BFRNet: Bidimensional Feature Representation Network for Remote Sensing Images Classification
IEEE TRANSACTIONS ON GEOSCIENCE AND REMOTE SENSING(2023)
摘要
In recent years, convolutional neural network (CNN) and transformer, as mainstream classification methods, have made good progress in improving the classification performance of remote sensing (RS) images. Furthermore, the CNN-transformer hybrid architecture has shown a greater potential for enabling models to obtain local information and global dependency relationships. In general, numerous researches improve self-attention block of vision transformer (ViT) in the spatial dimension. Nevertheless, spatial self-attention mostly achieves a single spatial feature extraction, which cannot meet the requirement of accurate recognition of high-resolution RS images. In this work, a method for representing spatial and channel dimensions of RS images is proposed, which not only extracts global-local spatial features but also pays special attention to incorporate channel information. Specifically, bidimensional local window self-attention (BLWS) and pyramid pool self-attention are conducted to extract local-global features. Subsequently, a linear attention module will fuse local-global information in the channel dimension when computing multihead self-attention (MHSA). A bidimensional gating unit (BGU) is used to replace the traditional multilayer perceptron (MLP) of the feedforward network (FFN). The above improvements result in a bidimensional feature representation (BFR) block, and BFR network (BFRNet) is designed based on BFR blocks. BFRNet consists of four stages, and each stage repeatedly stacks BFR blocks with different layers. Experiments show that the classification accuracy of BFRNet is significantly better than the existing methods of CNN, ViTs, and CNN-transformer networks. On dataset RSSCN7, BFRNet achieves a classification accuracy of 98.75% with only 1.9G floating point operations (FLOPs), which is 8.21% higher than ViT, 3.21% higher than Resnet50, and 2.68% higher than CoAtNet, respectively.
更多查看译文
关键词
Channel attention,convolutional neural network (CNN),image classification,remote sensing (RS),spatial attention,transformer
AI 理解论文
溯源树
样例
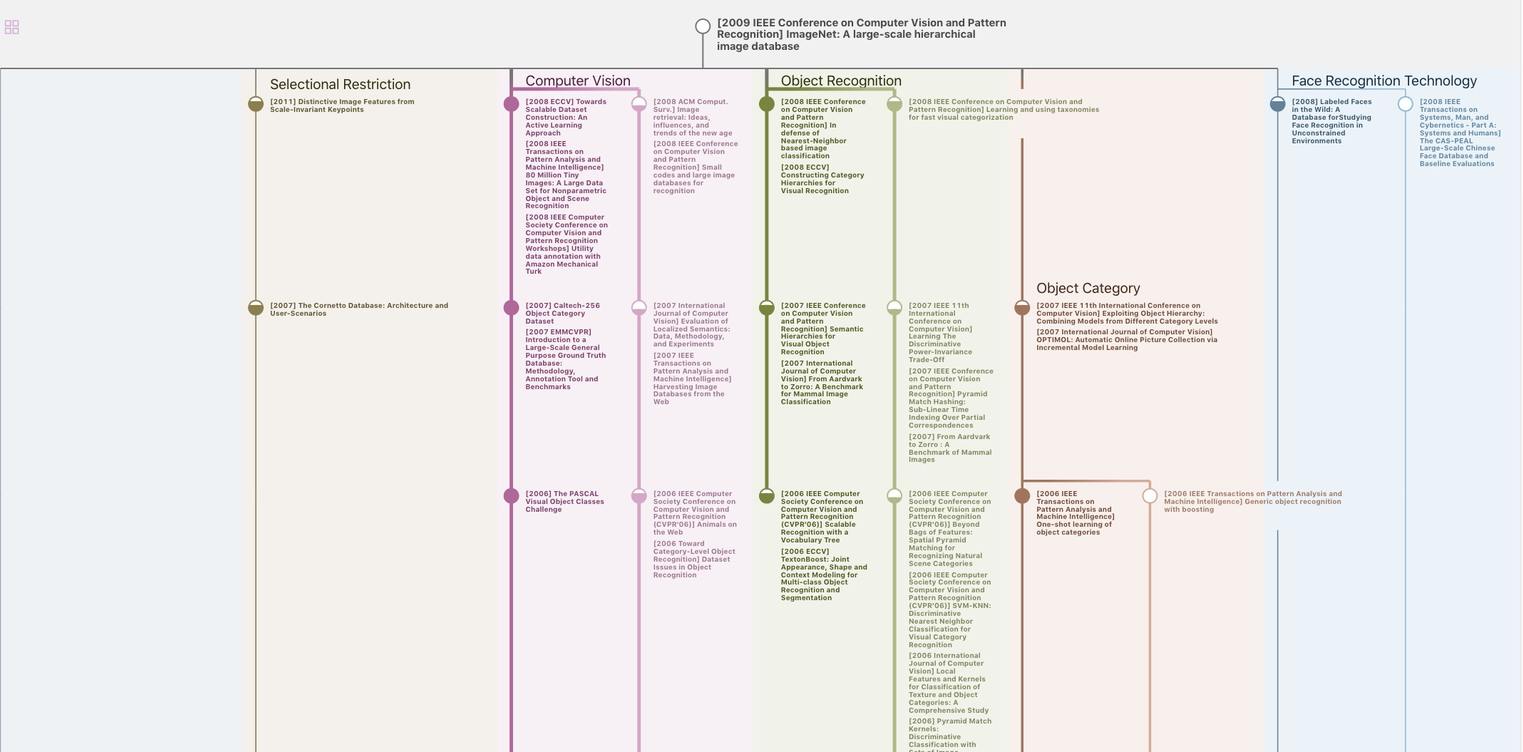
生成溯源树,研究论文发展脉络
Chat Paper
正在生成论文摘要