Transferability-Guided Multi-source Model Adaptation for Medical Image Segmentation
MEDICAL IMAGE COMPUTING AND COMPUTER ASSISTED INTERVENTION, MICCAI 2023, PT II(2023)
摘要
Unsupervised domain adaptation has drawn sustained attentions in medical image segmentation by transferring knowledge from labeled source data to unlabeled target domain. However, most existing approaches assume the source data are collected from a single client, which cannot be successfully applied to explore complementary transferable knowledge from multiple source domains with large distribution discrepancy. Moreover, they require access to source data during training, which is inefficient and unpractical due to privacy preservation and memory storage. To address these challenges, we study a novel and practical problem, named multi-source model adaptation (MSMA), which aims to transfer multiple source models to the unlabeled target domain without any source data. Since no target label and source data is provided to evaluate the transferability of each source model or domain gap between the source and the target domain, we may encounter negative transfer by those less related source domains, thus hurting target performance. To solve this problem, we propose a transferability-guided model adaptation (TGMA) framework to eliminate negative transfer. Specifically, 1) A label-free transferability metric (LFTM) is designed to evaluate transferability of source models without target annotations for the first time. 2) Based on the designed metric, we compute instance-level transferability matrix (ITM) for target pseudo label correction and domain-level transferability matrix (DTM) to achieve model selection for better target model initialization. Extensive experiments on multi-site prostate segmentation dataset demonstrate the superiority of our framework.
更多查看译文
关键词
Source-free Domain Adaptation,Multi-source Label-free transferability metric
AI 理解论文
溯源树
样例
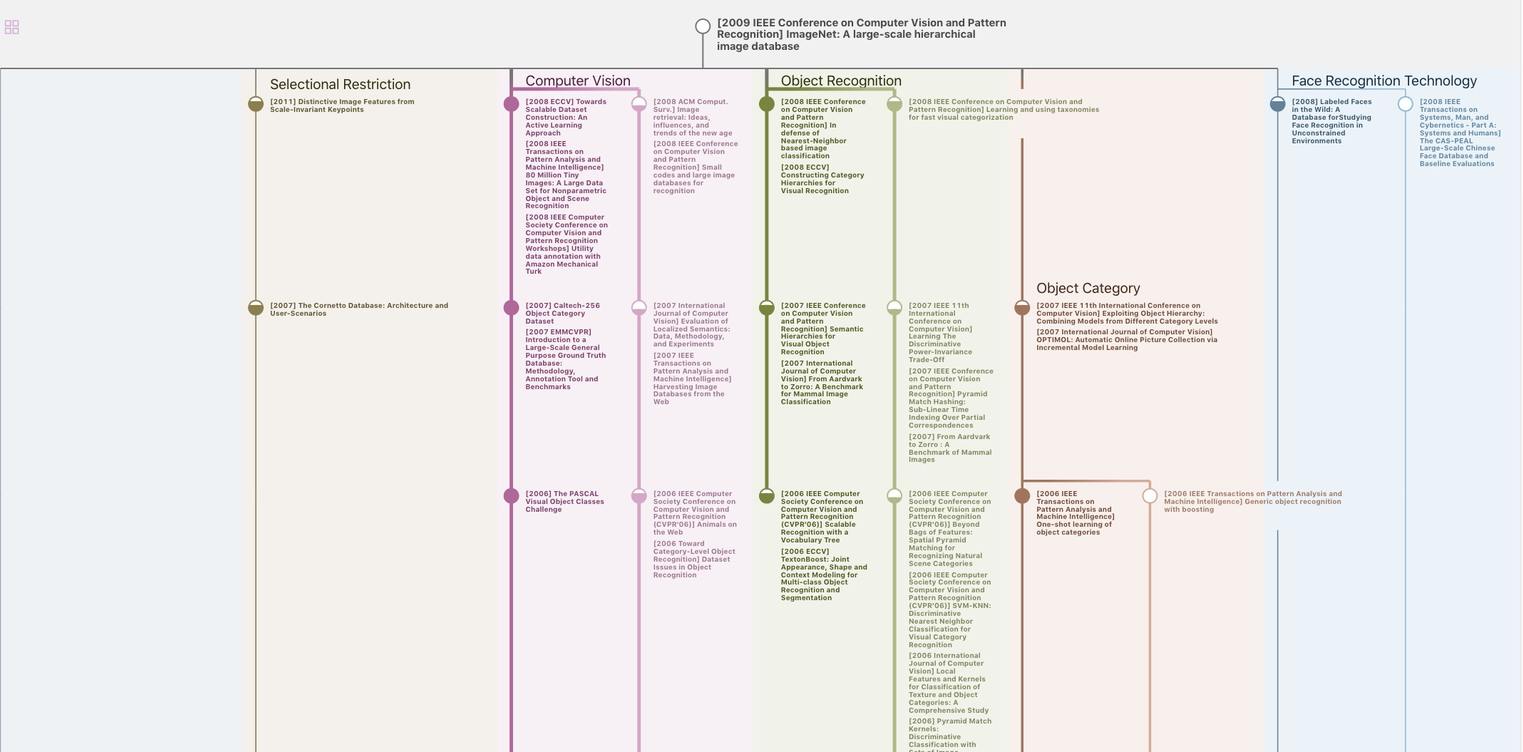
生成溯源树,研究论文发展脉络
Chat Paper
正在生成论文摘要