Masked Image Modeling for Label-Efficient Segmentation in Two-Photon Excitation Microscopy.
MILLanD@MICCAI(2023)
摘要
Generating accurate structural segmentation for 3D two-photon excitation microscopy (TPEM) images affords insights into how cellular-scale networks in living animal models respond to disease. Manual creation of dense segmentation masks is, however, very time-consuming image modeling (MIM) has recently emerged as a highly effective self-supervised learning (SSL) formulation for feature extraction in natural images, reducing reliance on human-created labels. Here, we extend MIM to 3D TPEM datasets and show that a model pretrained using MIM obtains improved downstream segmentation performance relative to random initialization. We assessed our novel pipeline using multi-channel TPEM data on two common segmentation tasks, neuronal and vascular segmentation. We also introduce intensity-based and channel-separated masking strategies that respectively aim to exploit the intra-channel correlation of intensity and foreground structures, and inter-channel correlations that are specific to microscopy images. We show that these methods are effective for generating representations of TPEM images, and identify novel insights on how MIM can be modified to yield more salient image representations for microscopy. Our method reaches statistically similar performances to a fully-supervised model (using the entire dataset) when only requiring just 25% of the labeled data for both neuronal and vascular segmentation tasks. To the best of our knowledge, this is the first investigation applying MIM methods to microscopy, and we hope our presented SSL pipeline may both reduce the necessary labeling effort and improve downstream analysis of TPEM images for neuroscience investigations. To this end, we plan to make the SSL pipeline, pretrained models and training code available under the following GitHub organization: https://github.com/AICONSlab .
更多查看译文
关键词
microscopy,segmentation,image modeling,label-efficient,two-photon
AI 理解论文
溯源树
样例
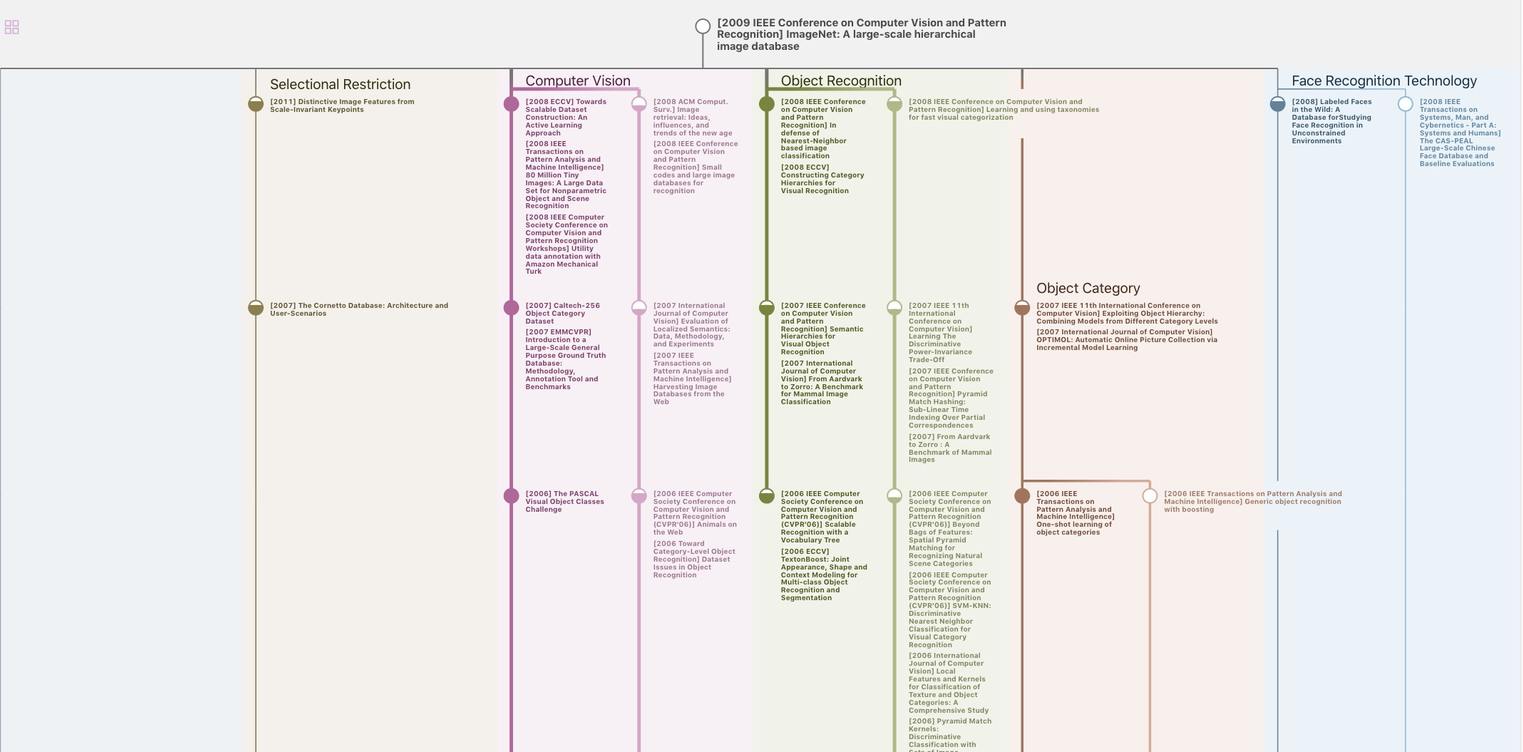
生成溯源树,研究论文发展脉络
Chat Paper
正在生成论文摘要