Distributed Clustering for Secure Multitask Estimation Based on Adaptive Task Switching
IEEE TRANSACTIONS ON AEROSPACE AND ELECTRONIC SYSTEMS(2023)
摘要
Consider adversarial multitask networks with a high cost of sharing genuine information, where agents feed others unreliable information according to a time-varying probability caused by a high privacy cost. This makes agents in the network tend to be selfish, and it greatly increases the difficulty of effective information sharing and distributed multitask estimation without the prior cluster information. To address these problems, we propose an adaptive task-switching strategy that is governed by our formulated information sharing model, which cooperates with the proposed distributed adaptive task-switching learning and clustering for secure estimation over adversarial multi-task network (DATSLCS-AM) algorithm. This distributed algorithm guides agents to cooperate reasonably following reputation updating and pseudoclustering, which can improve the effectiveness of information sharing and also increase the security of estimation over adversarial multitask networks. The theoretical analysis of the adaptive pseudoclustering threshold is provided. Finally, extensive simulations validate the superior performance of our distributed algorithm.
更多查看译文
关键词
Task analysis,Multitasking,Adaptation models,Estimation,Information sharing,Data models,Adaptive systems,Adaptive task switching,adversarial multitask networks,distributed secure estimation,information sharing,pseudoclustering
AI 理解论文
溯源树
样例
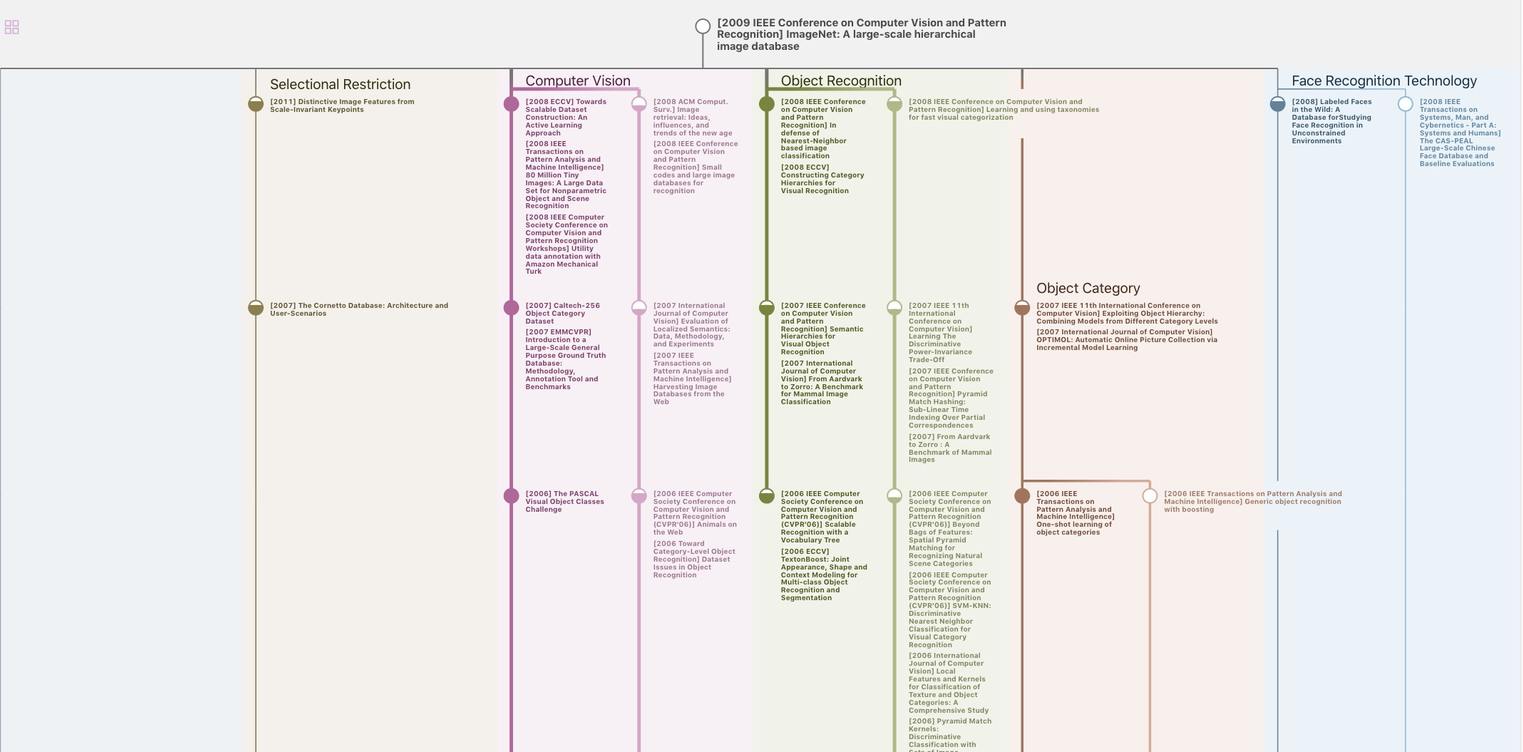
生成溯源树,研究论文发展脉络
Chat Paper
正在生成论文摘要