Leveraging Shape and Spatial Information for Spontaneous Preterm Birth Prediction
SIMPLIFYING MEDICAL ULTRASOUND, ASMUS 2023(2023)
Abstract
Spontaneous preterm birth prediction from transvaginal ultrasound images is a challenging task of profound interest in gynecological obstetrics. Existing works are often validated on small datasets and may lack validation of model calibration and interpretation. In this paper, we present a comprehensive study of methods for predicting preterm birth from transvaginal ultrasound using a large clinical dataset. We propose a shape- and spatially-aware network that leverages segmentation predictions and pixel spacing information as additional input to enhance predictions. Our model demonstrates competitive performance on our benchmark, providing additional interpretation and achieving the highest performance across both clinical and machine learning baselines. Through our evaluation, we provide additional insights which we hope may lead to more accurate predictions of preterm births going forwards.
MoreTranslated text
Key words
Spontaneous Preterm Birth,Transvaginal Ultrasound,Transparency
AI Read Science
Must-Reading Tree
Example
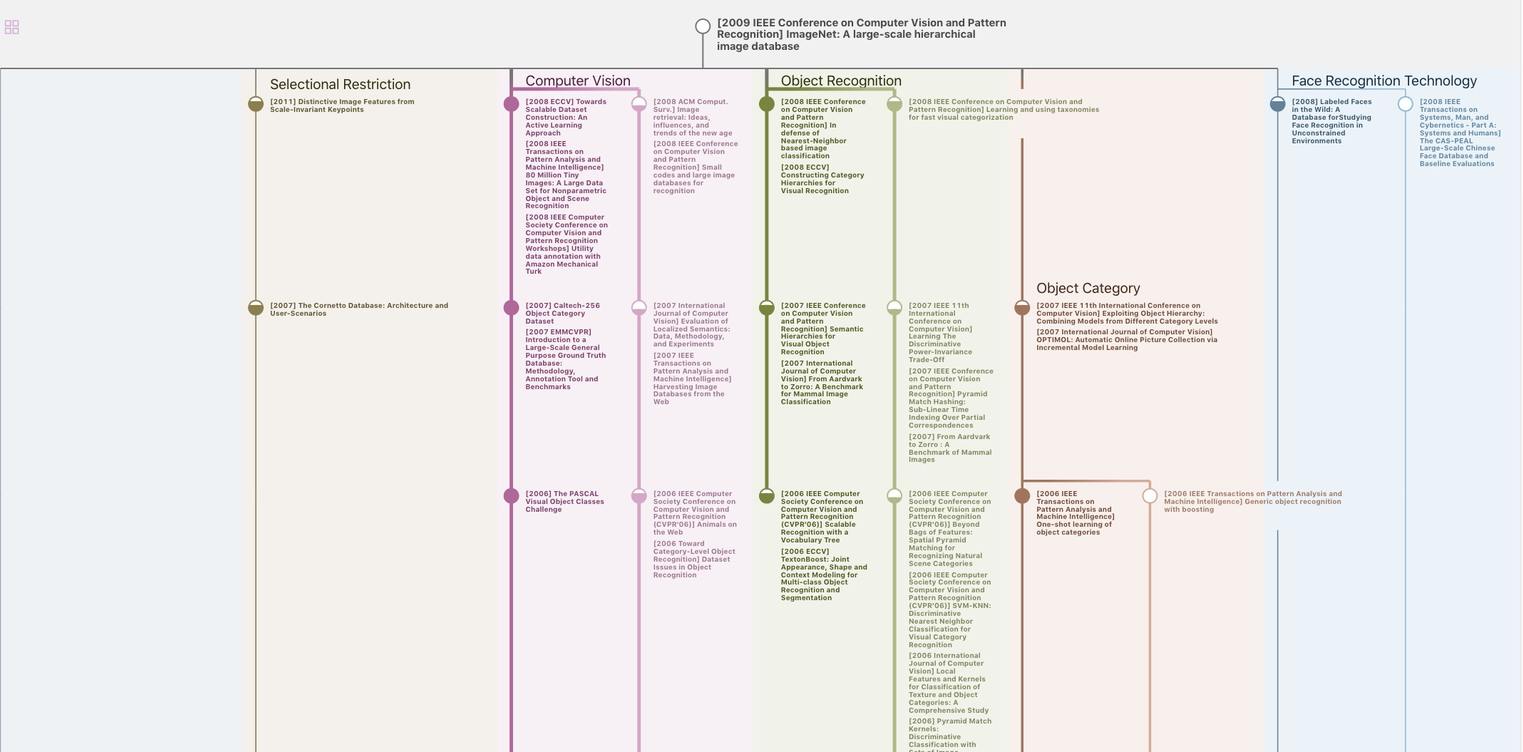
Generate MRT to find the research sequence of this paper
Chat Paper
Summary is being generated by the instructions you defined