Time-delayed Multivariate Time Series Predictions.
SDM(2023)
摘要
A major issue with real-time monitoring is to collect complete data. Hardware or software failures, network issues or, more frequently, time delays can disrupt such a collection. This results in having two versions of the same information: one in real-time but with potentially missing data, and the another, albeit complete, is delayed. Many works have studied how to handle missing data for classification and prediction. However, to the best of our knowledge, they do not consider how to leverage the delayed complete data to assist in learning the representation of real-time available data with missing values. This is despite the fact that the delayed complete data contain all the information (e.g., periodicities and trends). In this paper, we propose a framework to enhance the representation learning of the real-time available data by aligning the representation of past real-time but with missing data to that of past delayed but complete data. We test both a distance metric and contrastive learning to achieve this alignment. We implement our framework on a Transformer-based model and experiment it on three datasets. The efficiency of our solution is evaluated against seven baselines and considering four distinct patterns of missing data. Our experiments show that this proposal has a significant improvement in prediction accuracy (5.21% on average) over the baselines.Keywordsmultivariate time series predictiontime delayrepresentation alignmentcontrastive learning
更多查看译文
关键词
time-delayed
AI 理解论文
溯源树
样例
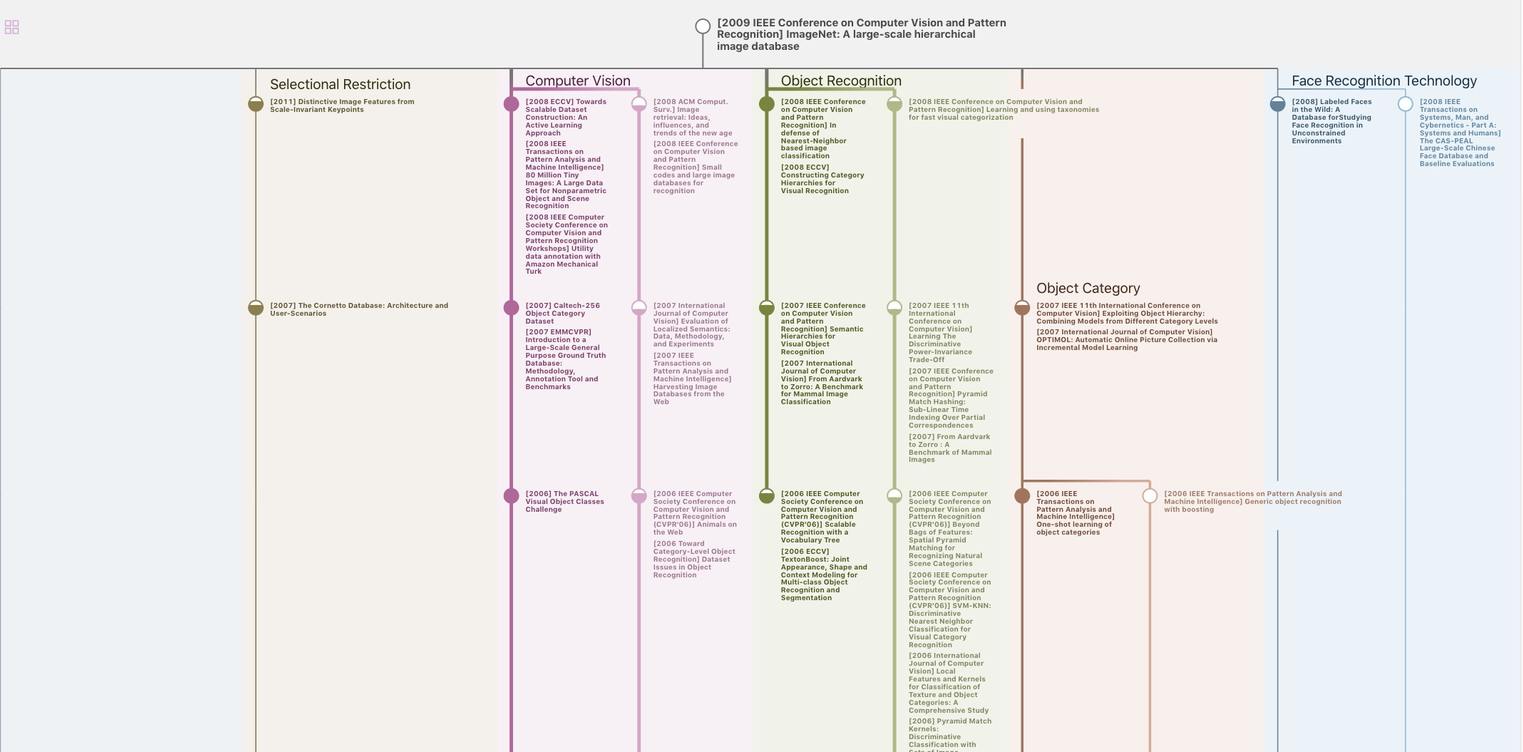
生成溯源树,研究论文发展脉络
Chat Paper
正在生成论文摘要