Adversarial Attacks on Deep Learning-Based DOA Estimation With Covariance Input.
IEEE Signal Process. Lett.(2023)
摘要
Although deep learning methods have made significant advancements across various domains, recent research has shown that carefully crafted adversarial samples can lead to a significant degradation in the performance of deep learning models. Such adversarial examples raise concerns about the reliability and safety of deep learning-based models. Currently, there is a lack of research on the robustness of deep learning-based DOA methods against adversarial samples. This letter aims to fill this research gap by leveraging the differentiability of the transformation process from the original signal to the covariance matrix. By utilizing this differentiability, the robustness of the DOA estimation model, which takes the covariance matrix as input, is investigated. Four different white-box attack methods are considered to generate adversarial samples to evaluate the resilience of the model. The experimental results demonstrate that all four methods employed significantly increase the estimation error of the DOA estimation model, posing a serious threat to the model's security.
更多查看译文
关键词
doa estimation,covariance input,learning-based
AI 理解论文
溯源树
样例
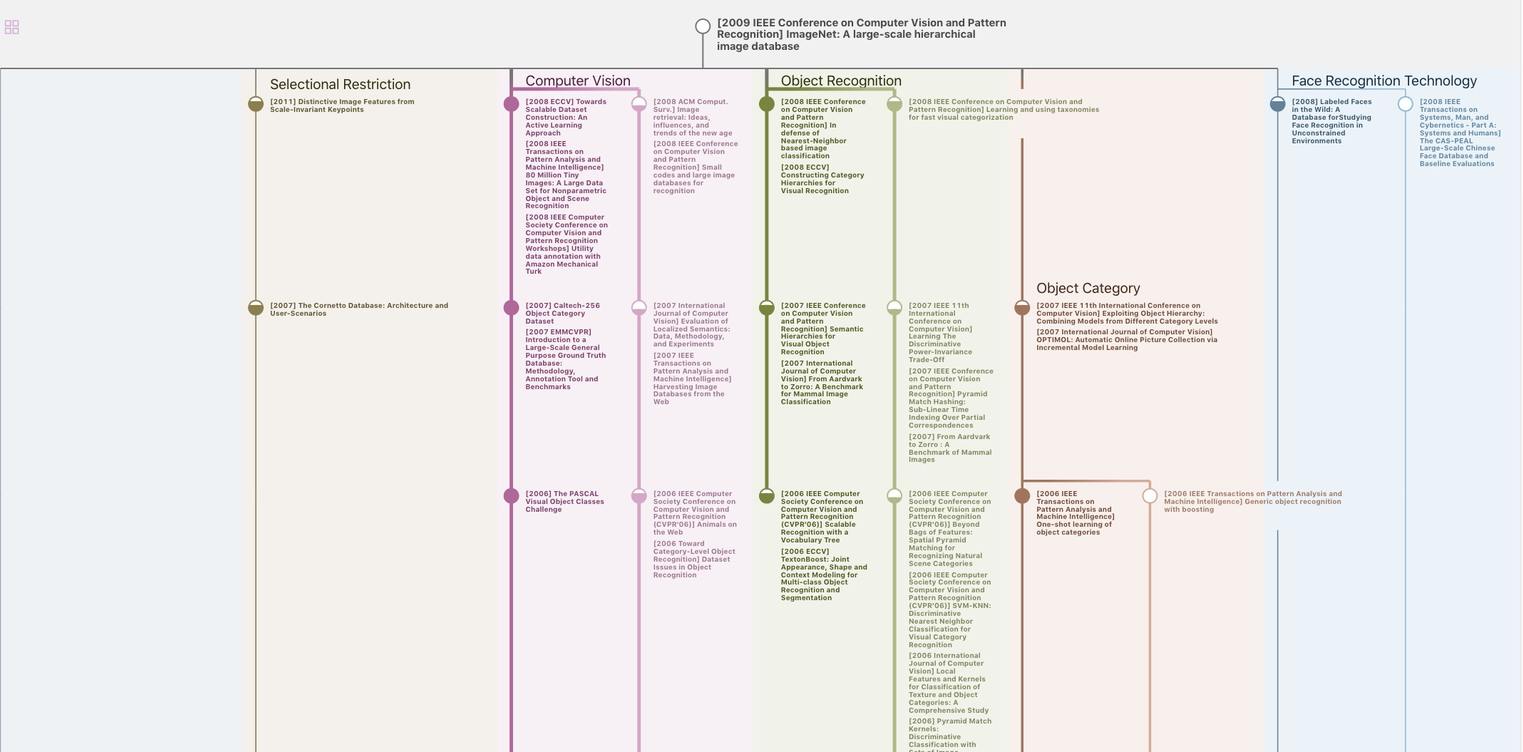
生成溯源树,研究论文发展脉络
Chat Paper
正在生成论文摘要