Accurate Multi-contrast MRI Super-Resolution via a Dual Cross-Attention Transformer Network
MEDICAL IMAGE COMPUTING AND COMPUTER ASSISTED INTERVENTION, MICCAI 2023, PT X(2023)
摘要
Magnetic Resonance Imaging (MRI) is a critical imaging tool in clinical diagnosis, but obtaining high-resolution MRI images can be challenging due to hardware and scan time limitations. Recent studies have shown that using reference images from multi-contrast MRI data could improve super-resolution quality. However, the commonly employed strategies, e.g., channel concatenation or hard-attention based texture transfer, may not be optimal given the visual differences between multi-contrast MRI images. To address these limitations, we propose a new Dual Cross-Attention Multi-contrast Super Resolution (DCAMSR) framework. This approach introduces a dual cross-attention transformer architecture, where the features of the reference image and the upsampled input image are extracted and promoted with both spatial and channel attention in multiple resolutions. Unlike existing hard-attention based methods where only the most correlated features are sought via the highly down-sampled reference images, the proposed architecture is more powerful to capture and fuse the shareable information between the multi-contrast images. Extensive experiments are conducted on fastMRI knee data at high field and more challenging brain data at low field, demonstrating that DCAMSR can substantially outperform the state-of-the-art single-image and multi-contrast MRI super-resolution methods, and even remains robust in a self-referenced manner. The code for DCAMSR is avaliable at https://github.com/Solor-pikachu/DCAMSR.
更多查看译文
关键词
Magnetic resonance imaging,Super-resolution,Multi-contrast
AI 理解论文
溯源树
样例
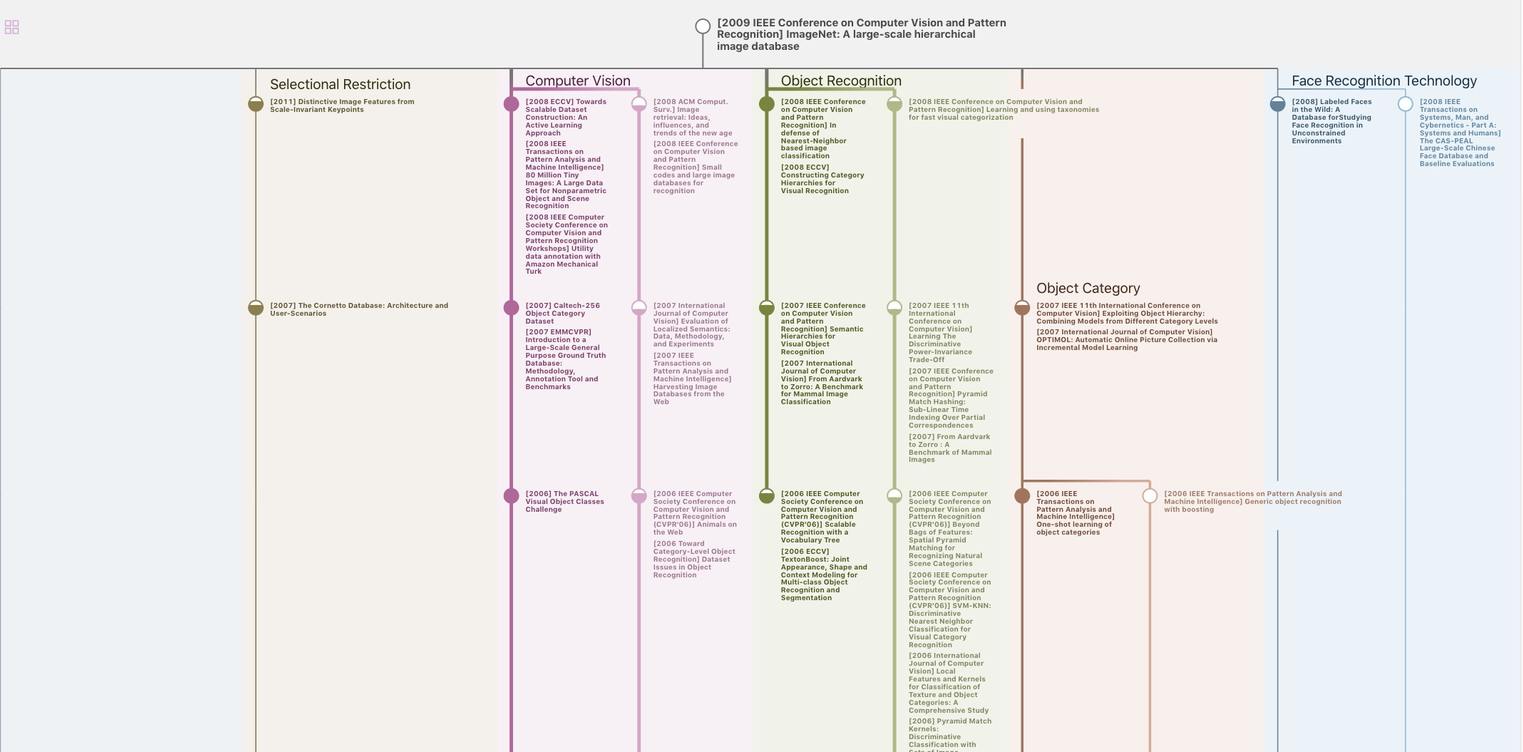
生成溯源树,研究论文发展脉络
Chat Paper
正在生成论文摘要