Unsupervised Liver Tumor Segmentation with Pseudo Anomaly Synthesis
SIMULATION AND SYNTHESIS IN MEDICAL IMAGING, SASHIMI 2023(2023)
Abstract
Liver lesion segmentation is a challenging task. Liver lesions often appear as regional heterogeneity in various shapes and intensities, while collecting a comprehensive dataset for supervised learning is costly. To address this issue, this study formulates unsupervised liver tumor segmentation as an anomaly segmentation problem and presents a pseudo-supervised anomaly segmentation solution with synthetic anomalies. In this regard, we investigate two fundamental, yet under-explored questions: (1) how to generate anomalies? and (2) how to address a covariant shift between synthesis data and real tumor samples in model training? To the first question, instead of fabricating anomalies approximating the known abnormal patterns, we propose to generate anomalies spreading over a broader spectrum to encourage a model to learn the cluster boundary of normal samples. Our rationale toward the second question suggests light training on synthesis data for model generalizability. Based on these insights, this study incorporates a random-shaped anomaly synthesis module and two-stage training strategy into the DRAEM architecture for unsupervised liver tumor segmentation. Experiments on the public benchmark show that the proposed method trained on various synthetic anomalies has good generalizability on real tumor and achieves a comparable performance to prior arts. Our code is available at: https://github.com/nono-zz/LiTs-Segmentation.
MoreTranslated text
Key words
Lesion Segmentation,Unsupervised Learning,Anomaly Synthesis,Two-stage Training,CT
AI Read Science
Must-Reading Tree
Example
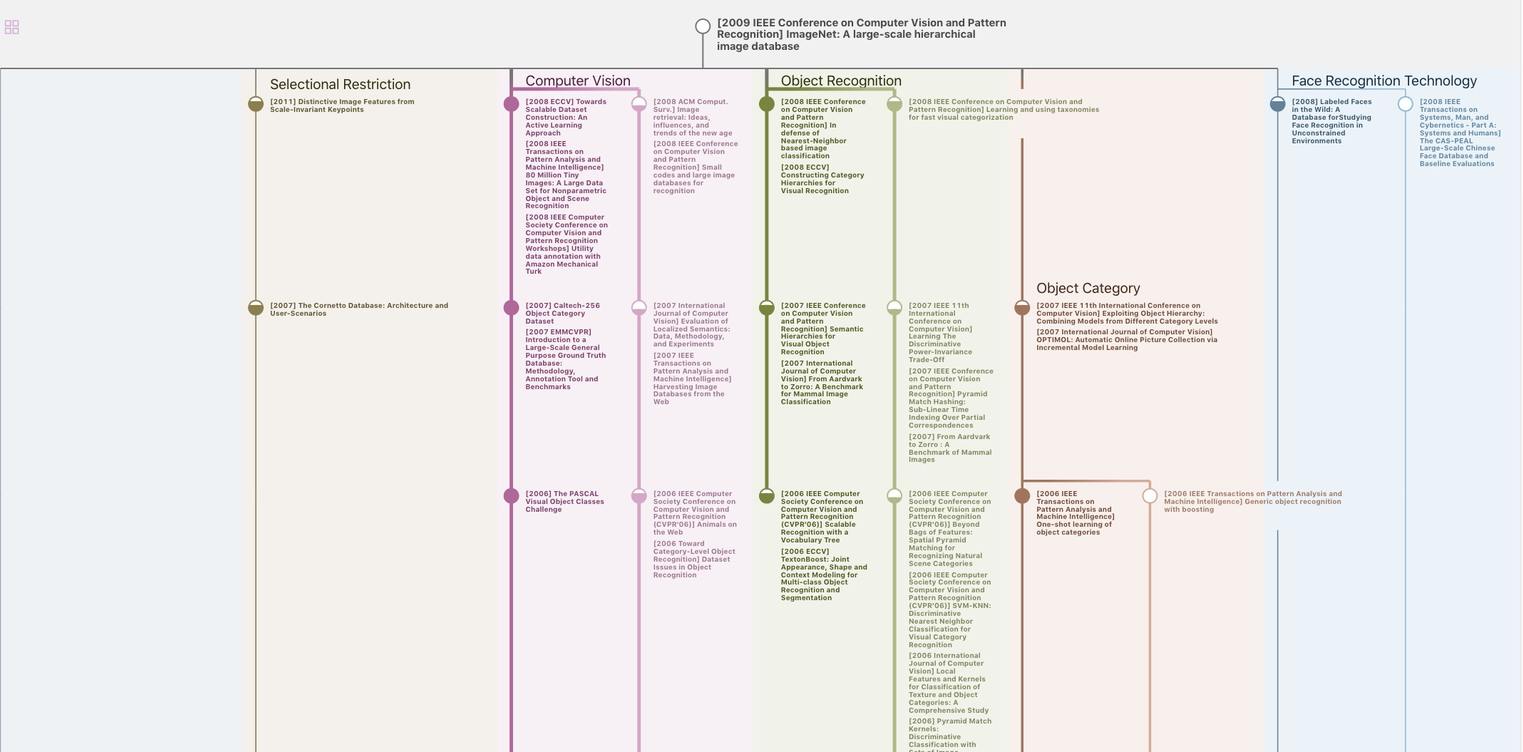
Generate MRT to find the research sequence of this paper
Chat Paper
Summary is being generated by the instructions you defined