Milstein-driven neural stochastic differential equation model with uncertainty estimates
Pattern Recognit. Lett.(2023)
摘要
Incorporating uncertainty quantification into the modeling of deep learning-based model has become a research focus in the deep learning community. Within this group of methods, stochastic differential equation (SDE)-based models have demonstrated advantages in their ability to model uncertainty quantification. However, the use of Euler’s method in these models introduces imprecise numerical solutions, which limits the accuracy of SDE systems and weakens the performance of the network. In this study, we build a more precise Milstein-driven SDE network (MDSDE-Net) to improve the network performance. In addition, we analyze the convergence of the Milstein scheme and theoretically guarantee the feasibility of MDSDE-Net. Experimental and theoretical results show that the MDSDE-Net outperforms existing models.
更多查看译文
关键词
Uncertainty quantification,Stochastic differential equation,Milstein-driven SDE-net
AI 理解论文
溯源树
样例
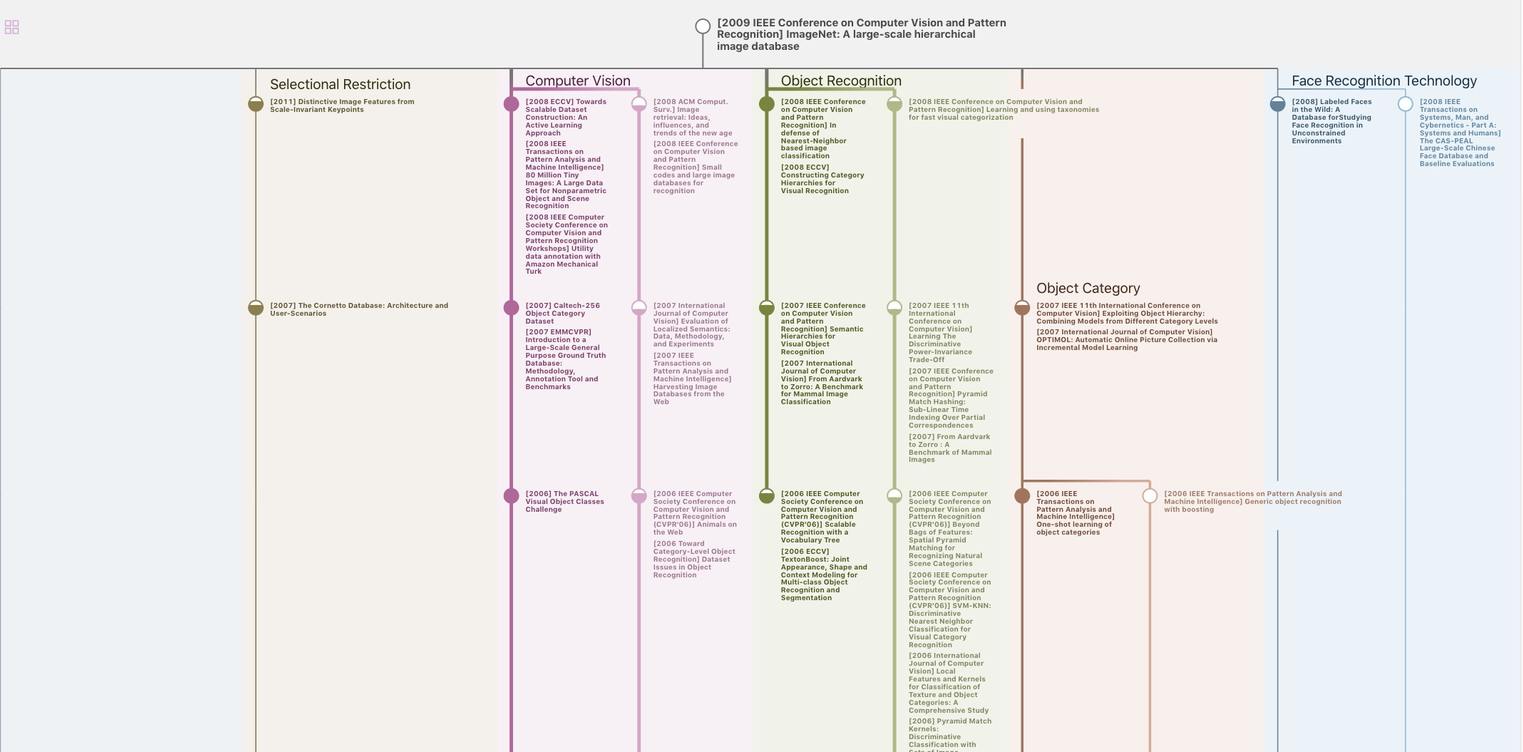
生成溯源树,研究论文发展脉络
Chat Paper
正在生成论文摘要