3D Dental Mesh Segmentation Using Semantics-Based Feature Learning with Graph-Transformer
MEDICAL IMAGE COMPUTING AND COMPUTER ASSISTED INTERVENTION, MICCAI 2023, PT VII(2023)
摘要
Accurate segmentation of digital 3D dental mesh plays a crucial role in various specialized applications within oral medicine. While certain deep learning-based methods have been explored for dental mesh segmentation, the current quality of segmentation fails to meet clinical requirements. This limitation can be attributed to the complexity of tooth morphology and the ambiguity of gingival line. Further more, the semantic information of mesh cells which can provide valuable insights into their categories and enhance local geometric attributes is usually disregarded. Therefore, the segmentation of dental mesh presents a significant challenge in digital oral medicine. To better handle the issue, we propose a novel semantics-based feature learning for dental mesh segmentation that can fully leverage the semantic information to grasp the local and non-local dependencies more accurately through a well-designed graph-transformer. Moreover, we perform adaptive feature aggregation of cross-domain features to obtain high-quality cell-wise 3D dental mesh segmentation results. We validate our method using real 3D dental mesh, and the results demonstrate that our method outperforms the state-of-the-art one-stage methods on 3D dental mesh segmentation. Our Codes are available at https://github.com/df-boy/SGTNet.
更多查看译文
关键词
Dental mesh segmentation,Graph-Transformer,Adaptive feature aggregation
AI 理解论文
溯源树
样例
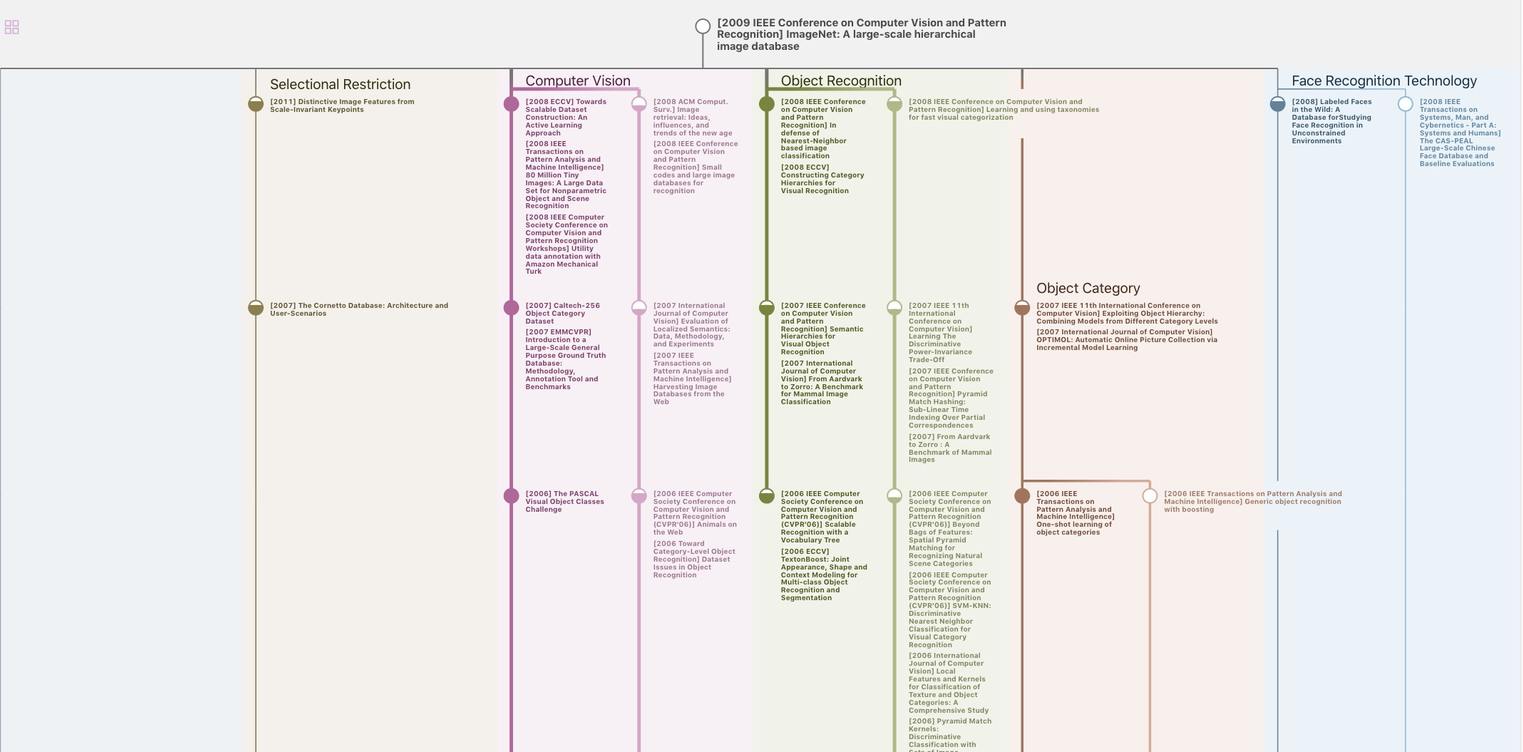
生成溯源树,研究论文发展脉络
Chat Paper
正在生成论文摘要