Graph-Theoretic Automatic Lesion Tracking and Detection of Patterns of Lesion Changes in Longitudinal CT Studies
MICCAI (5)(2023)
Abstract
Radiological follow-up of oncological patients requires the analysis and comparison of multiple unregistered scans acquired every few months. This process is currently partial, time-consuming and subject to variability. We present a new, generic, graph-based method for tracking individual lesion changes and detecting patterns in the evolution of lesions over time. The tasks are formalized as graph-theoretic problems in which lesions are vertices and edges are lesion pairings computed by overlap-based lesion matching. We define seven individual lesion change classes and five lesion change patterns that fully summarize the evolution of lesions over time. They are directly computed from the graph properties and its connected components with graph-based methods. Experimental results on lung (83 CTs from 19 patients) and liver (77 CECTs from 18 patients) datasets with more than two scans per patient yielded an individual lesion change class accuracy of 98% and 85%, and identification of patterns of lesion change with an accuracy of 96% and 76%, respectively. Highlighting unusual lesion labels and lesion change patterns in the graph helps radiologists identify overlooked or faintly visible lesions. Automatic lesion change classification and pattern detection in longitudinal studies may improve the accuracy and efficiency of radiological interpretation and disease status evaluation.
MoreTranslated text
Key words
longitudinal follow-up,lesion matching,lesion change analysis
AI Read Science
Must-Reading Tree
Example
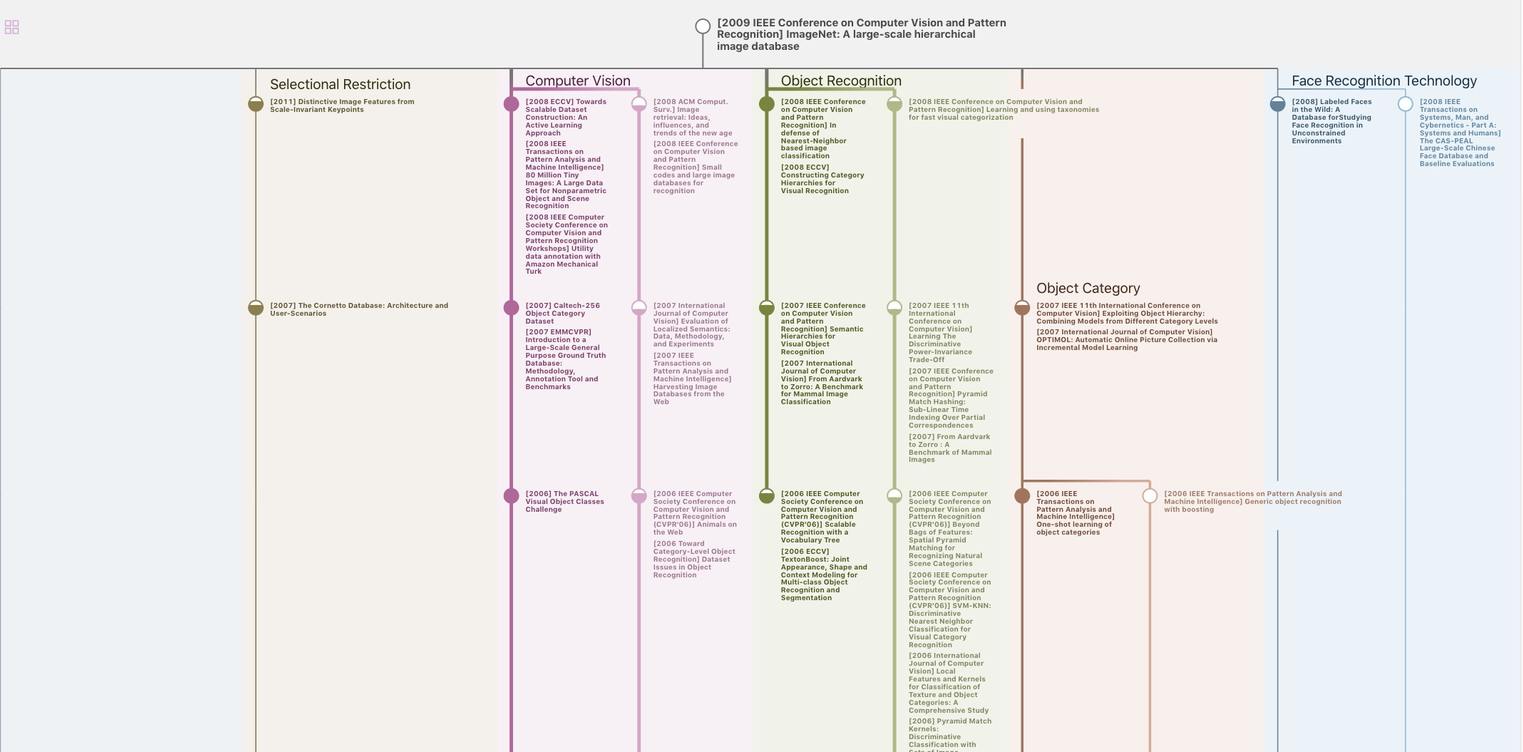
Generate MRT to find the research sequence of this paper
Chat Paper
Summary is being generated by the instructions you defined