Shadow Mask-Driven Multimodal Intrinsic Image Decomposition for Hyperspectral and LiDAR Data Fusion.
IEEE Trans. Geosci. Remote. Sens.(2023)
摘要
Remote sensing (RS) modalities from multisensor platforms, including hyperspectral (HS) and light detection and ranging (LiDAR) data, have been garnering increasing attention in overcoming the lack of information diversity. However, the specific modality correlation is always weakened and neglected. By reducing the spectral uncertainty, HS intrinsic image decomposition (HIID) has been proven to be effective in enhancing the HS image quality. It is an ill-posed problem and is challenged to capture the inherent modality connection. This article proposes a shadow mask-driven multimodal intrinsic image decomposition (smMIID) for HS and LiDAR data fusion. Notably, smMIID creatively clarifies and activates the potential of the crossmodal information in RS modalities and builds on the strength of the LiDAR elevation information when constructing HIID constraints. Our motivation is to overcome the deficiency of information diversity and modality correlation in existing intrinsic image decomposition (IID)-based frameworks for better data fusion performance. Classic IID methods decompose the HS image into one reflectance component (RC) and one shading component (SC). There are three constraints in smMIID: 1) the fundamental constraint on RC and SC; 2) the HS-LiDAR hybrid gradient-based constraint on RC; and 3) the LiDAR gradient-based constraint on SC. A shadow mask is obtained and utilized to guarantee spectral consistency when combining neighboring samples. Experiments on HS and LiDAR datasets prove that smMIID outperforms other techniques in terms of visualization and classification. The analyses are provided theoretically and experimentally to demonstrate that smMIID is robust to shadow mask generation.
更多查看译文
关键词
hyperspectral,fusion
AI 理解论文
溯源树
样例
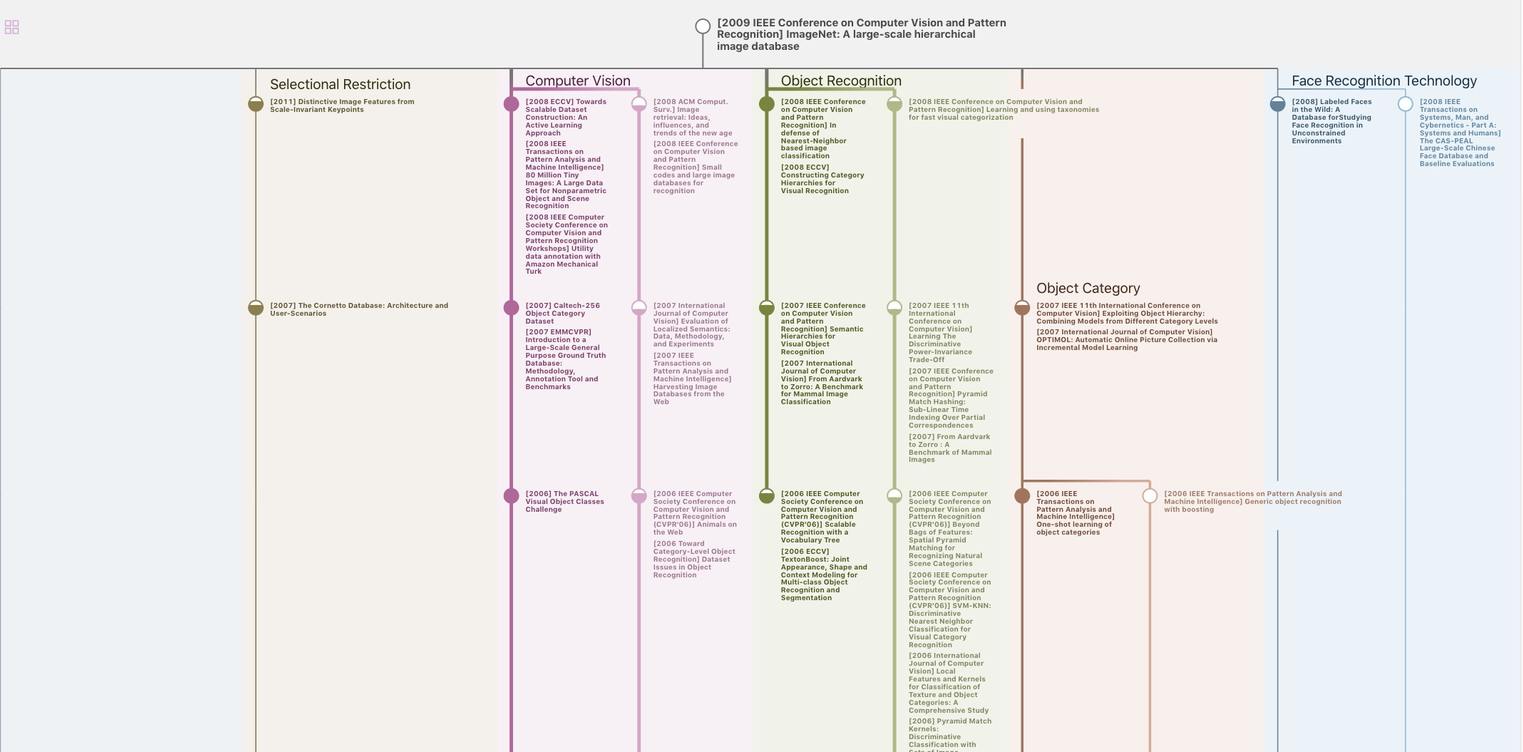
生成溯源树,研究论文发展脉络
Chat Paper
正在生成论文摘要