PLST: A Pseudo-labels with a Smooth Transition Strategy for Medical Site Adaptation
DOMAIN ADAPTATION AND REPRESENTATION TRANSFER, DART 2023(2024)
摘要
This study addresses the challenge of medical image segmentation when transferring a pre-trained model from one medical site to another without access to pre-existing labels. The method involves utilizing a self-training approach by generating pseudo-labels of the target domain data. To do so, a strategy that is based on a smooth transition between domains is implemented where we initially feed easy examples to the network and gradually increase the difficulty of the examples. To identify the level of difficulty, we use a binary classifier trained to distinguish between the two domains by considering that target images easier if they are classified as source examples. We demonstrate the improved performance of our method on a range of medicalMRI image segmentation tasks. When integrating our approach as a post-processing step in several standard Unsupervised Domain Adaptation (UDA) algorithms, we consistently observed significant improvements in the segmentation results on test images from the target site.
更多查看译文
关键词
domain shift,domain adaptation,self-training,pseudo-labels
AI 理解论文
溯源树
样例
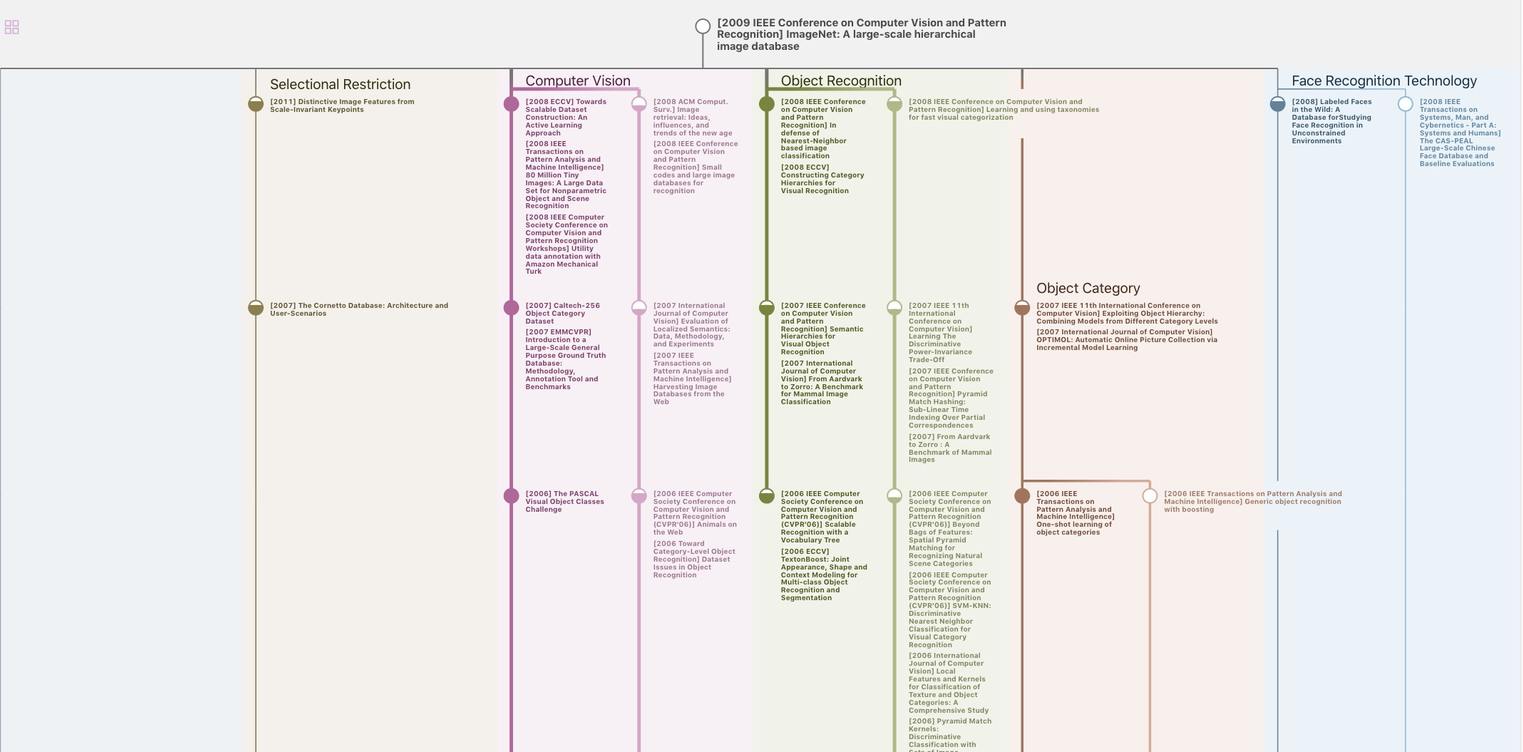
生成溯源树,研究论文发展脉络
Chat Paper
正在生成论文摘要