SAR Despeckling Using Multiobjective Neural Network Trained With Generic Statistical Samples
IEEE Trans. Geosci. Remote. Sens.(2023)
摘要
Synthetic aperture radar (SAR) images are impaired by the presence of speckles. Despite the deep interest of scholars in the last decades, SAR image despeckling is still an open issue. Among different approaches, recently, many deep-learning (DL) methods have been proposed following both supervised and unsupervised training approaches. There are two main challenges within the supervised framework: training data and cost functions. Our approach builds training datasets that are varied and realistic using a multicategory generalized Gaussian coherent SAR simulator. It allows modeling a variety of SAR scenarios beyond the fully developed speckle hypothesis, which is only valid in homogeneous areas. Such multicategory simulated speckle is then applied to a noise-free reference obtained by multilooking a temporal stack of actual SAR images to obtain the noisy input. We design an effective multiobjective cost function that accounts for texture, edge, and statistical properties preservation. We show the superiority of our approach assessing numerically and quantitatively its performance with three different SAR datasets.
更多查看译文
关键词
Convolutional neural network (CNN),deep learning (DL),despeckling,statistical distribution,synthetic aperture radar (SAR)
AI 理解论文
溯源树
样例
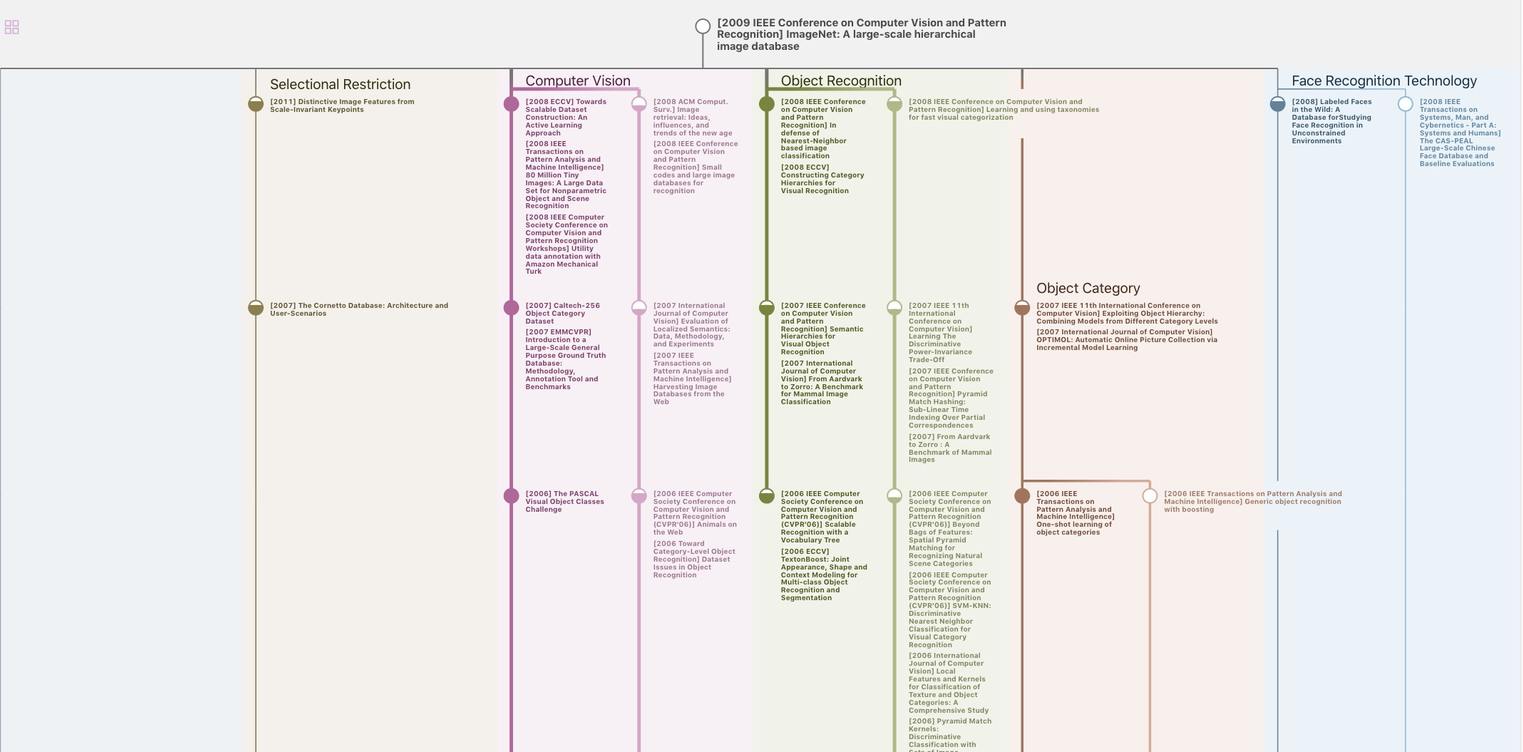
生成溯源树,研究论文发展脉络
Chat Paper
正在生成论文摘要