Extension of the Dip-test Repertoire -- Efficient and Differentiable p-value Calculation for Clustering
SDM(2023)
Abstract
Over the last decade, the Dip-test of unimodality has gained increasing
interest in the data mining community as it is a parameter-free statistical
test that reliably rates the modality in one-dimensional samples. It returns a
so called Dip-value and a corresponding probability for the sample's
unimodality (Dip-p-value). These two values share a sigmoidal relationship.
However, the specific transformation is dependent on the sample size. Many
Dip-based clustering algorithms use bootstrapped look-up tables translating
Dip- to Dip-p-values for a certain limited amount of sample sizes. We propose a
specifically designed sigmoid function as a substitute for these
state-of-the-art look-up tables. This accelerates computation and provides an
approximation of the Dip- to Dip-p-value transformation for every single sample
size. Further, it is differentiable and can therefore easily be integrated in
learning schemes using gradient descent. We showcase this by exploiting our
function in a novel subspace clustering algorithm called Dip'n'Sub. We
highlight in extensive experiments the various benefits of our proposal.
MoreTranslated text
Key words
clustering,dip-test,p-value
AI Read Science
Must-Reading Tree
Example
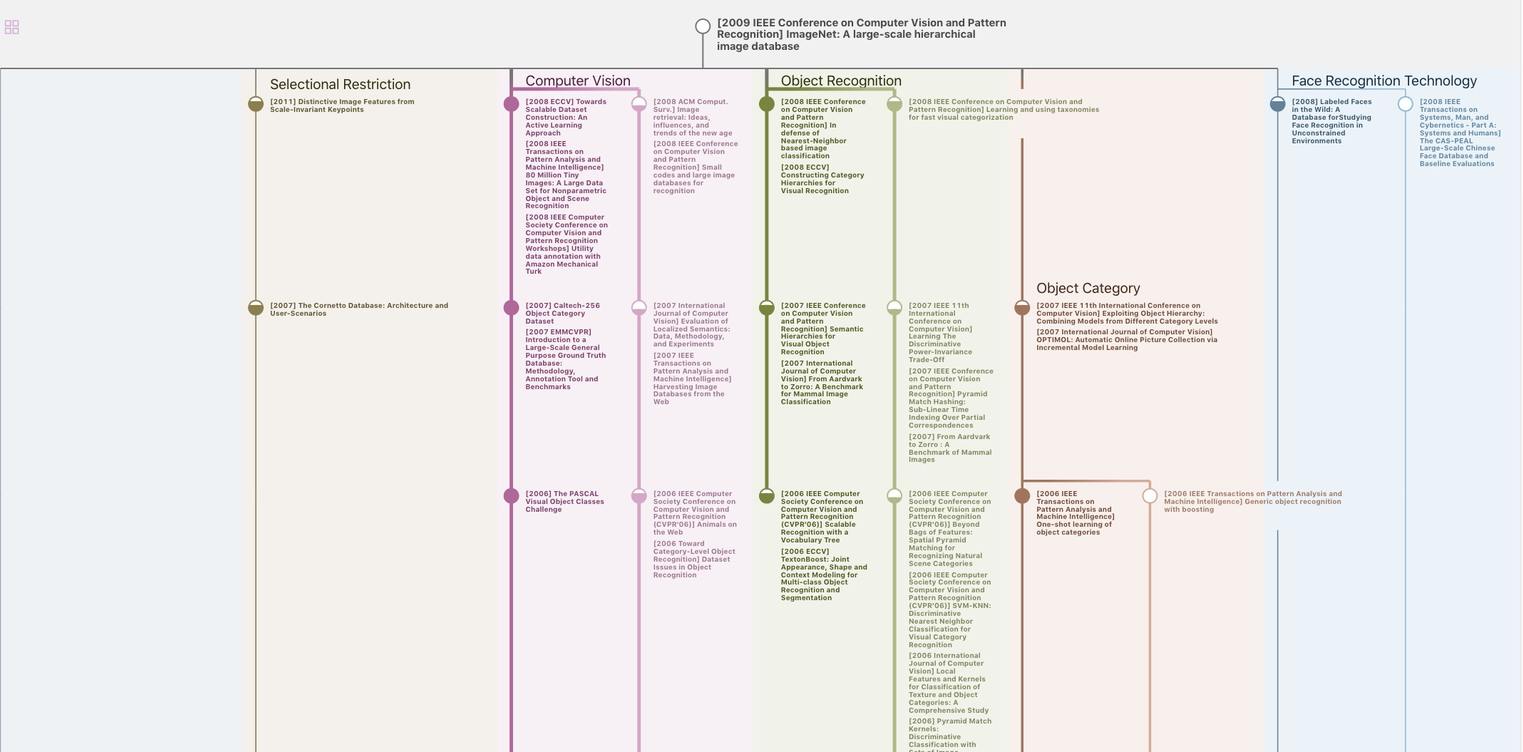
Generate MRT to find the research sequence of this paper
Chat Paper
Summary is being generated by the instructions you defined