PASSNet: A Spatial-Spectral Feature Extraction Network With Patch Attention Module for Hyperspectral Image Classification.
IEEE Geosci. Remote. Sens. Lett.(2023)
Abstract
Convolutional neural networks (CNNs) have achieved success in hyperspectral image (HSI) classification, but the performance is constrained by the limited reception field. In this regard, vision transformer (ViT) is introduced recently, which is of powerful capabilities in long-range feature extraction for HSI classification. However, transformers are computation intensive and poor for local feature extraction. The motivation for this study is to build a lightweight hybrid model, which ensembles the respective inductive bias from CNNs and global receptive field from transformers. In this work, we propose a concise and efficient framework—the spatial–spectral feature extraction network with patch attention module (PAM) (PASSNet), to simultaneously extract both local and global features. Specifically, we design an innovative plugin called PAM, which can be easily integrated into both CNNs and transformers blocks to extract spatial–spectral features from multiple spatial perspectives. Besides, a novel partial convolution (PConv) operation is introduced, with a reduced computational cost than vanilla convolution operation. Through coupling the local attention from the CNNs with the global receptive fields in the transformers, the proposed PASSNet exhibits a superior classification performance on three well-known datasets with a small training sample size.
MoreTranslated text
Key words
hyperspectral image classification,patch attention module,spatial-spectral
AI Read Science
Must-Reading Tree
Example
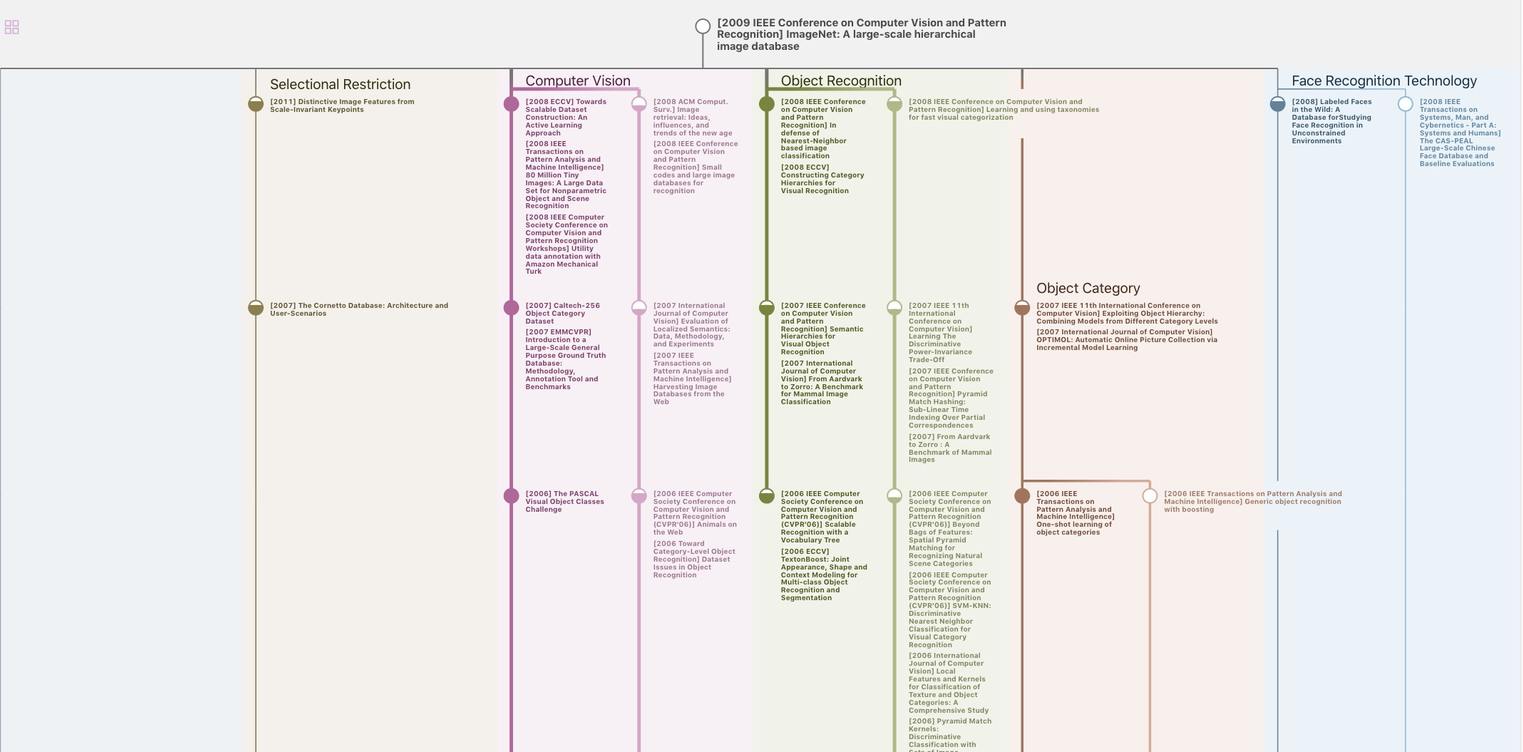
Generate MRT to find the research sequence of this paper
Chat Paper
Summary is being generated by the instructions you defined