Deep Unsupervised Clustering for Conditional Identification of Subgroups Within a Digital Pathology Image Set
MEDICAL IMAGE COMPUTING AND COMPUTER ASSISTED INTERVENTION, MICCAI 2023, PT VIII(2023)
摘要
Consideration of subgroups or domains within medical image datasets is crucial for the development and evaluation of robust and generalizable machine learning systems. To tackle the domain identification problem, we examine deep unsupervised generative clustering approaches for representation learning and clustering. The Variational Deep Embedding (VaDE) model is trained to learn lower-dimensional representations of images based on a Mixture-of-Gaussians latent space prior distribution while optimizing cluster assignments. We propose the Conditionally Decoded Variational Deep Embedding (CDVaDE) model which incorporates additional variables of choice, such as the class labels, as conditioning factors to guide the clustering towards subgroup structures in the data which have not been known or recognized previously. We analyze the behavior of CDVaDE on multiple datasets and compare it to other deep clustering algorithms. Our experimental results demonstrate that the considered models are capable of separating digital pathology images into meaningful subgroups. We provide a general-purpose implementation of all considered deep clustering methods as part of the open source Python package DomId (https://github.com/DIDSR/DomId).
更多查看译文
关键词
Domain Identification,Deep Clustering,Subgroup Identification,Variational Autoencoder,Generative Model
AI 理解论文
溯源树
样例
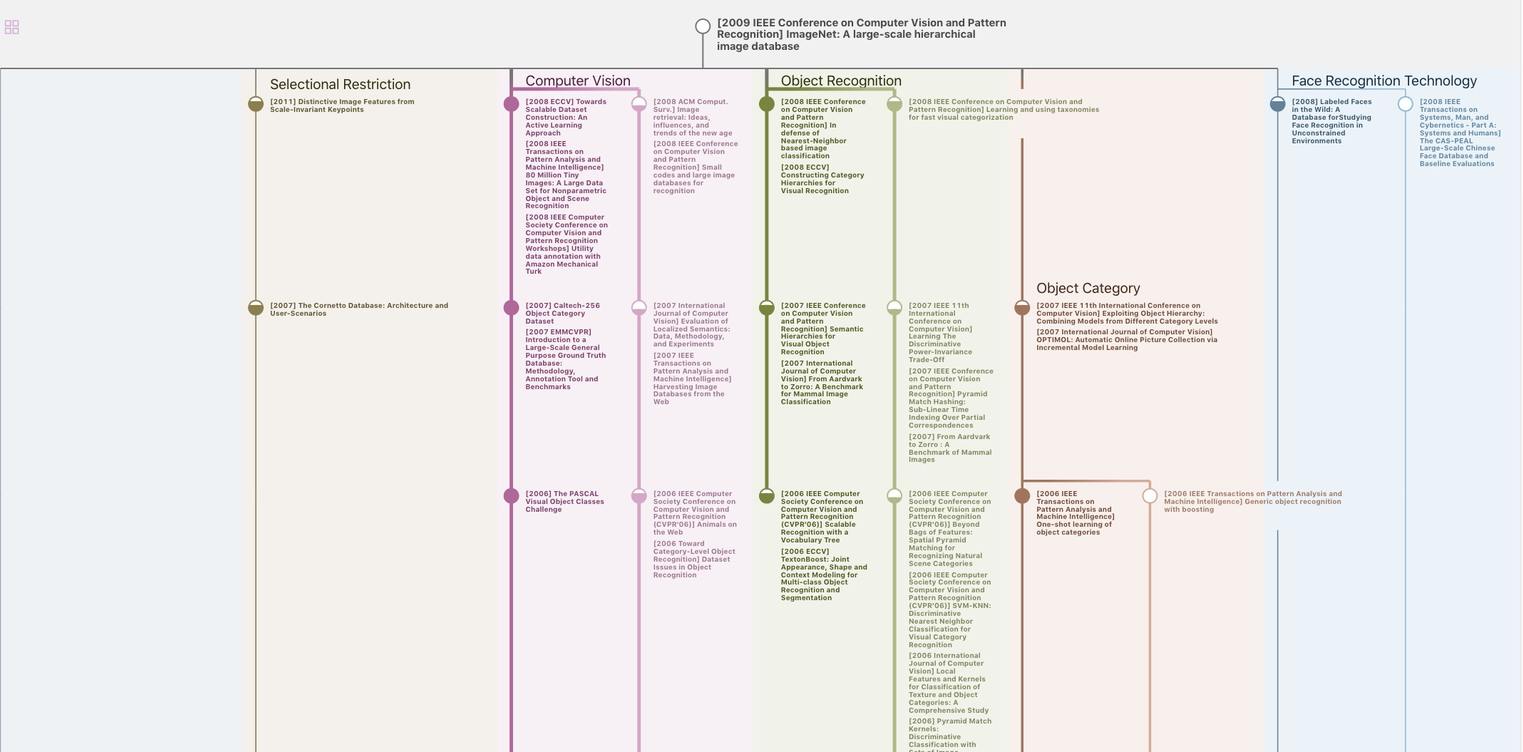
生成溯源树,研究论文发展脉络
Chat Paper
正在生成论文摘要