EFTuner: A Bi-Objective Configuration Parameter Auto-Tuning Method Towards Energy-Efficient Big Data Processing.
Internetware(2023)
摘要
Energy-efficiency now severely restricts the sustainable operation and development of big data services. In this paper, we propose a bi-objective configuration parameters auto-tuning method EFTuner towards energy-efficient big data processing. Following the sampling-modeling-searching workflow, EFTuner first leverages the Latin Hypercube Sampling to collect configuration sample data under multiple dataset input sizes and then separately build a datasize-aware prediction model for performance and energy consumption with Stochastic Gradient Boosted Regression Tree. Besides, to avoid meaningless variation in the evolutionary process of original NSGA-II, EFTuner also explores the Pareto-optimal configurations with a novel parameter importance-based mutation operation. Experiments conducted on a local 3-node Spark cluster with three different applications verify the advantages of EFTuner over the baselines.
更多查看译文
AI 理解论文
溯源树
样例
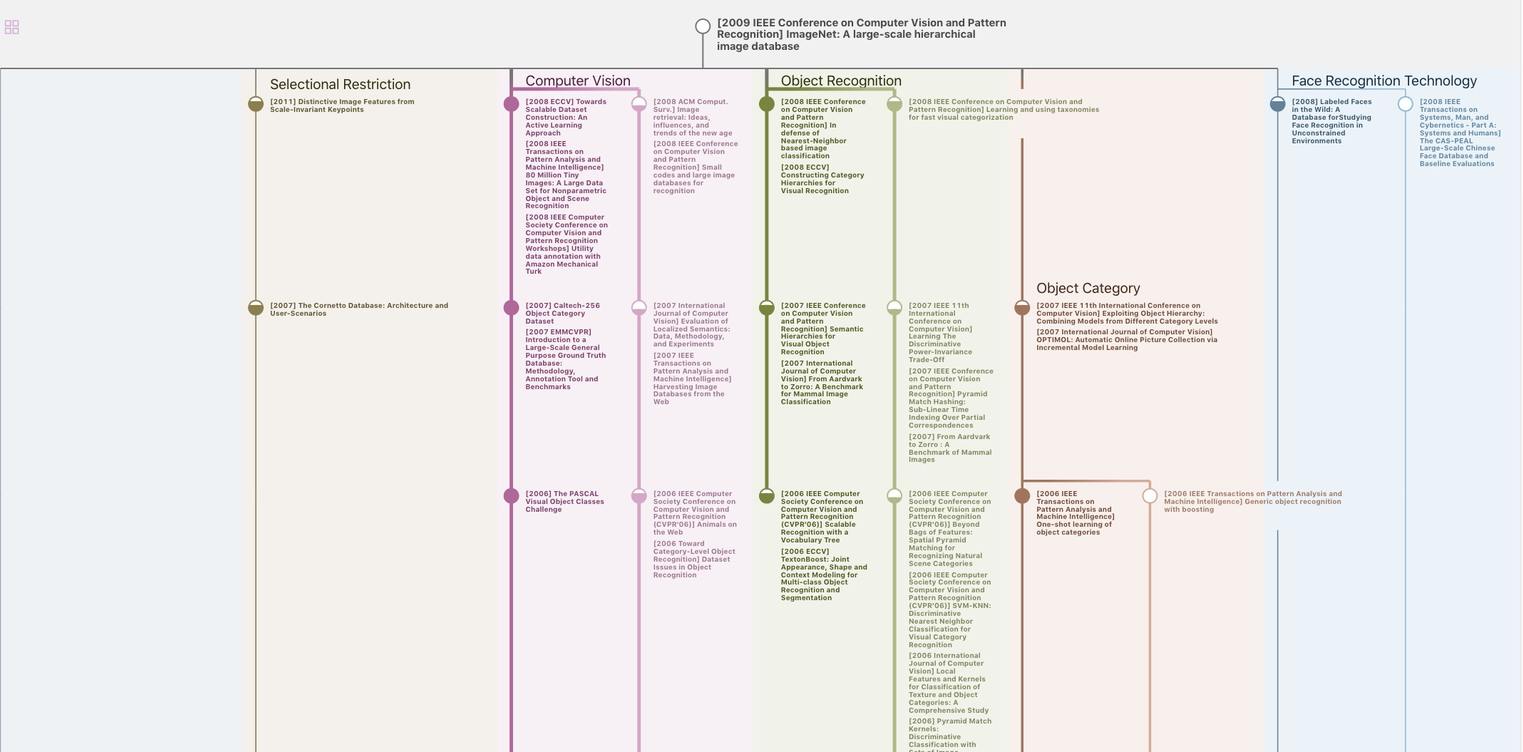
生成溯源树,研究论文发展脉络
Chat Paper
正在生成论文摘要