SIDA: Self-Supervised Imbalanced Domain Adaptation for Sound Enhancement and Cross-Domain WiFi Sensing.
Proc. ACM Interact. Mob. Wearable Ubiquitous Technol.(2023)
摘要
The coronavirus disease 2019 (COVID-19) pneumonia still persists and its chief complaint is dry cough. Physicians design wireless stethoscopes to facilitate diagnosis, however, lung sounds could be easily interfered with by external noises. To achieve lung sound enhancement, prior researches mostly assume the amount of clean and noisy data are the same. This assumption is hardly met due to extensive labor effort for data collection and annotation. The data imbalance across domains widely happens in real-world IoT systems, e.g. sound enhancement and WiFi-based human sensing. In this paper, we propose SIDA, a self-supervised imbalanced domain adaptation framework for sound enhancement and WiFi sensing, which makes it a generic time series domain adaptation solution for IoT systems. SIDA proposes a self-supervised imbalanced domain adaptation model that separately learns the representation of time series signals in a minority domain with limited samples, a majority domain with rich samples, and their mapping relations. For lung sound enhancement, we further proposes a phase correction model to sanitize the phase and a SNR prediction algorithm to recursively perform domain adaptation in an imbalanced noisy and clean lung sound dataset. Extensive experiments demonstrate SIDA increases noisy samples' SNR by 16.49dB and 4.06dB on a synthetic and a realistic imbalanced lung sound dataset, respectively. For WiFi-based human sensing, SIDA designs a cross-domain WiFi-based human identification model irrespective of walking trajectory. A specific trajectory where a group of people walks along in a realistic testing environment is considered the minority domain, and several other trajectories are stored at a server as the majority domain. Extensive experiments show SIDA could recognize individuals with an average accuracy of 94.72% and significantly outperform baselines on highly imbalanced WiFi dataset in cross-domain human identification tasks.
更多查看译文
关键词
Sound sensing, WiFi sensing, IoT, Domain imbalance, Domain adaptation, ICBHI dataset
AI 理解论文
溯源树
样例
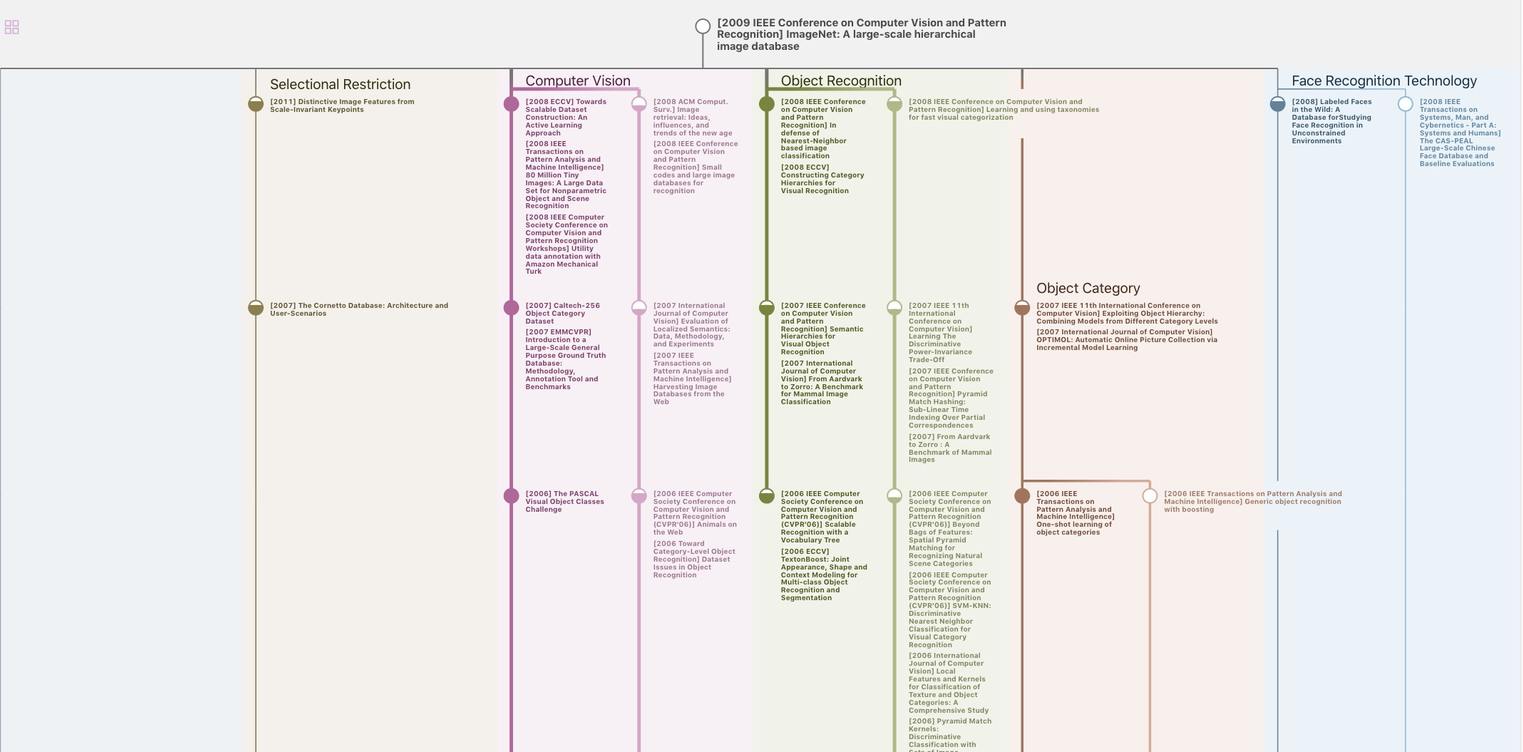
生成溯源树,研究论文发展脉络
Chat Paper
正在生成论文摘要