Maximum-Entropy Satisficing Linear Quadratic Regulator
IEEE Control. Syst. Lett.(2023)
摘要
This letter formalizes a constrained maximum-entropy Linear Quadratic Regulator (LQR) problem and provides a computationally-efficient solution for it. The traditional LQR cost is penalized with an additional entropy cost, and a cumulative safety constraint is also imposed. This formulation will not only promote randomness to achieve exploration or unpredictability but also ensure that the performance is deemed satisfactory despite randomness. A stochastic controller is designed, and it is first shown that the resulting optimization problem for finding the control gain and covariance is non-convex. By employing Lagrange duality formalism, the non-convex optimization problem is then transformed into a convex one. More specifically, the constrained entropy-regularized LQR problem is reformulated as a set of linear matrix inequalities (LMIs), facilitating the determination of the control gain and covariance. A simulation example is provided to verify the effectiveness of the presented results.
更多查看译文
关键词
Entropy,Costs,Safety,Cost function,Regulators,Optimal control,Covariance matrices,Maximum-entropy satisficing linear quadratic regulator (MESLQR),probabilistic control,semi definite programming (SDP)
AI 理解论文
溯源树
样例
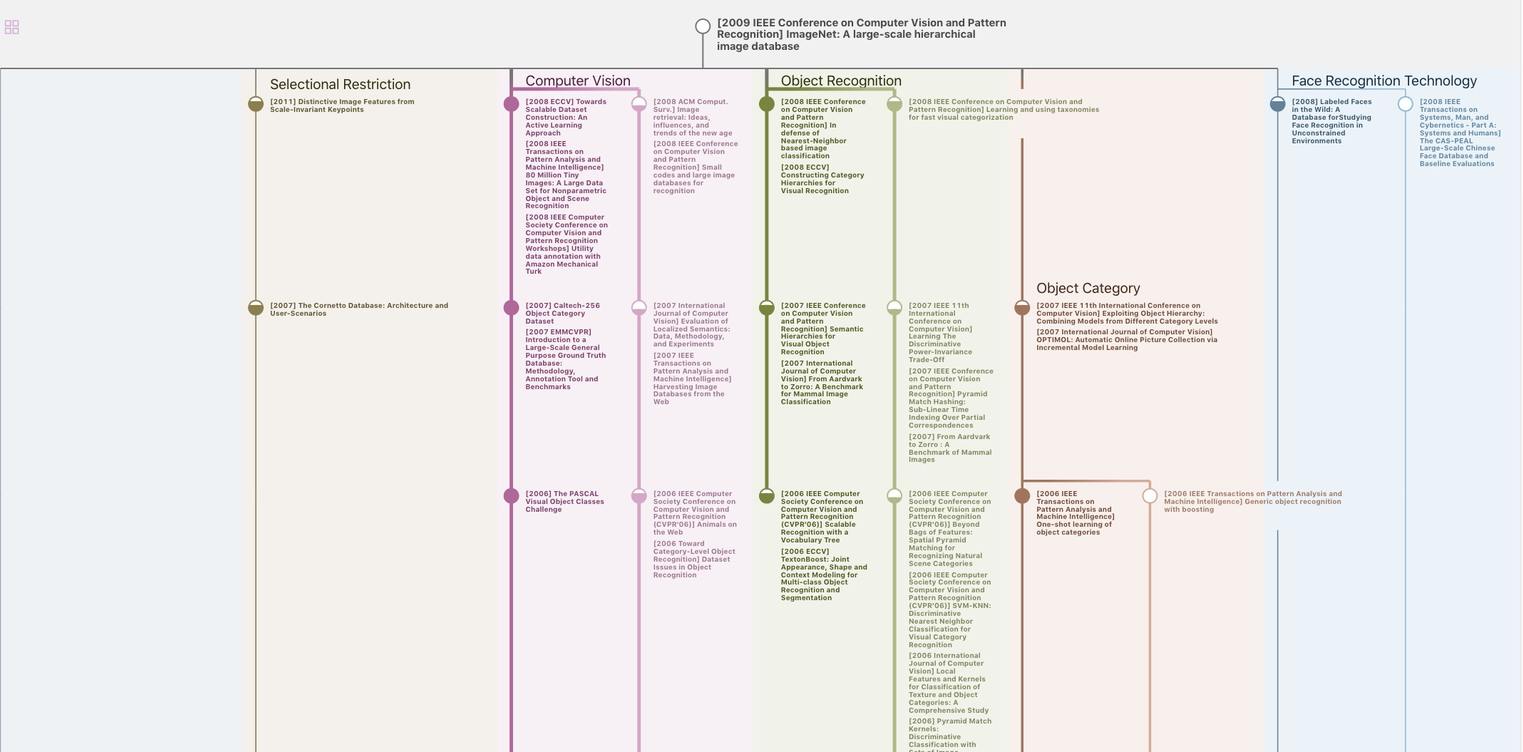
生成溯源树,研究论文发展脉络
Chat Paper
正在生成论文摘要