Auditing Unfair Biases in CNN-Based Diagnosis of Alzheimer's Disease
CLINICAL IMAGE-BASED PROCEDURES, FAIRNESS OF AI IN MEDICAL IMAGING, AND ETHICAL AND PHILOSOPHICAL ISSUES IN MEDICAL IMAGING, CLIP 2023, FAIMI 2023, EPIMI 2023(2023)
Abstract
Convolutional neural networks (CNN), while effective in medical diagnostics, have shown concerning biases against underrepresented patient groups. In this study, we provide an in-depth exploration of these biases in the realm of image-based Alzheimer's disease (AD) diagnosis using state-of-the-art CNNs, building upon and extending prior investigations. We dissect performance-based and calibrationbased biases across patient subgroups differentiated by sex, ethnicity, age, educational qualifications, and APOE4 status. Our findings reveal substantial disparities in model performance and calibration, underscoring the challenges intersectional identities impose. Such biases highlight the importance of fairness analysis in fostering equitable AI applications in the AD domain. Appropriate mitigation actions should be carried out to ensure that, those who need it, receive healthcare attention independently from the subgroup they belong to.
MoreTranslated text
Key words
fairness,bias,medical imaging,convolutional neural networks,Alzheimer's disease
AI Read Science
Must-Reading Tree
Example
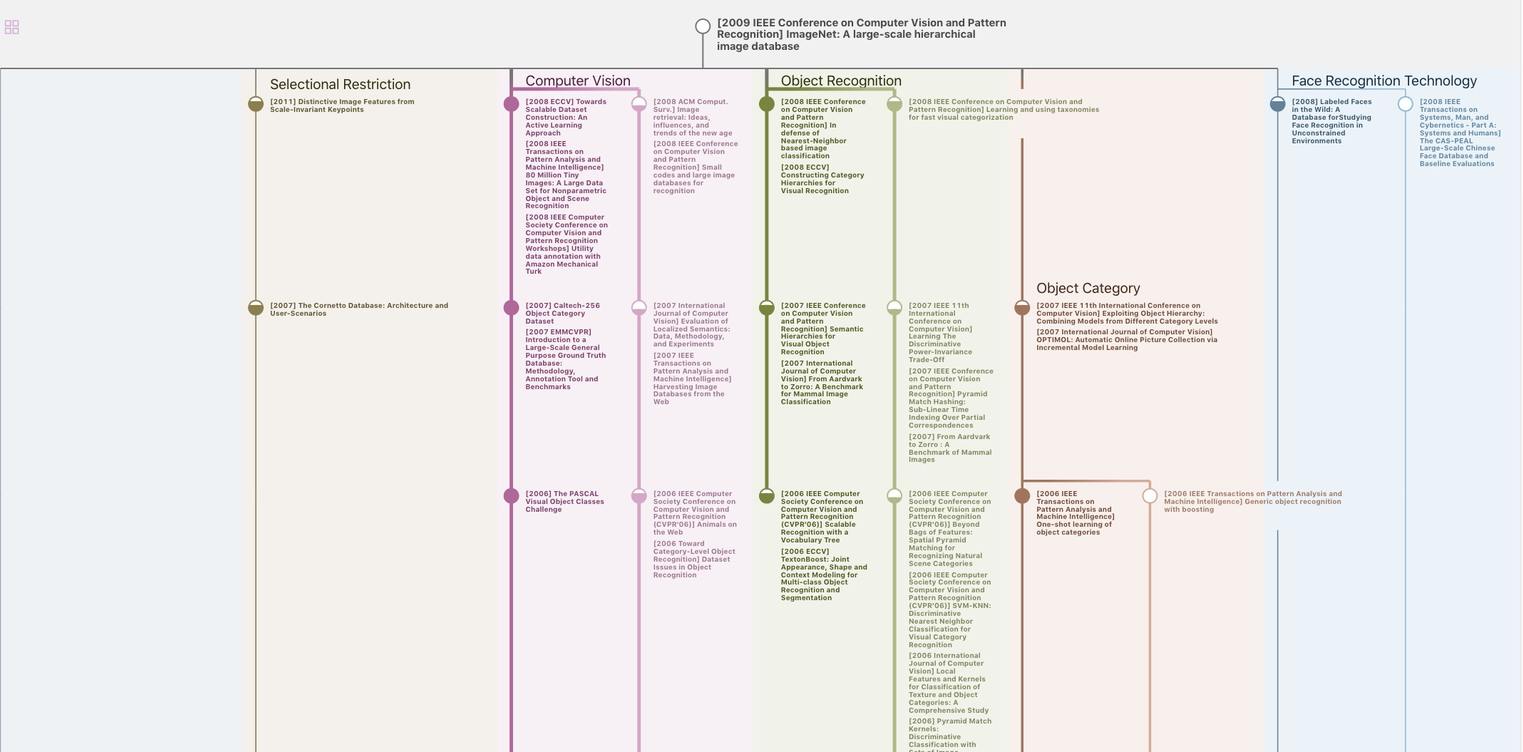
Generate MRT to find the research sequence of this paper
Chat Paper
Summary is being generated by the instructions you defined