Self-adaptive Adversarial Training for Robust Medical Segmentation
MEDICAL IMAGE COMPUTING AND COMPUTER ASSISTED INTERVENTION, MICCAI 2023, PT III(2023)
Abstract
Adversarial training has been demonstrated to be one of the most effective approaches to training deep neural networks that are robust to malicious perturbations. Research on effectively applying it to produce robust 3D medical image segmentation models is ongoing. While few empirical studies have been done in this area, developing effective adversarial training methods for complex segmentation models and high-volume 3D examples is challenging and requires theoretical support. In this paper, we consider the robustness of 3D segmentation tasks from a PAC-Bayes generalisation perceptive and show that reducing the trained models' Lipschitz constant benefits the models' robustness performance. Demonstrating by empirical investigation, we show that adjusting the adversarial iteration can help to reduce the model's Lipschitz constant, enabling a self-adaptive adversarial training strategy. Empirical studies on the medical segmentation decathlon dataset have been done to demonstrate the efficiency of the proposed adversarial training method. Our implementation is available at https://github.com/TrustAI/SEAT.
MoreTranslated text
Key words
Medical Image Segmentation,Adversarial Training
AI Read Science
Must-Reading Tree
Example
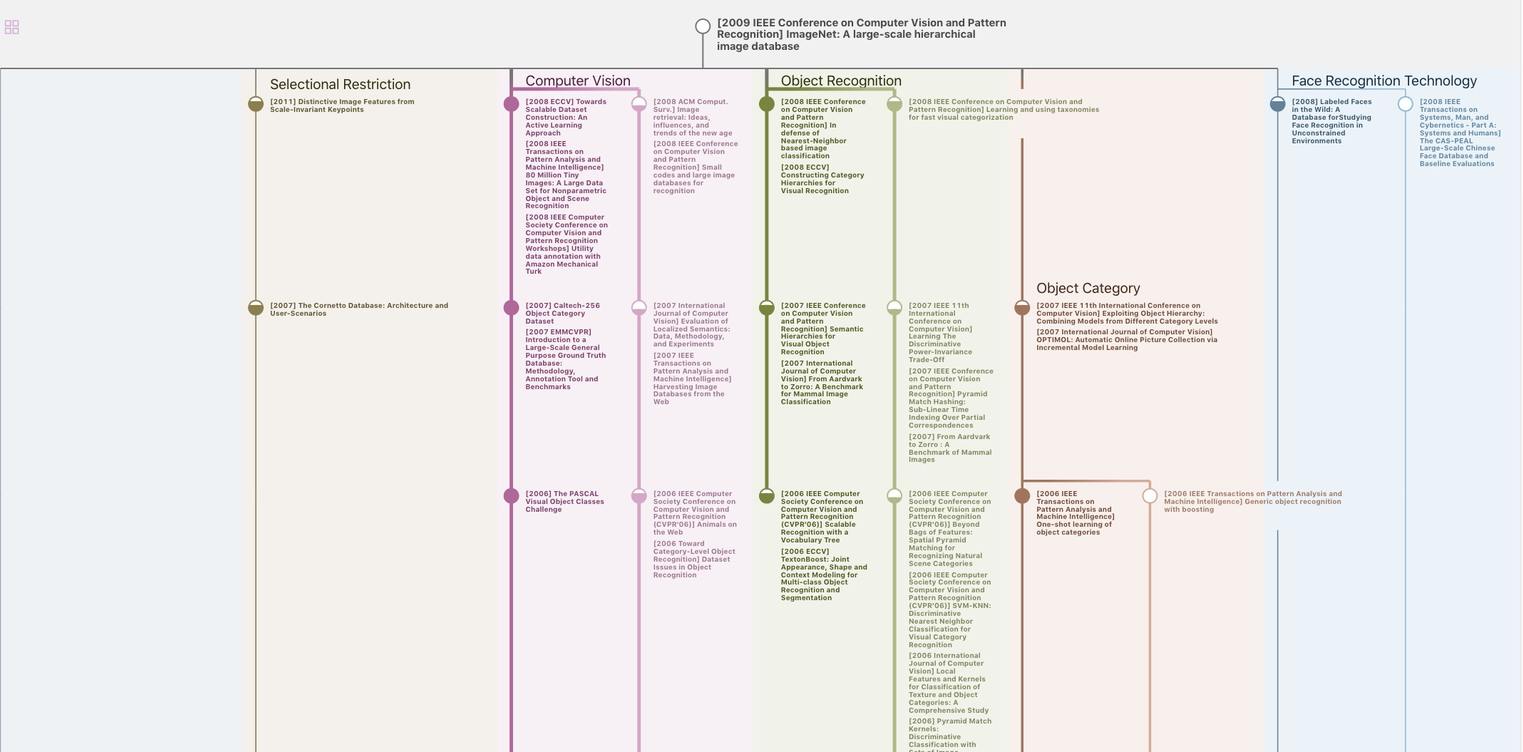
Generate MRT to find the research sequence of this paper
Chat Paper
Summary is being generated by the instructions you defined