Spatiotemporal Generative Adversarial Imputation Networks: An Approach to Address Missing Data for Wind Turbines.
IEEE Trans. Instrum. Meas.(2023)
Abstract
Wind turbine data collection often suffers from missing data due to network blockage and sensor failure. Existing data imputation methods require complete datasets for training and are limited to individual turbines, resulting in time-consuming dataset preparation and a lack of generalization. To address these limitations, spatiotemporal generative adversarial imputation networks (STGAINs) are proposed for data imputation. First, generative adversarial networks (GANs) containing a hint-mask fusion mechanism are presented, enabling data imputation without complete datasets. Second, a spatiotemporal module is proposed to capture the historical decay and feature correlation of wind turbine data to obtain potential trends. Third, an online update mechanism based on decision error is proposed to adapt the model parameters to varying environmental conditions. STGAIN overcomes geographic constraints, imputes data reflecting change patterns, avoids extensive sample collection, and enables online adjustments. Finally, experiments with real wind turbine data demonstrate that the proposed method produces imputed data conforming to the original distribution while considering spatiotemporal correlation. Evaluating the metrics results reveals that STGAIN significantly outperforms comparison methods, improving data imputation performance by at least 6%, demonstrating its significant benefits.
MoreTranslated text
Key words
Data imputation,generative adversarial networks (GANs),online update,spatial–temporal,wind turbine
AI Read Science
Must-Reading Tree
Example
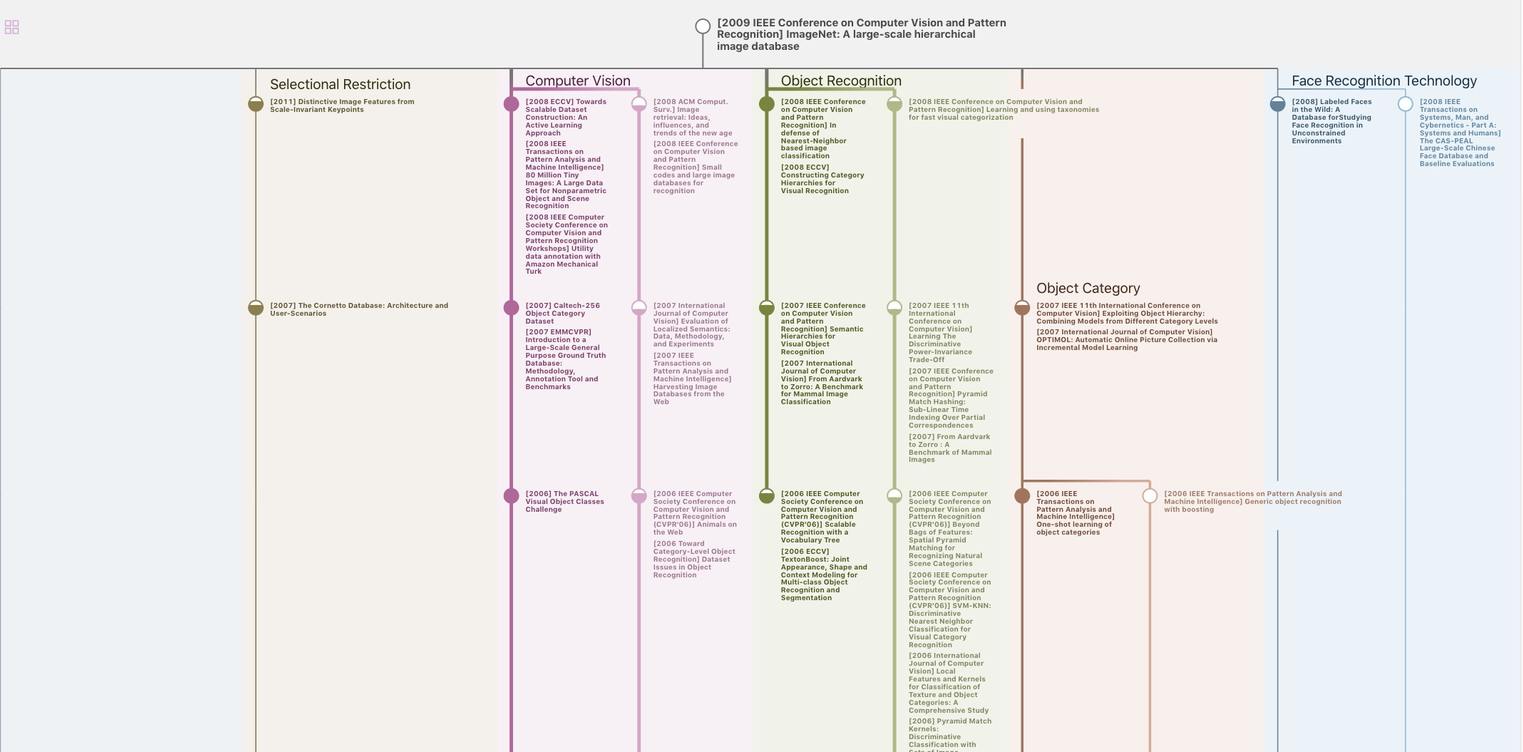
Generate MRT to find the research sequence of this paper
Chat Paper
Summary is being generated by the instructions you defined