Invited Paper: Detection of False Data Injection Attacks in Power Systems Using a Secured-Sensors and Graph-Based Method.
SSS(2023)
摘要
False data injection (FDI) attacks pose a significant threat to the reliability of power system state estimation (PSSE). Recently, graph signal processing (GSP)-based detectors have been shown to enable the detection of well-designed cyber attacks named unobservable FDI attacks. However, current detectors, including GSP-based detectors, do not consider the impact of secured sensors on the detection process; thus, they may have limited power, especially in the low signal-to-noise ratio (SNR) regime. In this paper, we propose a novel FDI attack detection method that incorporates both knowledge of the locations of secured sensors and the GSP properties of power system states (voltages). We develop the secured-sensors-and-graph-Laplacian-based generalized likelihood ratio test (SSGL-GLRT) that integrates the secured data and the graph smoothness properties of the state variables. Furthermore, we introduce a generalization of the method that allows the use of different high-pass GSP filters together with prior knowledge of the locations of the secured sensors. Then, we develop the SSGL-GLRT for a distributed PSSE based on the alternating direction method of multipliers (ADMM). Numerical simulations demonstrate that the proposed method significantly improves the probability of detecting FDI attacks compared to existing GSP-based detectors, achieving an increase of up to 30% in the detection probability for the same false alarm rate by integrating secured sensor location information.
更多查看译文
关键词
false data injection attacks,power systems,secured-sensors,graph-based
AI 理解论文
溯源树
样例
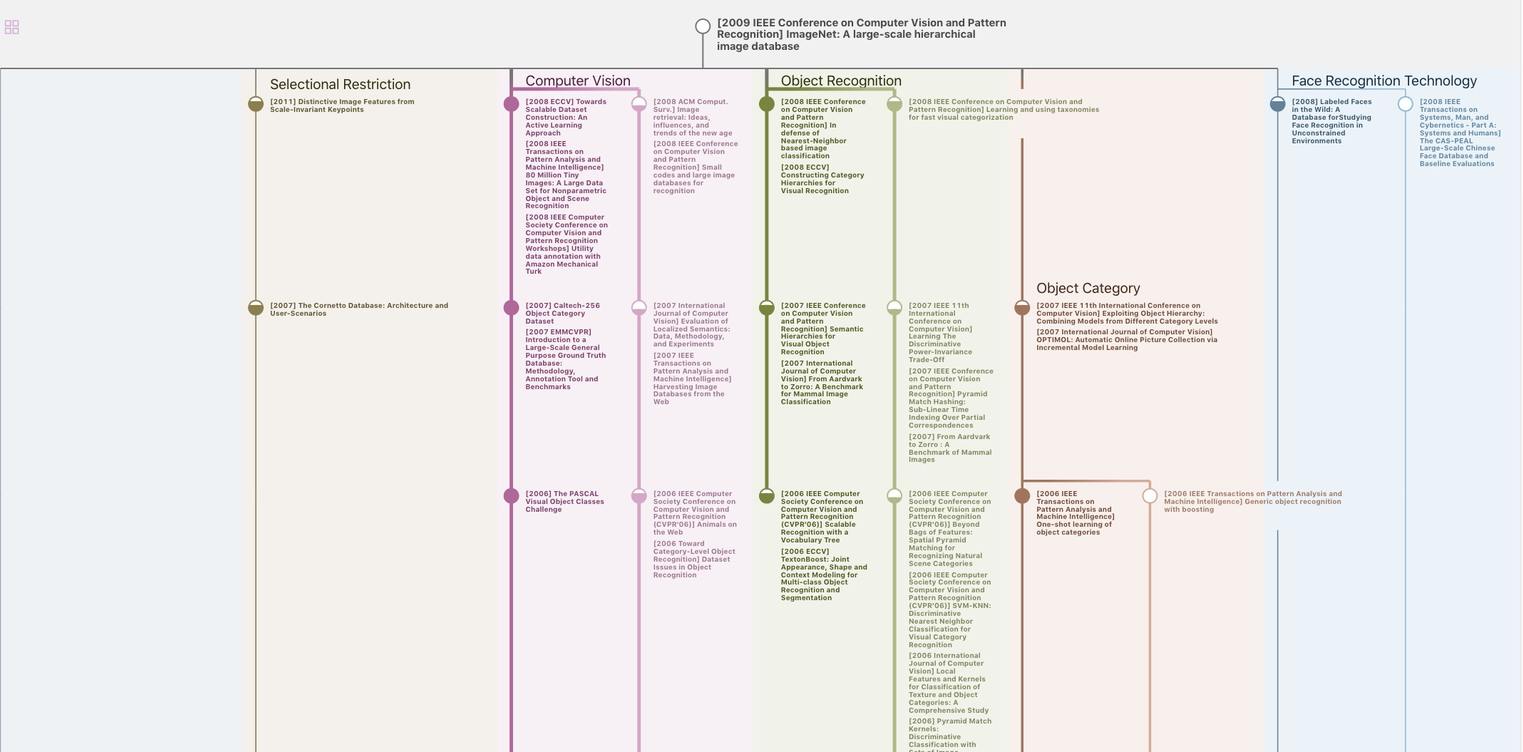
生成溯源树,研究论文发展脉络
Chat Paper
正在生成论文摘要