On Improving Fairness of AI Models with Synthetic Minority Oversampling Techniques.
SDM(2023)
摘要
Biased AI models result in unfair decisions. In response, a number of algorithmic solutions have been engineered to mitigate bias, among which the Synthetic Minority Oversampling Technique (SMOTE) has been studied, to an extent. Although the SMOTE technique and its variants have great potentials to help improve fairness, there is little theoretical justification for its success. In addition, formal error and fairness bounds are not clearly given. This paper attempts to address both issues. We prove and demonstrate that synthetic data generated by oversampling underrepresented groups can mitigate algorithmic bias in AI models, while keeping the predictive errors bounded. We further compare this technique to the existing state-of-the-art fair AI techniques on five datasets using a variety of fairness metrics. We show that this approach can effectively improve fairness even when there is a significant amount of label and selection bias, regardless of the baseline AI algorithm.KeywordsAI fairnesssensitive featuresynthetic dataSMOTE
更多查看译文
关键词
synthetic minority oversampling techniques,improving fairness,fairness models
AI 理解论文
溯源树
样例
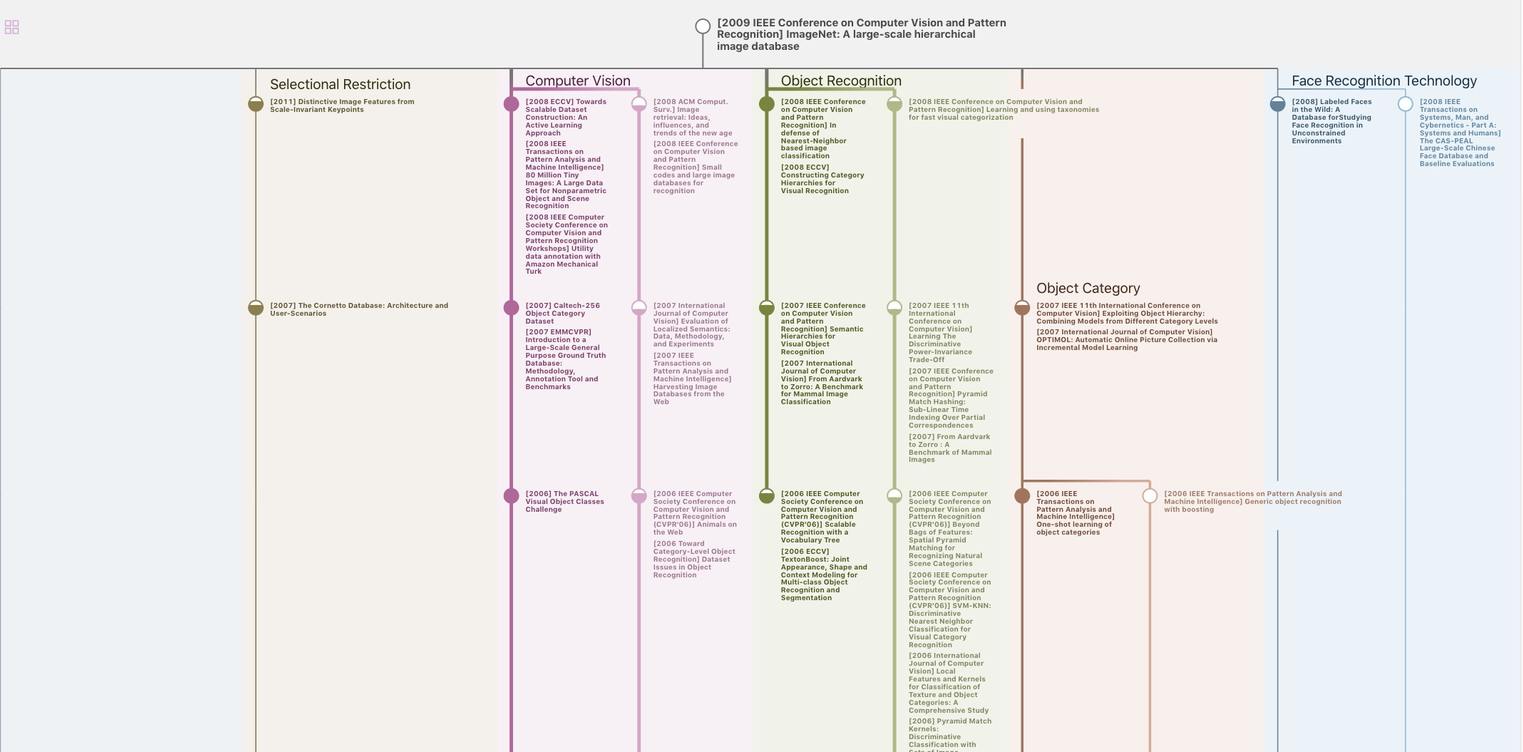
生成溯源树,研究论文发展脉络
Chat Paper
正在生成论文摘要