Wind Turbine Blade Breakage Monitoring With Mogrifier LSTM Autoencoder.
IEEE Trans. Instrum. Meas.(2023)
Abstract
Wind turbines (WTs) often work in harsh environmental conditions. The risk of blade breakage by hitting the tower has increased because of the decreased bending stiffness of the WT blades with the increasing sizes of WTs. To address the system dynamics of WTs, this article proposes a Mogrifier long short-term memory autoencoder (MLSTM-AE) method to monitor blade breakage using the supervisory control and data acquisition (SCADA) data. Firstly, the Pearson correlation coefficient (PCC) is calculated for variable selection. Secondly, the time-lagged multivariate variables are augmented and taken as input for the encoder which consists of a Mogrifier long short-term memory (MLSTM) layer to learn the deep features. Another MLSTM layer runs as the decoder to reconstruct the input. The proposed MLSTM-AE model can extract spatial-temporal information more effectively than traditional long short-term memory (LSTM) and autoencoder (AE). Next, kernel density estimation (KDE) is applied to develop boundaries for generating blade breakage alerts based on reconstruction errors, which can reflect changes in system dynamics caused by blade breakage. The advantages of the proposed MLSTM-AE-based monitoring method are illustrated by employing real blade breakage cases from several wind farms located in China by comparing with other related methods in terms of warning time, false alarm rate (FAR), and accuracy.
MoreTranslated text
Key words
Blade breakage monitoring, long short-term memory (LSTM), mogrifier long short-term memory autoencoder (MLSTM-AE), supervisory control and data acquisition (SCADA) data, wind turbine (WT)
AI Read Science
Must-Reading Tree
Example
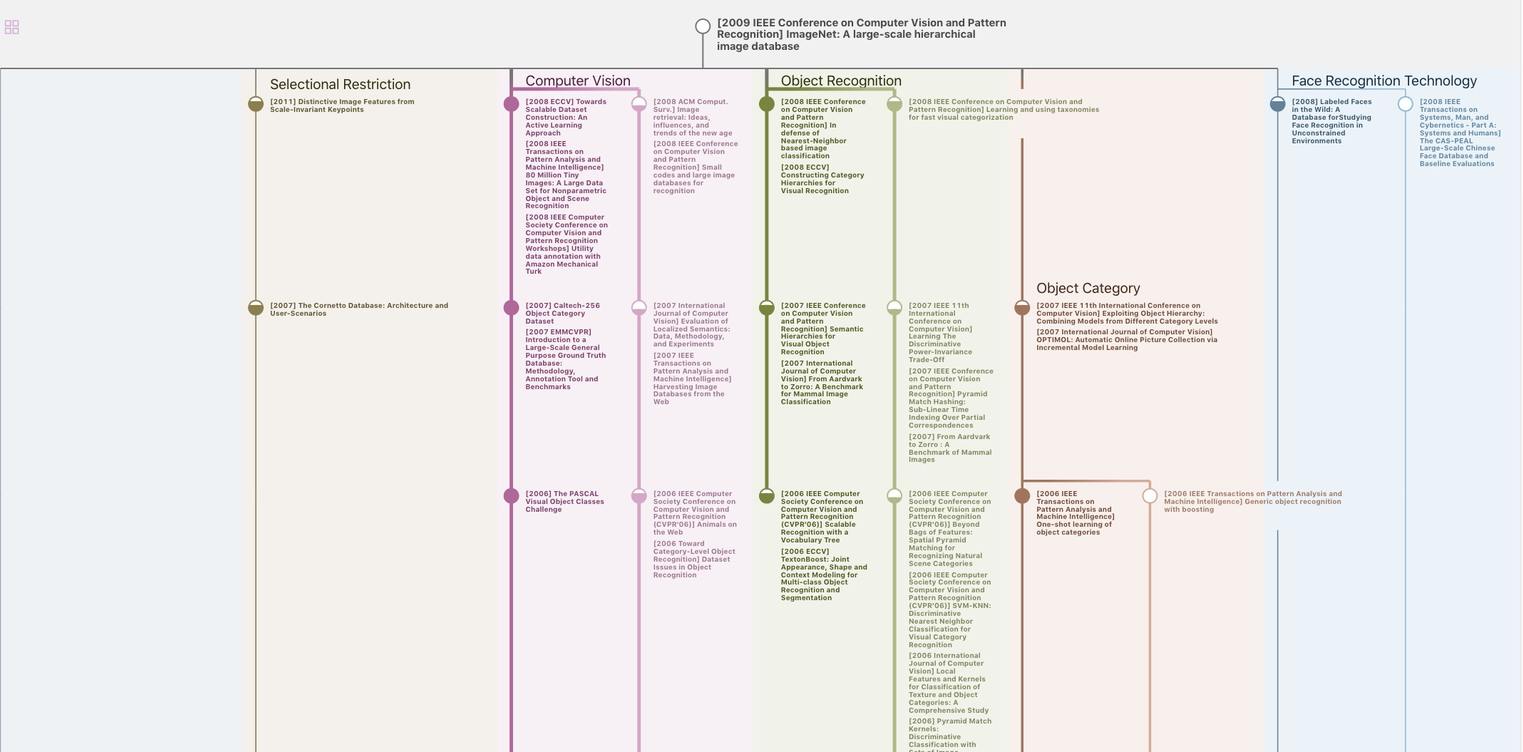
Generate MRT to find the research sequence of this paper
Chat Paper
Summary is being generated by the instructions you defined