StructuRegNet: Structure-Guided Multimodal 2D-3D Registration
MEDICAL IMAGE COMPUTING AND COMPUTER ASSISTED INTERVENTION, MICCAI 2023, PT X(2023)
Abstract
Multimodal 2D-3D co-registration is a challenging problem with numerous clinical applications, including improved diagnosis, radiation therapy, or interventional radiology. In this paper, we present StructuRegNet, a deep-learning framework that addresses this problem with three novel contributions. First, we combine a 2D-3D deformable registration network with an adversarial modality translation module, allowing each block to benefit from the signal of the other. Second, we solve the initialization challenge for 2D-3D registration by leveraging tissue structure through cascaded rigid areas guidance and distance field regularization. Third, StructuRegNet handles out-of-plane deformation without requiring any 3D reconstruction thanks to a recursive plane selection. We evaluate the quantitative performance of StructuRegNet for head and neck cancer between 3D CT scans and 2D histopathological slides, enabling pixel-wise mapping of low-quality radiologic imaging to gold-standard tumor extent and bringing biological insights toward homogenized clinical guidelines. Additionally, our method can be used in radiation therapy by mapping 3D planning CT into the 2D MR frame of the treatment day for accurate positioning and dose delivery. Our framework demonstrates superior results to traditional methods for both applications. It is versatile to different locations or magnitudes of deformation and can serve as a backbone for any relevant clinical context.
MoreTranslated text
Key words
Multimodal,Registration,2D-3D,Histopathology,Radiology
AI Read Science
Must-Reading Tree
Example
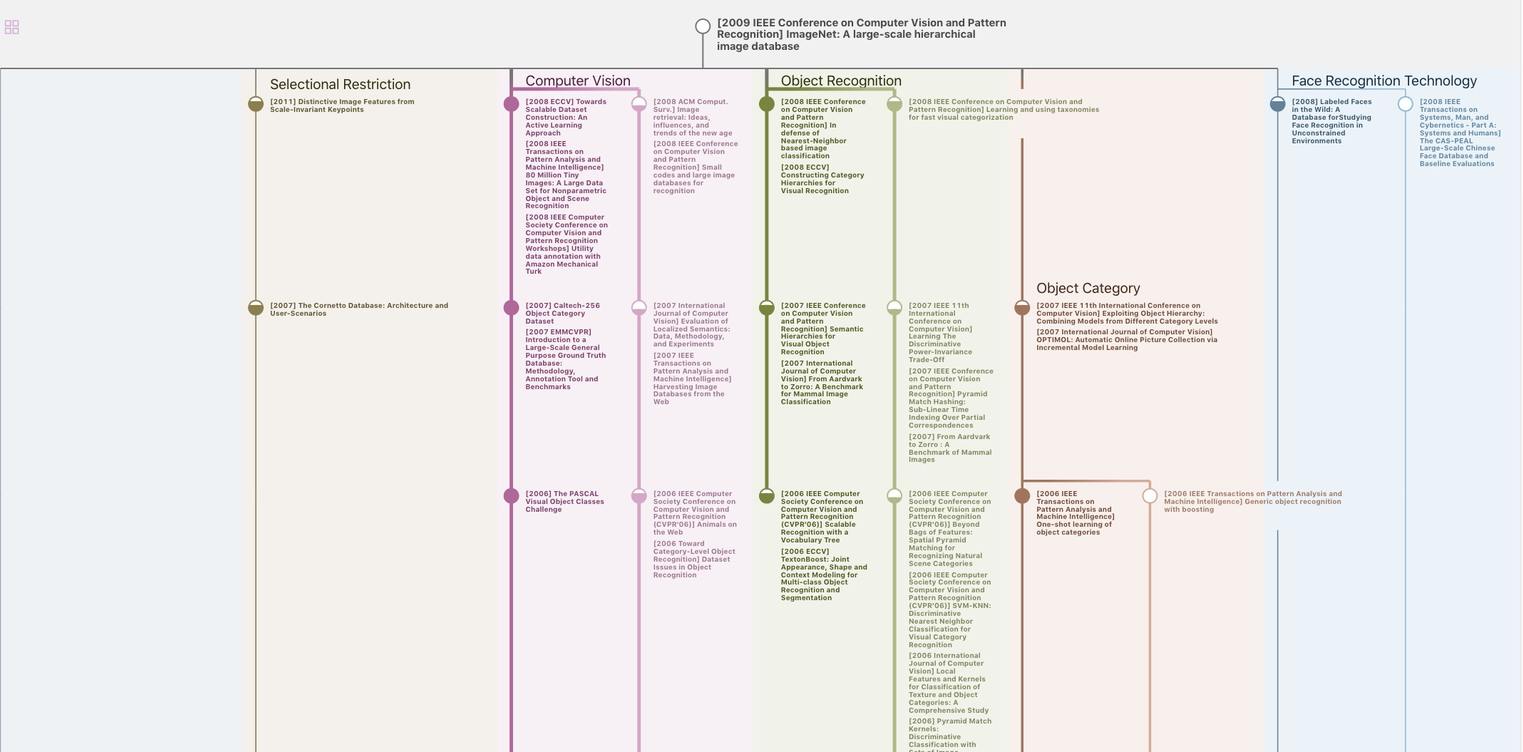
Generate MRT to find the research sequence of this paper
Chat Paper
Summary is being generated by the instructions you defined