Minimal-Supervised Medical Image Segmentation via Vector Quantization Memory
MEDICAL IMAGE COMPUTING AND COMPUTER ASSISTED INTERVENTION, MICCAI 2023, PT III(2023)
摘要
Medical imaging segmentation is a critical key task for computer-assisted diagnosis and disease monitoring. However, collecting a large-scale medical dataset with well-annotation is time-consuming and requires domain knowledge. Reducing the number of annotations poses two challenges: obtaining sufficient supervision and generating high-quality pseudo labels. To address these, we propose a universal framework for annotation-efficient medical segmentation, which is capable of handling both scribble-supervised and point-supervised segmentation. Our approach includes an auxiliary reconstruction branch that provides more supervision and backwards sufficient gradients for learning visual representations. Besides, a novel pseudo label generation branch utilizes the Vector Quantization (VQ) bank to store texture-oriented and global features for generating pseudo labels. To boost the model training, we generate the high-quality pseudo labels by mixing the segmentation prediction and pseudo labels from the VQ bank. The experimental results on the ACDC MRI segmentation dataset demonstrate effectiveness of our designed method. We obtain a comparable performance (0.86 vs. 0.87 DSC score) with a few points.
更多查看译文
关键词
Annotation-efficient Learning,Vector Quantization
AI 理解论文
溯源树
样例
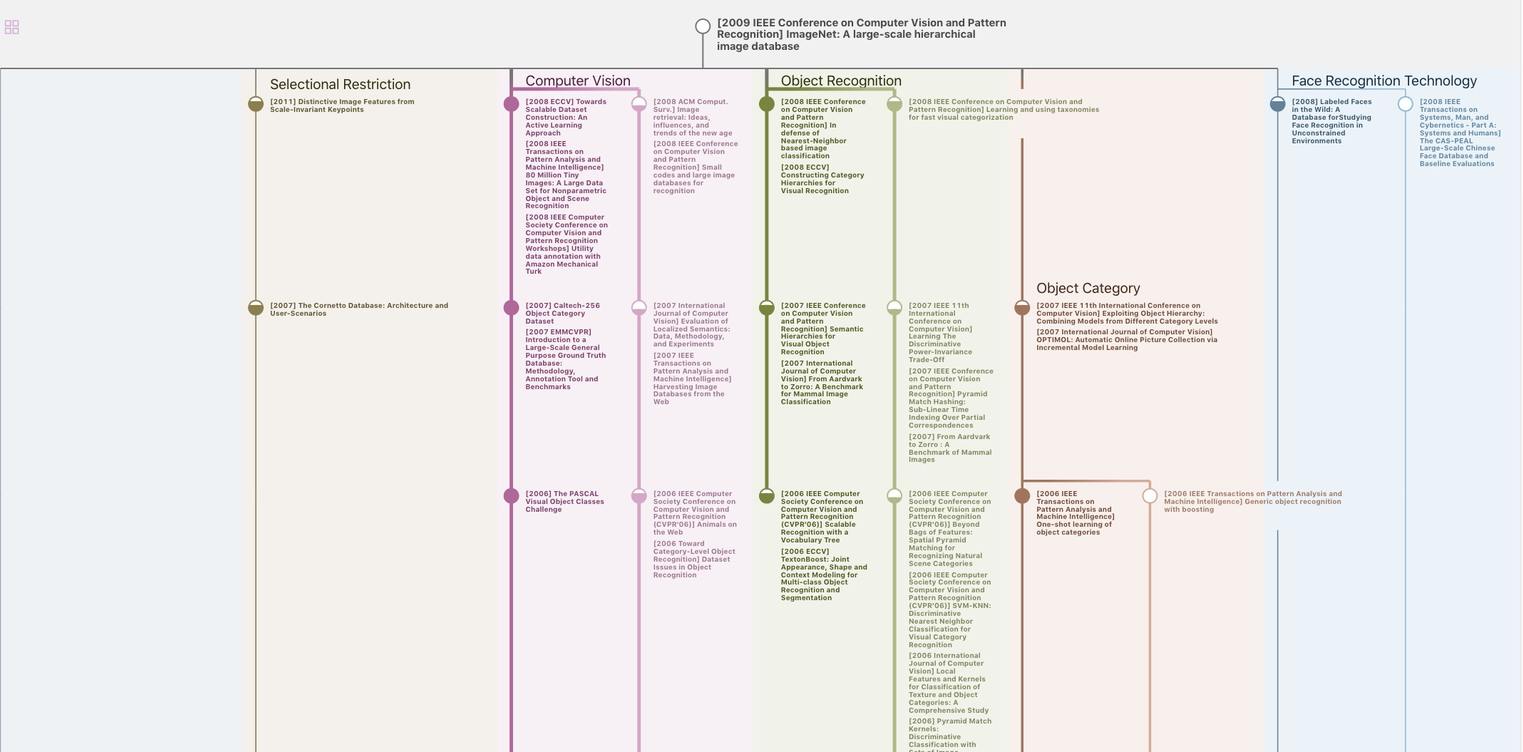
生成溯源树,研究论文发展脉络
Chat Paper
正在生成论文摘要