Hierarchical Reinforced Urban Planning: Jointly Steering Region and Block Configurations.
SDM(2023)
摘要
With the explosive accumulation of urban geographic, mobile, and IoT service data, AI-assisted automated urban planning, with a goal of configuring land-uses, has become an emerging interdisciplinary topic for smart cities. Existing literature mostly views urban planning as a generative task from the perspective of generating land-use configuration images. Such perspective is limited by two issues: 1) hierarchical planning dependency across multi scales : there are hierarchical dependencies between region-level urban function configurations and block-level building configurations. 2) sequential planning dependency within a scale : when planning the buildings of a place, planning a shopping mall can impose constraints on planning subsequent Points of Interest (POIs). In response, we propose a new perspective of formulating urban planning as a hierarchical decision process. That is, given a target region with many geographic blocks, a machine planner firstly selects the optimized urban function portfolios, thereafter, sequentially selects the most appropriate POI for each block based on its urban functions and previously-placed POIs over planning steps. We reformulate this decision process into a hierarchical reinforcement learning task and develop a novel hierarchical reinforced urban planning framework. This framework includes two components: 1) In region-level configuration, we present an actor- critic based method to overcome the challenge of weak reward feedback in planning the urban functions of regions. 2) In block-level configuration, we propose a single-agent iterative POI allocation strategy to model dependencies between POIs and urban functions, and between current and previous POIs. Finally, we present extensive experimental results on real-world urban data to demonstrate the enhanced performances of the “planning as hierarchical decision process” perspective and the reinforced planning model.
更多查看译文
关键词
urban planning,steering region,block configurations
AI 理解论文
溯源树
样例
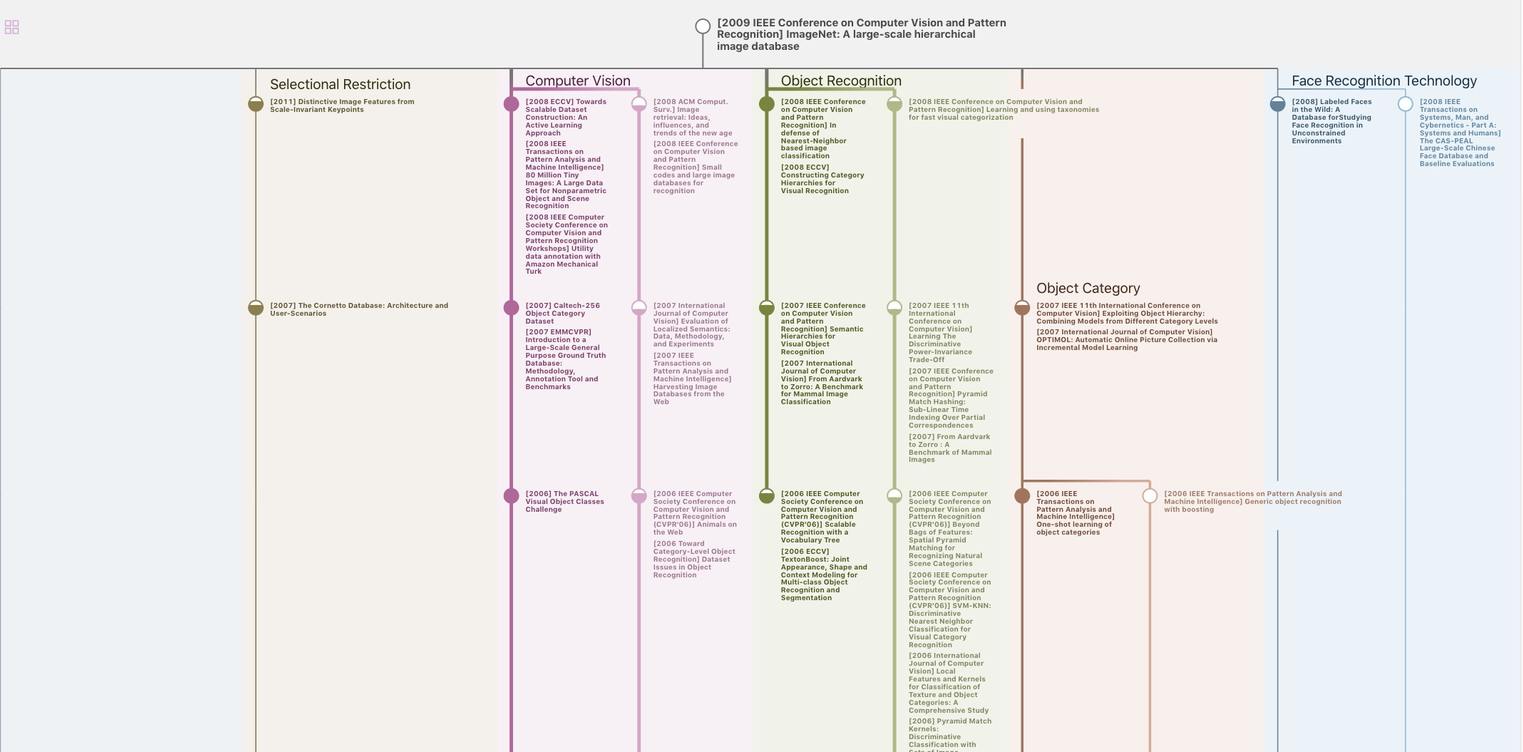
生成溯源树,研究论文发展脉络
Chat Paper
正在生成论文摘要