Spatiotemporal Hub Identification in Brain Network by Learning Dynamic Graph Embedding on Grassmannian Manifold
MEDICAL IMAGE COMPUTING AND COMPUTER ASSISTED INTERVENTION, MICCAI 2023, PT II(2023)
摘要
Advancements in neuroimaging technology have made it possible to measure the connectivity evolution between different brain regions over time. Emerging evidence shows that some critical brain regions, known as hub nodes, play a significant role in updating brain network connectivity over time. However, current spatiotemporal hub identification is built on static network-based approaches, where hub regions are identified independently for each temporal brain network without considering their temporal consistency, and fails to align the evolution of hubs with changes in connectivity dynamics. To address this problem, we propose a novel spatiotemporal hub identificationmethod that utilizes dynamic graph embedding to distinguish temporal hubs from peripheral nodes. Specially, to preserve the time consistency information, we put the dynamic graph embedding learning upon a smooth physics model of network-to-network evolution, which mathematically expresses as a total variation of dynamic graph embedding with respect to time. A novel Grassmannian manifold optimization scheme is further introduced to learn the embeddings accurately and capture the time-varying topology of brain network. Experimental results on real data demonstrate the highest temporal consistency in hub identification, surpassing conventional approaches.
更多查看译文
AI 理解论文
溯源树
样例
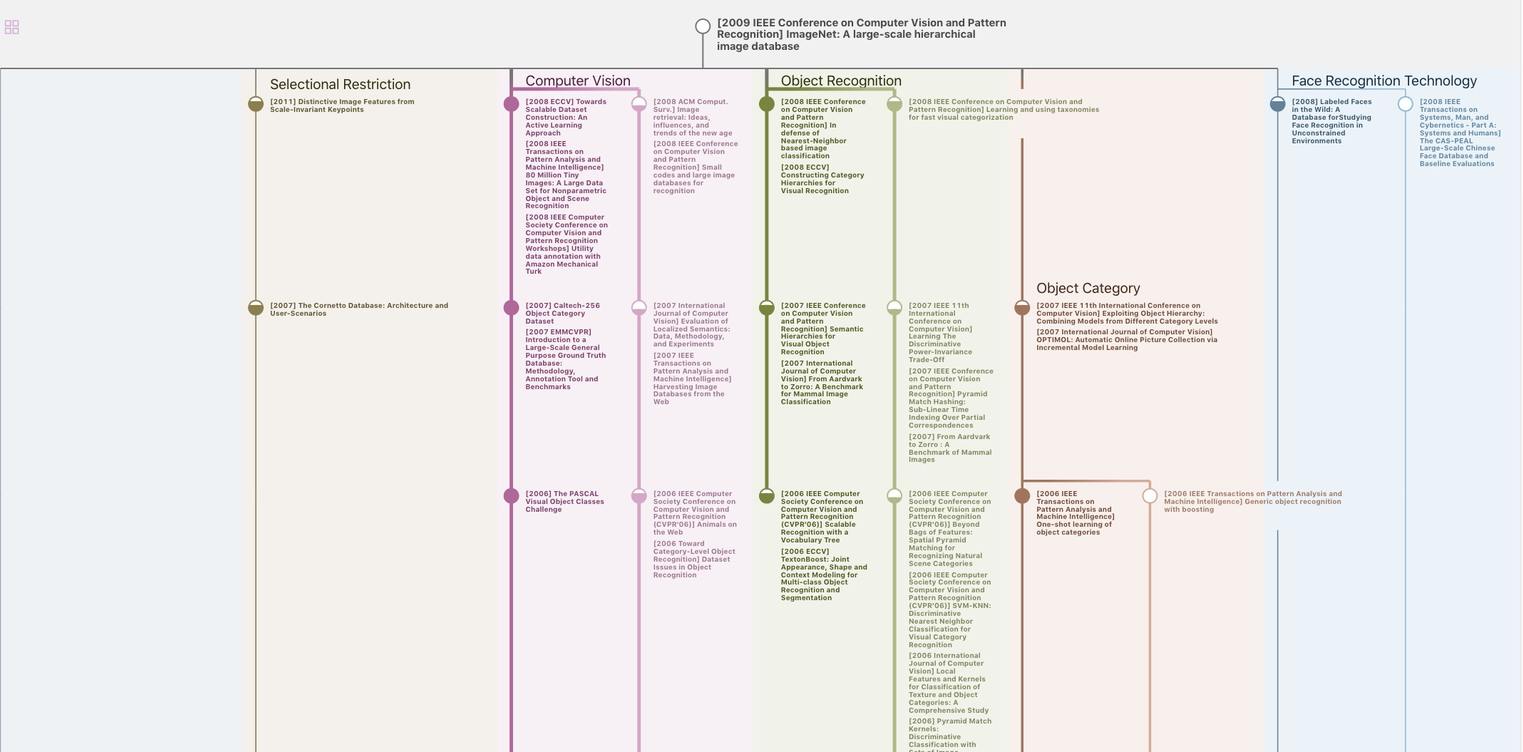
生成溯源树,研究论文发展脉络
Chat Paper
正在生成论文摘要