CrowdQ: Predicting the Queue State of Hospital Emergency Department Using Crowdsensing Mobility Data-Driven Models.
Proc. ACM Interact. Mob. Wearable Ubiquitous Technol.(2023)
摘要
Hospital Emergency Departments (EDs) are essential for providing emergency medical services, yet often overwhelmed due to increasing healthcare demand. Current methods for monitoring ED queue states, such as manual monitoring, video surveillance, and front-desk registration are inefficient, invasive, and delayed to provide real-time updates. To address these challenges, this paper proposes a novel framework, CrowdQ, which harnesses spatiotemporal crowdsensing data for real-time ED demand sensing, queue state modeling, and prediction. By utilizing vehicle trajectory and urban geographic environment data, CrowdQ can accurately estimate emergency visits from noisy traffic flows. Furthermore, it employs queueing theory to model the complex emergency service process with medical service data, effectively considering spatiotemporal dependencies and event context impact on ED queue states. Experiments conducted on large-scale crowdsensing urban traffic datasets and hospital information system datasets from Xiamen City demonstrate the framework's effectiveness. It achieves an F1 score of 0.93 in ED demand identification, effectively models the ED queue state of key hospitals, and reduces the error in queue state prediction by 18.5%-71.3% compared to baseline methods. CrowdQ, therefore, offers valuable alternatives for public emergency treatment information disclosure and maximized medical resource allocation.
更多查看译文
关键词
Mobile trajectory mining, Hospital queue state modeling, Spatiotemporal crowdsensing data, Urban computing
AI 理解论文
溯源树
样例
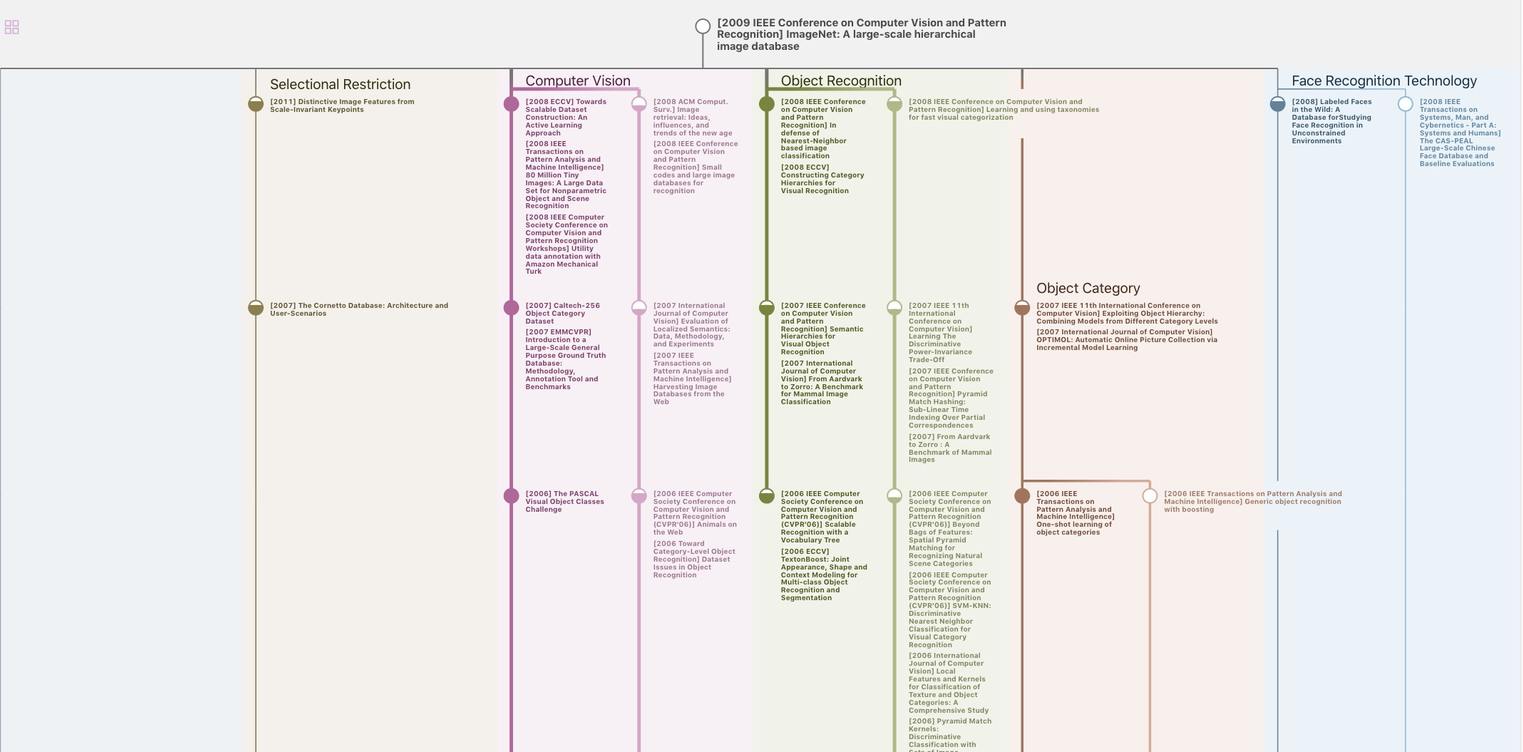
生成溯源树,研究论文发展脉络
Chat Paper
正在生成论文摘要