RATIR-Net: Adaptive SAR Image Reconstruction Based on Transformer Architecture.
IEEE Trans. Geosci. Remote. Sens.(2023)
摘要
Despite its widespread use in Earth remote sensing, synthetic aperture radar (SAR) image reconstruction remains challenging. The difficulties mainly lie in the handling of diverse scenes and motion errors with sparsely sampled data. Existing matched filter (MF)-based methods cannot handle sparsely sampled data, while regularization-based methods lack adaptability to scene diversity. Although deep learning-based SAR methods can deal with these two issues, their performance will be degraded by motion errors. To address this, we propose a Transformer-based SAR image reconstruction method called residual attention transformer image reconstruction network (RATIR-Net). The proposed method can obtain SAR images of various scenes under sparse sampling and motion errors by learning the correlations between echo data. In RATIR-Net, convolutional neural network (CNN)-based encoding and decoding blocks are constructed to implement azimuth processes of range profiles (RPs) in the range-Doppler (RD) domain according to the MF-based method. Meanwhile, a residual attention transformer (RAT) block is designed to extract correlations between RPs, compensating for information loss caused by sparse sampling and suppressing noncorrelated perturbations caused by motion errors. The CNN-based encoding and decoding blocks help reduce computing costs, and the RAT block mitigates the dependence on scene features and the influence of motion errors. These make RATIR-Net efficient and effective. Simulation experiments have been conducted to verify the proposed method.
更多查看译文
关键词
adaptive sar image reconstruction,transformer,ratir-net
AI 理解论文
溯源树
样例
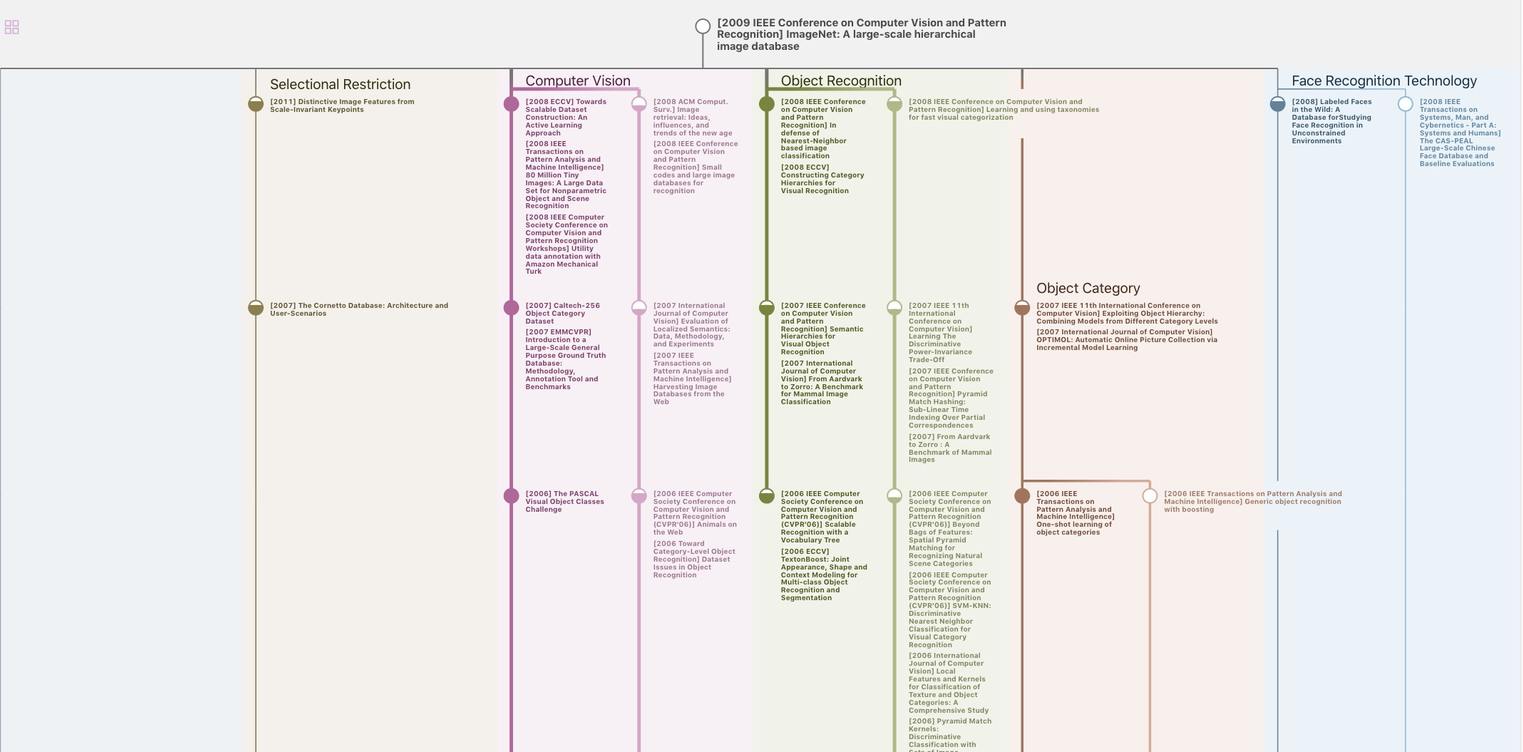
生成溯源树,研究论文发展脉络
Chat Paper
正在生成论文摘要