Hierarchical Compositionality in Hyperbolic Space for Robust Medical Image Segmentation
DOMAIN ADAPTATION AND REPRESENTATION TRANSFER, DART 2023(2024)
摘要
Deep learning based medical image segmentation models need to be robust to domain shifts and image distortion for the safe translation of these models into clinical practice. The most popular methods for improving robustness are centred around data augmentation and adversarial training. Many image segmentation tasks exhibit regular structures with only limited variability. We aim to exploit this notion by learning a set of base components in the latent space whose composition can account for the entire structural variability of a specific segmentation task. We enforce a hierarchical prior in the composition of the base components and consider the natural geometry in which to build our hierarchy. Specifically, we embed the base components on a hyperbolic manifold which we claim leads to a more natural composition. We demonstrate that our method improves model robustness under various perturbations and in the task of single domain generalisation.
更多查看译文
关键词
hierarchical compositionality,hyperbolic space
AI 理解论文
溯源树
样例
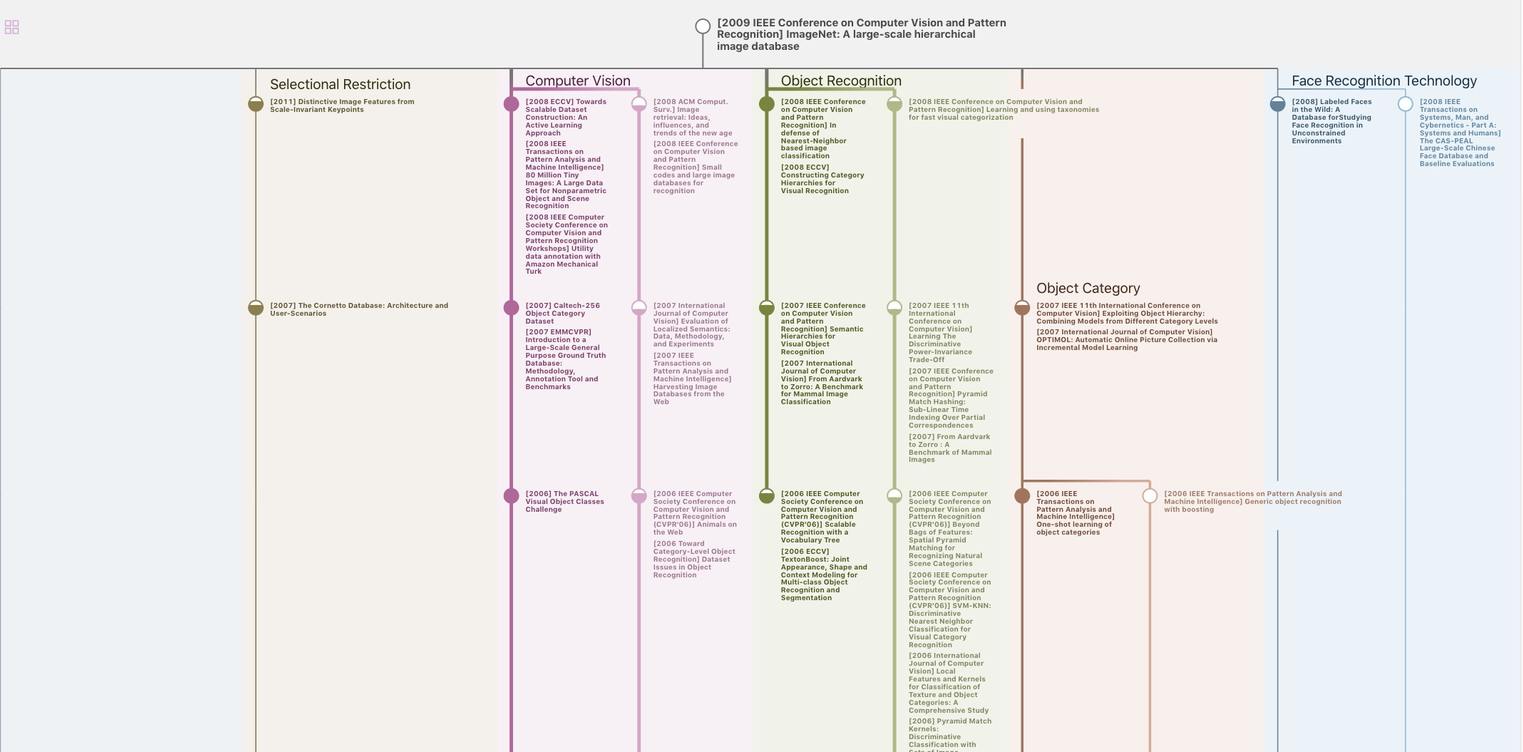
生成溯源树,研究论文发展脉络
Chat Paper
正在生成论文摘要