MF-GCN: Motion Flow-Based Graph Network Learning Dynamics for Aerial IR Target Recognition
IEEE TRANSACTIONS ON AEROSPACE AND ELECTRONIC SYSTEMS(2023)
摘要
The primary technology of the IR imaging seeker optoelectronic countermeasure system includes the automatic recognition and tracking of air targets. New complex IR interference factors pose severe challenges to the ability of IR thermal imaging seekers to identify and lock targets accurately. Despite significant recent advances, machine vision systems still lag substantially behind biological vision systems in terms of performance and robustness. The distinguishing feature of the brain is its ability to detect learned objects under various nonideal situations. Inspired by the sensitivity of the dorsal visual pathway to object motion information, a graph convolutional network learning model is developed for aerial target recognition, called the adaptive graph reasoning method driven by a motion flow model, which employs the predicted object optical flow information to promote the keypoint estimation performance. First, an adaptive graph fusion module is designed to establish an adaptation mechanism to generalize keypoint features from the regular network to the target skeleton graph model. Then, the optical flow estimation features are utilized to understand the graph reasoning of the target keypoint motion. Finally, we propose an aerial target pose estimation framework which effectively integrates the temporal cues across frames by combining the motion features of optical flow estimation, and effectively promotes the estimation accuracy of keypoints. Finally, our extensive experiments in the established aerial target flight IR dataset show the efficiency of the presented model.
更多查看译文
关键词
Visualization,Target tracking,Target recognition,Predictive models,Interference,Brain modeling,Optical imaging,Aerial infrared (IR) target,antiocclusion,graph network,motion flow,target recognition
AI 理解论文
溯源树
样例
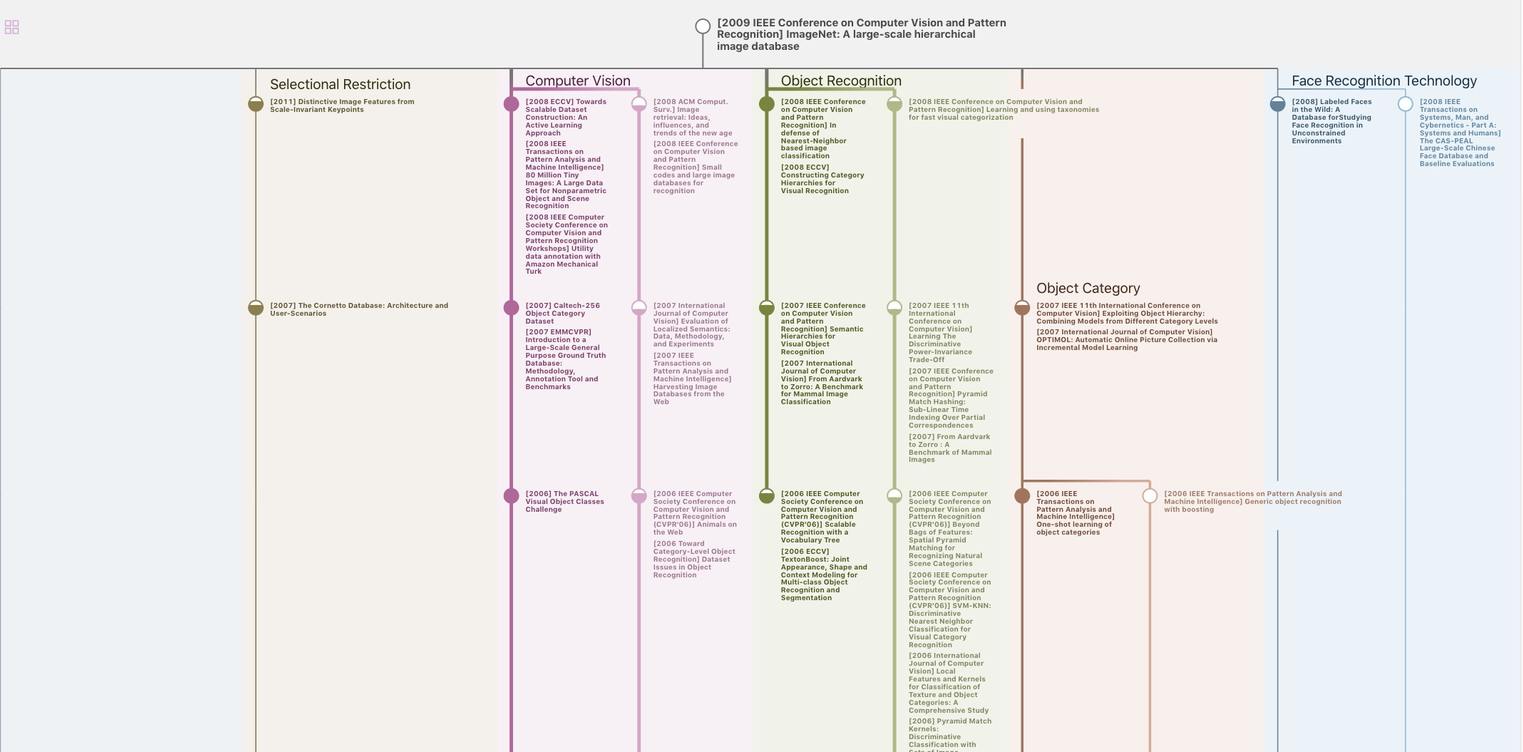
生成溯源树,研究论文发展脉络
Chat Paper
正在生成论文摘要