Style-Based Manifold for Weakly-Supervised Disease Characteristic Discovery
MEDICAL IMAGE COMPUTING AND COMPUTER ASSISTED INTERVENTION, MICCAI 2023, PT V(2023)
摘要
In Alzheimer's Disease (AD), interpreting tissue changes is key to discovering disease characteristics. However, AD-induced brain atrophy can be difficult to observe without Cognitively Normal (CN) reference images, and collecting co-registered AD and CN images at scale is not practical. We propose Disease Discovery GAN (DiDiGAN), a style-based network that can create representative reference images for disease characteristic discovery. DiDiGAN learns a manifold of disease-specific style codes. In the generator, these style codes are used to "stylize" an anatomical constraint into synthetic reference images (for various disease states). The constraint in this case underpins the high-level anatomical structure upon which disease features are synthesized. Additionally, DiDiGAN's manifold is smooth such that seamless disease state transitions are possible via style interpolation. Finally, to ensure the generated reference images are anatomically correlated across disease states, we incorporate anti-aliasing inspired by StyleGAN3 to enforce anatomical correspondence. We test DiDiGAN on the ADNI dataset involving CN and AD magnetic resonance images (MRIs), and the generated reference AD and CN images reveal key AD characteristics (hippocampus shrinkage, ventricular enlargement, cortex atrophies). Moreover, by interpolating DiDiGAN's manifold, smooth CN-AD transitions were acquired further enhancing disease visualization. In contrast, other methods in the literature lack such dedicated disease manifolds and fail to synthesize usable reference images for disease characteristic discovery.
更多查看译文
关键词
Disease learning,Weak supervision,Alzheimer's Disease
AI 理解论文
溯源树
样例
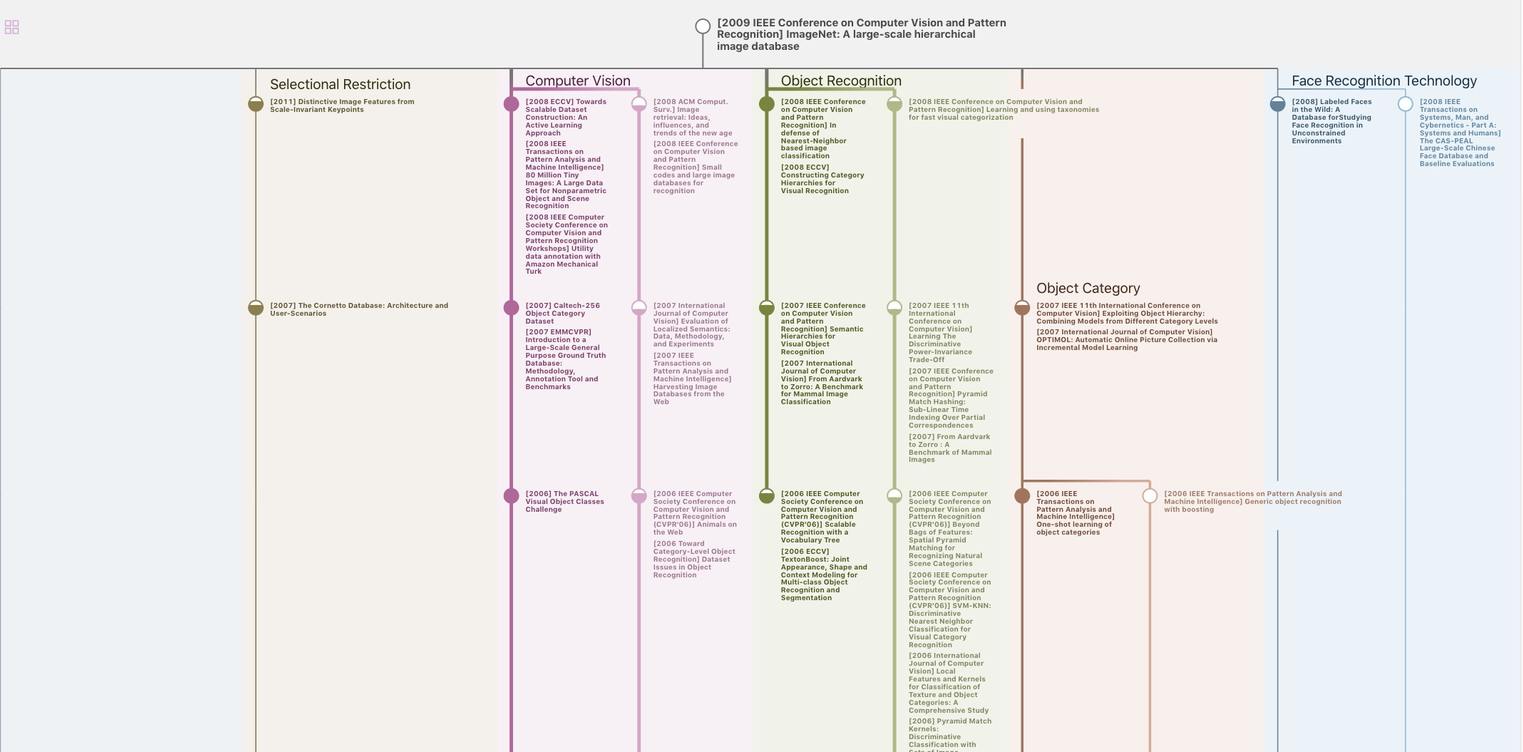
生成溯源树,研究论文发展脉络
Chat Paper
正在生成论文摘要