Automated Segmentation of Nasopharyngeal Carcinoma Based on Dual-Sequence Magnetic Resonance Imaging Using Self-supervised Learning
COMPUTATIONAL MATHEMATICS MODELING IN CANCER ANALYSIS, CMMCA 2023(2023)
摘要
Radiation therapy stands as a principal treatment for nasopharyngeal carcinoma (NPC). The contouring of tumor regions for radiotherapy planning is traditionally done manually by oncologists, a process that is time-consuming and subject to individual subjectivity. Although fully automated deep-learning models could offer a solution, their segmentation performance is often hindered by the lack of abundant annotated data. We retrospectively analyzed the image data of 116 NPC patients, with lesions from 44 patients labeled and those from the remaining 72 left unlabeled. We proposed a fully automated method leveraging self-supervised learning to overcome the issue of scarce data annotations, aiming to achieve precise segmentation of NPC lesions from dual-sequence magnetic resonance images. The mean Dice Similarity Coefficient in a ten-fold cross-validation was 0.75, comparable to results using 4100 annotated cases for training, while only utilizing 44 annotated cases in our study. Examination of the results demonstrated that our method requires minimal modification, less than 20% for the Dice score primarily, to fulfill clinical application requirements. We proposed a fully automatic segmentation method for NPC, showcasing both accuracy and stability. This approach, constructed with a minimal amount of labeled data and enhanced with unlabeled data, can significantly contribute to the automated segmentation of tumor lesions, easing the annotation burden on oncologists, diminishing subjectivity, and increasing the reliability of lesion delineation.
更多查看译文
关键词
Nasopharyngeal carcinoma,deep learning,self-supervised learning,automatic segmentation
AI 理解论文
溯源树
样例
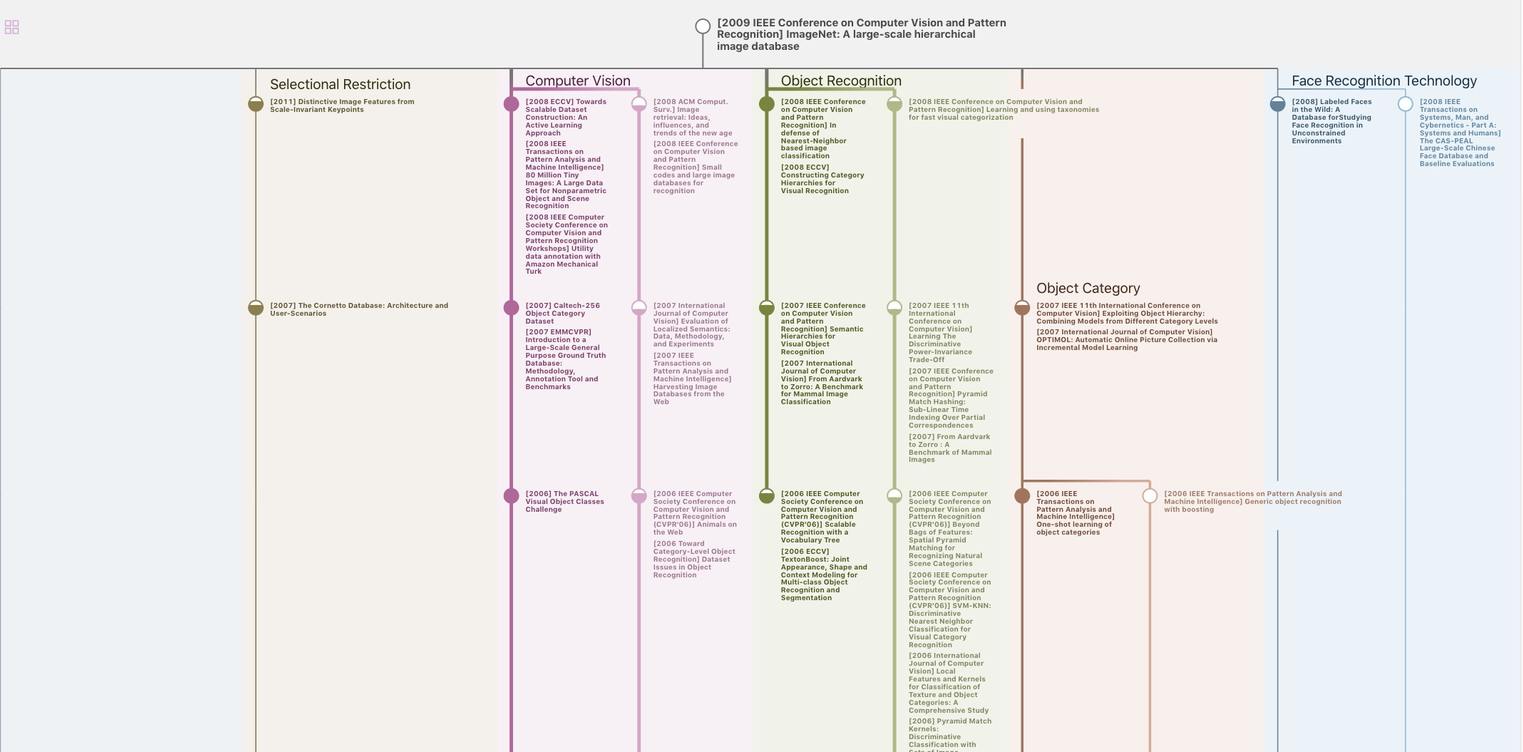
生成溯源树,研究论文发展脉络
Chat Paper
正在生成论文摘要