Multi-input Vision Transformer with Similarity Matching
PREDICTIVE INTELLIGENCE IN MEDICINE, PRIME 2023(2023)
Abstract
Multi-input models for image classification have recently gained considerable attention. However, multi-input models do not always exhibit superior performance compared to single models. In this paper, we propose a multi-input vision transformer (ViT) with similarity matching, which uses original and cropped images based on the region of interest (ROI) as inputs, without additional encoder architectures. Specifically, two types of images are matched on the basis of their cosine similarity in descending order, and they serve as inputs for a multi-input model with two parallel ViT-architectures. We conduct two experiments using a dataset of pediatric orbital wall fracture and chest X-rays. Consequently, the multi-input models with similarity matching outperform the baseline models and achieve balanced results. Furthermore, it is feasible that our method provides both global and local features, and the Grad-CAM results demonstrate that two different inputs of the proposed mechanism can help complementarily study the image. The code is available at https://github.com/duneag2/vit- similarity.
MoreTranslated text
Key words
Multi-input Learning,X-Ray Classification,Similarity
AI Read Science
Must-Reading Tree
Example
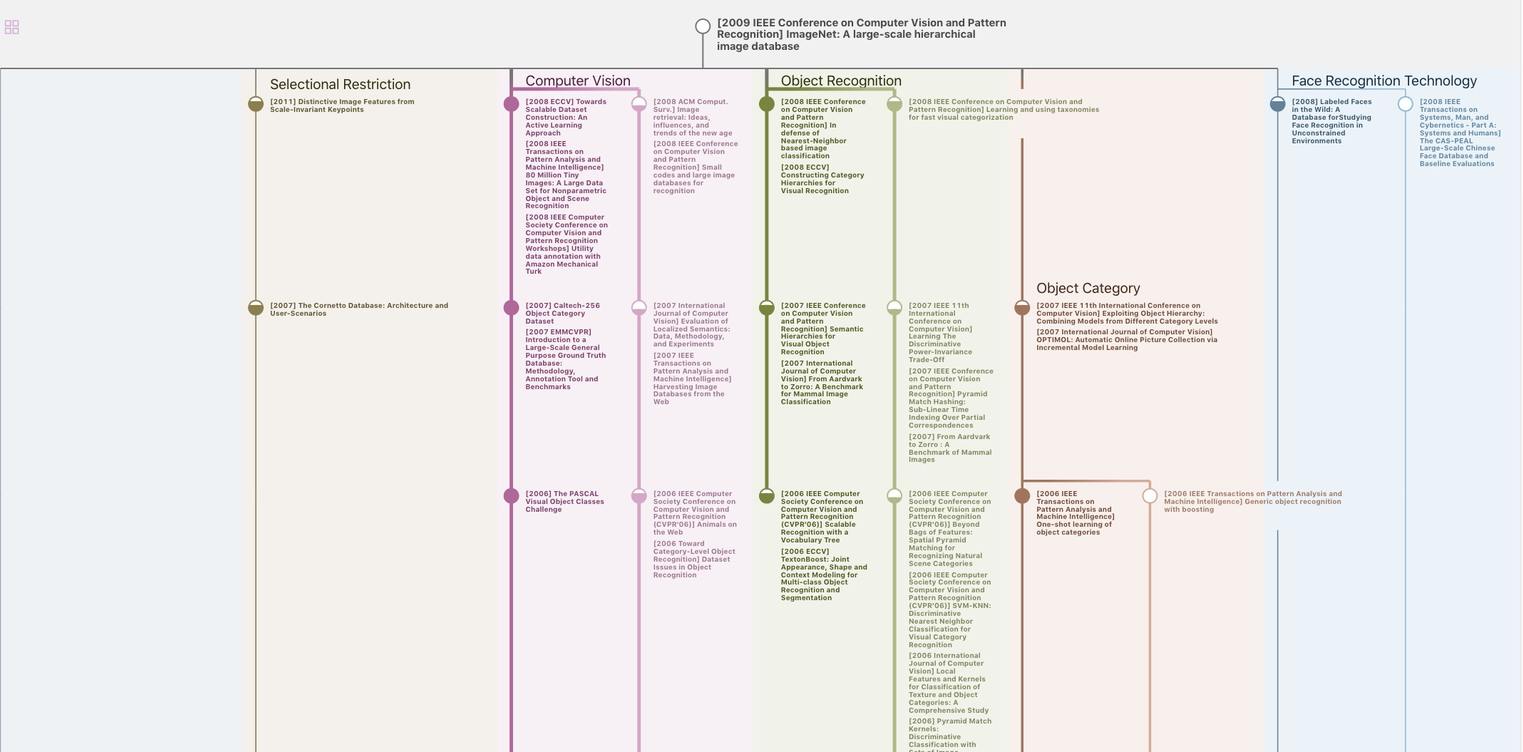
Generate MRT to find the research sequence of this paper
Chat Paper
Summary is being generated by the instructions you defined