Geometry-Invariant Abnormality Detection
MEDICAL IMAGE COMPUTING AND COMPUTER ASSISTED INTERVENTION, MICCAI 2023, PT I(2023)
摘要
Cancer is a highly heterogeneous condition best visualised in positron emission tomography. Due to this heterogeneity, a general-purpose cancer detection model can be built using unsupervised learning anomaly detection models. While prior work in this field has showcased the efficacy of abnormality detection methods (e.g. Transformer-based), these have shown significant vulnerabilities to differences in data geometry. Changes in image resolution or observed field of view can result in inaccurate predictions, even with significant data pre-processing and augmentation. We propose a new spatial conditioning mechanism that enables models to adapt and learn from varying data geometries, and apply it to a state-of-the-art Vector-Quantized Variational Autoencoder + Transformer abnormality detection model. We showcase that this spatial conditioning mechanism statistically-significantly improves model performance on whole-body data compared to the same model without conditioning, while allowing the model to perform inference at varying data geometries.
更多查看译文
关键词
abnormality,detection,geometry-invariant
AI 理解论文
溯源树
样例
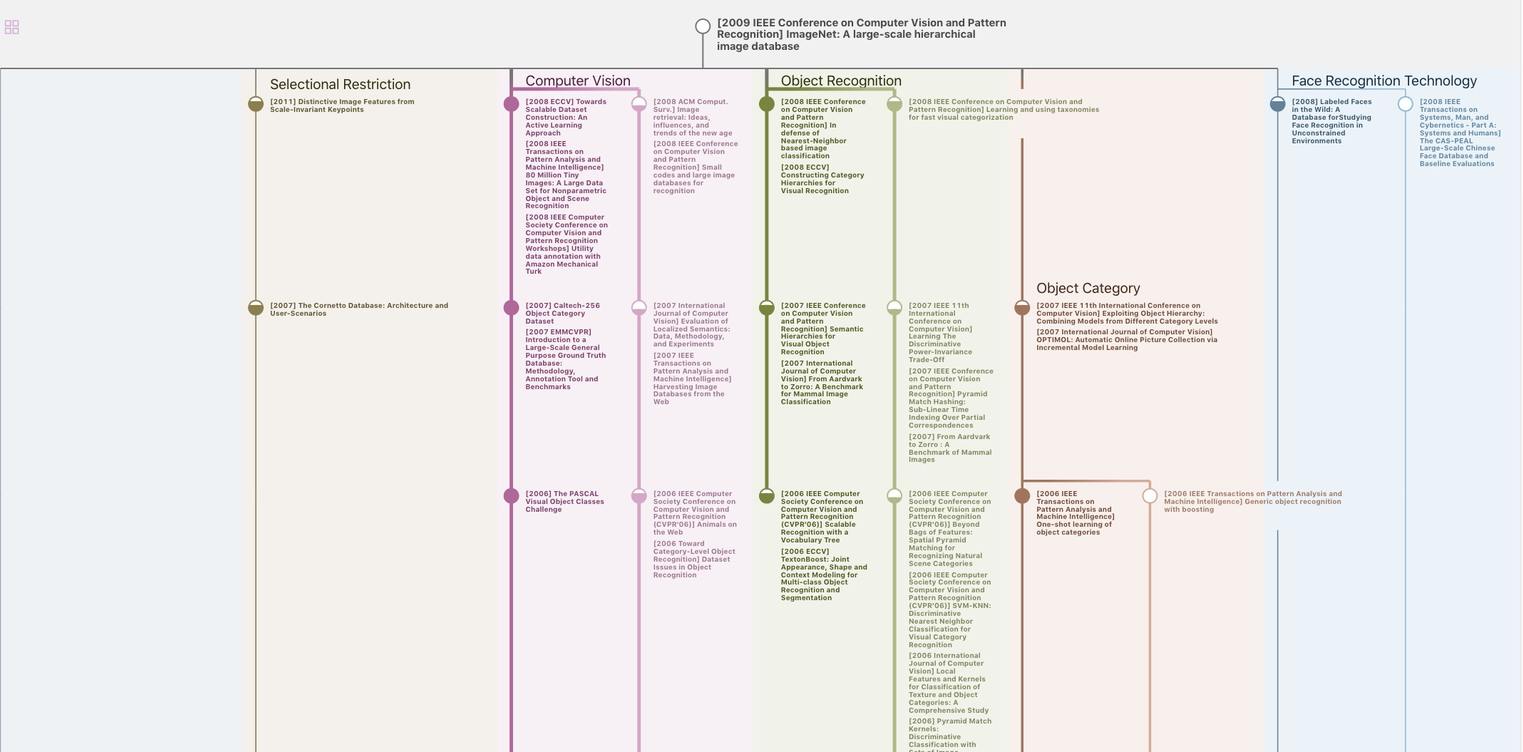
生成溯源树,研究论文发展脉络
Chat Paper
正在生成论文摘要